Key Takeaways
1. Model selection requires balancing performance, complexity, and practical constraints
"Simplicity serves three purposes. First, simpler models are easier to deploy, and deploying your model early allows you to validate that your prediction pipeline is consistent with your training pile. Second, starting with something simple and adding more complex components step-by-step makes it easier to understand your model and debug it. Third, the simplest model serves as a baseline to which you can compare your more complex models."
Performance vs. complexity tradeoff. When selecting a model, consider:
- Model performance (accuracy, F1 score, etc.)
- Computational requirements (training time, inference speed)
- Interpretability
- Amount of training data needed
- Ease of deployment and maintenance
Start with simple baseline models like logistic regression or decision trees before moving to more complex neural networks. This allows you to:
- Establish a performance benchmark
- Validate your data pipeline and problem framing
- Gain interpretability into how features impact predictions
Avoid state-of-the-art trap. The latest models may not be optimal for your specific use case. Consider:
- Your data characteristics and volume
- Computational constraints
- Need for explainability
- Deployment environment
2. Ensemble methods can significantly boost model performance
"20 out of 22 winning solutions on Kaggle competitions in 2021, as of August 2021, use ensembles."
Ensemble types:
- Bagging: Train models on bootstrapped datasets (e.g. Random Forests)
- Boosting: Train models sequentially, focusing on misclassified examples (e.g. XGBoost)
- Stacking: Use predictions from base models as inputs to a meta-learner
Ensembles work by combining diverse models to reduce bias and variance. Key considerations:
- Use uncorrelated base models for maximum benefit
- Balance performance gain vs increased complexity
- Popular in competitions but can be challenging to deploy in production
Ensemble methods like XGBoost and Random Forests often provide strong performance out-of-the-box for many problems, especially with structured data.
3. AutoML and hyperparameter tuning automate model optimization
"Instead of paying a group of 100 ML researchers/engineers to fiddle with various models and eventually select a sub-optimal one, why not use that money on compute to search for the optimal model?"
Levels of AutoML:
- Hyperparameter tuning: Optimize model hyperparameters
- Neural architecture search: Discover optimal neural network architectures
- Learned optimizers: Use ML to learn optimization algorithms
Hyperparameter tuning is the most accessible and widely used form of AutoML. Common approaches:
- Random search
- Grid search
- Bayesian optimization
Benefits of AutoML:
- Reduces manual effort in model development
- Can discover non-intuitive model configurations
- Enables non-experts to apply ML effectively
Challenges include high computational costs and potential overfitting to the validation set. Use AutoML judiciously and always evaluate final performance on a held-out test set.
4. Distributed training enables scaling to large datasets and models
"As models are getting bigger and more resource-intensive, companies care a lot more about training at scale."
Distributed training challenges:
- Data that doesn't fit in memory
- Coordinating updates across multiple machines
- Balancing computation and communication overhead
Techniques for large-scale training:
- Gradient checkpointing: Trade computation for memory savings
- Large batch training: Efficiently utilize multiple GPUs/TPUs
- Mixed precision: Use lower precision arithmetic to speed up training
Distributed training requires careful consideration of:
- Hardware setup (GPUs, high-speed interconnects)
- Software frameworks (PyTorch Distributed, Horovod)
- Optimization algorithms (synchronous vs asynchronous SGD)
As models grow (e.g. large language models like GPT-3), distributed training becomes essential for pushing the boundaries of ML capabilities.
5. Experiment tracking and versioning are crucial for reproducibility
"Imagine this scenario. You and your team spent the last few weeks tweaking your model and one of the runs finally showed promising results. You wanted to use it for more extensive tests so you tried to replicate it using the set of hyperparameters you'd noted down somewhere, only to find out that the results weren't quite the same."
Key elements to track:
- Code versions
- Data versions and preprocessing steps
- Model architecture and hyperparameters
- Training environment (hardware, software versions)
- Evaluation metrics and artifacts (loss curves, model checkpoints)
Tools for experiment tracking:
- MLflow
- Weights & Biases
- DVC (Data Version Control)
Benefits of rigorous tracking:
- Reproducibility of results
- Easier collaboration within teams
- Ability to compare experiments and identify impactful changes
Challenges include versioning large datasets and handling non-determinism in training. Establish clear protocols for experiment documentation within your team.
6. Data parallelism and model parallelism offer different approaches to distributed training
"The most common parallelization method is data parallelism: you split your data on multiple machines, train your model on all of them, and accumulate gradients."
Data parallelism:
- Each machine has a full copy of the model
- Data is split across machines
- Gradients are aggregated to update the global model
Pros:
- Easier to implement
- Scales well with dataset size
Cons:
- Limited by model size fitting on a single device
- Communication overhead for gradient synchronization
Model parallelism:
- Model is split across multiple devices
- Each device processes a portion of the model
Pros:
- Can handle very large models
- Reduces memory requirements per device
Cons:
- More complex to implement
- Can lead to device underutilization
Hybrid approaches like pipeline parallelism combine elements of both to optimize resource usage. The best approach depends on your specific model architecture and hardware constraints.
7. Effective ML systems often start simple and increase in complexity over time
"There are four phases of adopting ML. The solutions from a phase can be used as baselines to evaluate the solutions from the next phase."
Four phases of ML adoption:
- Before ML: Use heuristics and rule-based systems
- Simplest ML models: Implement basic algorithms (e.g. logistic regression)
- Optimizing simple models: Feature engineering, ensembles, hyperparameter tuning
- Complex systems: Deep learning, AutoML, custom architectures
Benefits of this incremental approach:
- Establishes baselines for comparison
- Builds necessary infrastructure gradually
- Identifies low-hanging fruit for improvement
Start by clearly defining your problem and success metrics. A simple model that solves 80% of your problem may be sufficient initially. As you gather more data and insights, incrementally increase model complexity to address remaining challenges.
Remember that ML is not always the answer – sometimes simple heuristics or traditional software engineering approaches may be more appropriate and maintainable.
Last updated:
FAQ
What's "Designing Machine Learning Systems" about?
- Comprehensive Guide: "Designing Machine Learning Systems" by Chip Huyen is a comprehensive guide to building production-ready machine learning applications. It covers the entire lifecycle of machine learning systems, from data engineering to deployment and monitoring.
- Iterative Process: The book emphasizes an iterative process for developing machine learning systems, highlighting the importance of continuous improvement and adaptation to changing environments and requirements.
- Real-World Challenges: It addresses real-world challenges in deploying machine learning systems, such as data management, scalability, and ethical considerations, providing practical solutions and insights.
- Focus on Production: Unlike many other resources, this book focuses on the practical aspects of deploying machine learning systems in production, making it a valuable resource for practitioners in the field.
Why should I read "Designing Machine Learning Systems"?
- Practical Insights: The book offers practical insights into the challenges and solutions of deploying machine learning systems in production, which are often overlooked in academic settings.
- Comprehensive Coverage: It covers a wide range of topics, from data engineering and feature engineering to model development and deployment, providing a holistic view of the machine learning lifecycle.
- Real-World Examples: The author uses real-world examples and case studies to illustrate key concepts, making the content relatable and applicable to real-world scenarios.
- Expert Author: Chip Huyen is an experienced engineer and educator, bringing her expertise in machine learning systems design to the book, making it a credible and authoritative resource.
What are the key takeaways of "Designing Machine Learning Systems"?
- Iterative Development: Machine learning systems should be developed iteratively, with continuous monitoring and updates to adapt to changing data and requirements.
- Data is Crucial: The success of machine learning systems heavily depends on the quality and quantity of data, emphasizing the importance of data engineering and management.
- System Design: Designing machine learning systems involves considering various components, including algorithms, data, infrastructure, and hardware, to meet specified requirements.
- Ethical Considerations: The book highlights the importance of addressing ethical and societal challenges, such as fairness and interpretability, in deploying machine learning systems.
What are the best quotes from "Designing Machine Learning Systems" and what do they mean?
- "Data is profoundly dumb." This quote emphasizes the importance of intelligent design and the limitations of relying solely on data for machine learning systems.
- "Machine learning is not a magic tool that can solve all problems." It highlights the need for careful consideration of when and how to use machine learning, rather than viewing it as a one-size-fits-all solution.
- "Complex ML systems are made up of simpler building blocks." This underscores the importance of understanding and mastering the fundamental components of machine learning systems to build more complex applications.
- "The vast majority of ML-related jobs will be, and already are, in productionizing ML." This quote reflects the growing demand for skills in deploying and maintaining machine learning systems in production environments.
How does "Designing Machine Learning Systems" approach data engineering?
- Data Importance: The book emphasizes the critical role of data in building successful machine learning systems, highlighting the need for effective data management and processing.
- Data Sources: It discusses various data sources, including user input, system-generated data, and third-party data, and their implications for machine learning systems.
- Data Formats: The book covers different data serialization formats, such as JSON, CSV, and Parquet, and their suitability for different use cases.
- ETL Process: It explains the Extract, Transform, Load (ETL) process, which is essential for preparing data for machine learning applications.
What is the iterative process for designing machine learning systems in "Designing Machine Learning Systems"?
- Project Scoping: The process begins with scoping the project, defining goals, objectives, constraints, and evaluation criteria.
- Data Engineering: It involves processing and manipulating data to create training datasets, which is crucial for model development.
- Model Development: This step includes generating features, training models, optimizing them, and evaluating their performance.
- Deployment and Monitoring: After deployment, models need to be monitored for performance decay and updated to adapt to changing environments and requirements.
How does "Designing Machine Learning Systems" address model development and evaluation?
- Model Selection: The book discusses how to select the best model for a problem, considering factors like performance, interpretability, and computational requirements.
- Training Techniques: It covers training techniques, including distributed training and experiment tracking, to handle large-scale models and datasets.
- Evaluation Methods: The book emphasizes the importance of robust evaluation methods, such as perturbation tests and slice-based evaluation, to ensure model reliability and fairness.
- AutoML: It explores the use of automated machine learning (AutoML) for hyperparameter tuning and architecture search to optimize model performance.
What are the challenges of deploying machine learning systems in production according to "Designing Machine Learning Systems"?
- Scalability: Machine learning systems must be scalable to handle large volumes of data and traffic, requiring efficient resource management and infrastructure.
- Reliability: The systems should be reliable, performing correctly even in the face of hardware or software faults and human errors.
- Ethical Challenges: Deploying machine learning systems involves addressing ethical challenges, such as fairness, interpretability, and bias, to ensure responsible AI use.
- Data Management: Effective data management is crucial, as data in production is often messy, constantly shifting, and subject to privacy and regulatory concerns.
How does "Designing Machine Learning Systems" handle feature engineering?
- Feature Importance: The book discusses the importance of feature engineering in improving model performance and provides techniques for handling missing values, scaling, and encoding categorical features.
- Data Leakage: It highlights the risk of data leakage, where information from the future or test data leaks into the training process, and provides strategies to detect and prevent it.
- Feature Generalization: The book emphasizes the need for features that generalize well to unseen data, considering factors like feature coverage and distribution.
- Cross Features: It covers the creation of cross features to model non-linear relationships between variables, which can enhance model performance.
What is the role of AutoML in "Designing Machine Learning Systems"?
- Hyperparameter Tuning: AutoML is used for hyperparameter tuning, automating the process of finding the optimal set of hyperparameters for a given model.
- Architecture Search: The book explores neural architecture search (NAS), which automates the design of model architectures by searching for the best configuration of building blocks.
- Learned Optimizers: It discusses the concept of learned optimizers, which replace traditional learning algorithms with neural networks to improve model training.
- Efficiency and Performance: AutoML techniques, such as EfficientNets, demonstrate significant improvements in model accuracy and efficiency, making them valuable for production systems.
How does "Designing Machine Learning Systems" address ethical considerations in machine learning?
- Fairness: The book emphasizes the importance of fairness in machine learning systems, highlighting the need to address biases and ensure equitable treatment of all users.
- Interpretability: It discusses the need for interpretability in machine learning models, enabling users to understand and trust the decisions made by the system.
- Bias Detection: The book provides methods for detecting and mitigating biases in machine learning models, such as invariance tests and slice-based evaluation.
- Societal Impact: It highlights the potential societal impact of machine learning systems, urging practitioners to consider the ethical implications of their work.
What are the best practices for deploying machine learning systems in production according to "Designing Machine Learning Systems"?
- Iterative Development: The book advocates for an iterative development process, with continuous monitoring and updates to adapt to changing data and requirements.
- Scalable Infrastructure: It emphasizes the need for scalable infrastructure to handle large volumes of data and traffic, ensuring efficient resource management.
- Robust Evaluation: The book highlights the importance of robust evaluation methods, such as perturbation tests and model calibration, to ensure model reliability and fairness.
- Ethical Considerations: It stresses the importance of addressing ethical challenges, such as fairness and interpretability, to ensure responsible AI use in production systems.
Review Summary
Designing Machine Learning Systems receives high praise for its comprehensive coverage of MLOps and practical ML system implementation. Readers appreciate its focus on concepts rather than specific tools, making it valuable for both beginners and experienced practitioners. The book covers data engineering, feature engineering, model deployment, and monitoring, offering insights into real-world challenges. Many reviewers consider it essential reading for data scientists and ML engineers, noting its relevance to current industry practices and its emphasis on end-to-end ML system design.
Similar Books
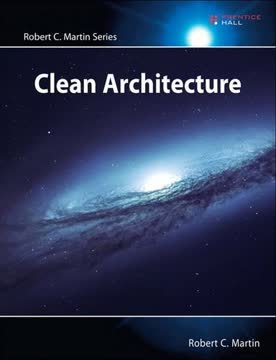
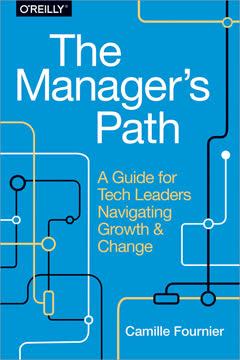
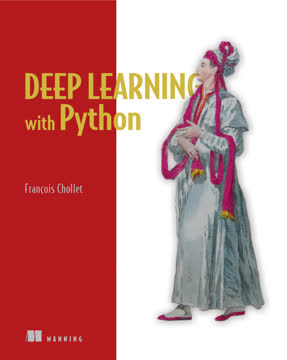
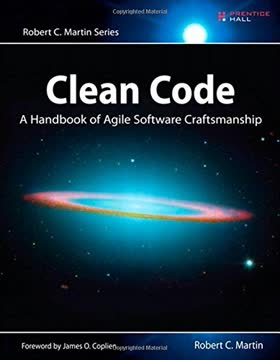
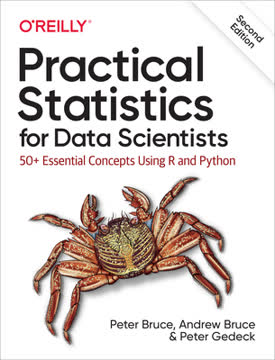
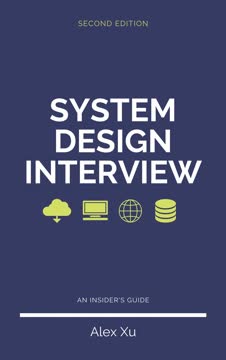
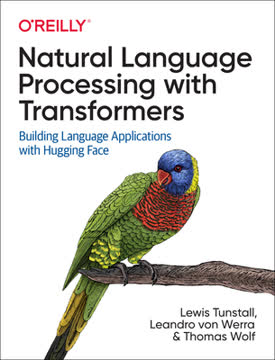
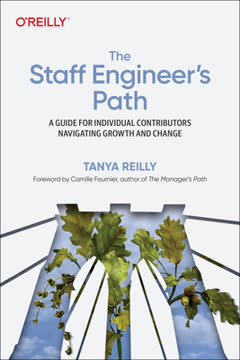
Download PDF
Download EPUB
.epub
digital book format is ideal for reading ebooks on phones, tablets, and e-readers.