Key Takeaways
1. Chess's Enduring Role in AI History
The ancient board game of chess has played a significant role in the history of artificial intelligence, if mostly as a chimera.
Early AI ambitions. From Alan Turing and Claude Shannon, pioneers of computation saw chess as a testbed for artificial intelligence. Early ideas focused on knowledge-based, human-like approaches, but limitations in computing power pushed development towards brute-force search algorithms. This era culminated in IBM's Deep Blue defeating Garry Kasparov in 1997, a landmark moment achieved through sheer calculation speed rather than human-like intelligence.
Brute force dominance. The success of brute-force methods, exemplified by engines like Stockfish, led many to believe chess had little more to offer AI research. These programs relied on massive opening books, endgame databases, and rapid calculation, seemingly exhausting the game's potential as a cognition laboratory. However, this focus on calculation overshadowed deeper questions about learning and intuition that early AI researchers had posed.
A new chapter. The advent of DeepMind's AlphaGo, which mastered Go (a game resistant to brute force), signaled a shift. Its successor, AlphaGo Zero, learned purely through self-play, eschewing human knowledge. This breakthrough paved the way for AlphaZero, demonstrating that a self-taught algorithm could not only compete with but surpass the strongest traditional chess engines, reigniting chess's relevance in the AI spotlight.
2. AlphaZero's Revolutionary Self-Learning Approach
Unlike with Go, of course IBM’s groundbreaking Deep Blue program had long proven chess could be mastered by computers. Subsequently its legion of successors, including Stockfish, Komodo and Houdini, have become extraordinarily strong. But all these programs rely on thousands of hardcoded rules and heuristics painstakingly handcrafted by human experts over years of work. By contrast, AlphaZero is nothing like these programs. It is entirely self-taught and learns to play chess completely from first principles.
Learning from scratch. AlphaZero starts with only the basic rules of chess and learns to play by playing millions of games against itself. This process, called reinforcement learning, allows it to discover strategies and evaluations independently, without any human chess knowledge or databases. This contrasts sharply with traditional engines built on decades of human expertise and handcrafted algorithms.
Neural network core. At the heart of AlphaZero is a neural network that serves two functions:
- Policy network: Predicts the probability of each possible move being the best.
- Value network: Estimates the expected outcome (win, loss, or draw) from a given position.
These networks guide a Monte Carlo Tree Search (MCTS), focusing computational resources on the most promising lines, unlike the exhaustive alpha-beta search used by traditional engines.
Rapid mastery. Within just nine hours of self-play, AlphaZero reached superhuman strength, playing 44 million games against itself. This rapid ascent demonstrates the power of its general learning algorithm, capable of mastering complex domains quickly without domain-specific tuning. This generality is a key goal for DeepMind, aiming to apply similar systems to real-world problems.
3. Beyond Brute Force: AlphaZero's Intuitive Evaluation
AlphaZero isn’t just applying human knowledge and plowing through billions of positions to generate moves – it’s creating its own knowledge first.
Probabilistic assessment. Unlike traditional engines that evaluate positions based on a single "best" line and express advantage in pawn units, AlphaZero uses a probabilistic approach. It estimates its expected score (win, draw, or loss percentage) based on an average evaluation across many likely continuations. This gives its evaluation a more "intuitive" feel, akin to a human grandmaster's overall sense of a position's promise.
Flexible evaluation function. AlphaZero's neural network allows for a highly flexible evaluation function, capable of understanding how different positional features interact in complex ways. This goes beyond the linear combination of predefined features used by traditional engines (like material, mobility, king safety scores), potentially enabling a deeper, more nuanced understanding of dynamic positions.
Challenging "0.00". AlphaZero's evaluations often differ significantly from traditional engines, particularly in complex positions where engines might default to a "0.00" (equal) assessment. AlphaZero's willingness to see a clear advantage in positions rated equal by others, especially those with dynamic imbalances or attacking potential, suggests it values factors like initiative and piece activity differently, often finding ways to convert these advantages.
4. AlphaZero's Distinctive Attacking Style
And while chess style is hardly of great interest to the AI crowd, I was quite happy to see AlphaZero’s dynamic, sacrificial style.
Aggressive and dynamic. AlphaZero exhibits a clear preference for dynamic, attacking play, often targeting the opponent's king directly from the opening. This contrasts with the often cautious, defensive style favored by traditional engines in complex positions. AlphaZero's games are characterized by:
- Early pawn sacrifices to open lines.
- Focus on piece activity over material balance.
- Relentless pressure on the opponent's king.
Schematic approach. AlphaZero often follows a clear, repeatable schematic approach to attack. This involves:
- Fixing the center to prevent counterplay.
- Opening lines (files and diagonals) towards the opponent's king, often through sacrifices.
- Bringing pieces (especially knights and rooks) to advanced outposts near the king.
- Combining pressure from multiple angles (e.g., open file + open diagonal).
Long-term vision. AlphaZero's attacks are not always immediate mating sequences. They are often "slow-burning," building pressure over many moves by improving piece coordination and restricting the opponent's options. This requires a long-term positional understanding that goes beyond tactical calculation.
5. Mastering Piece Mobility and Outposts
AlphaZero demonstrates an uncanny ability to discover strong, safe outposts for its pieces and to formulate a plan for establishing them there.
Activity is paramount. A core principle of AlphaZero's play is maximizing the activity and mobility of its own pieces while restricting the opponent's. This often outweighs material considerations, as superior activity can create overwhelming attacking chances or lead to positional advantages.
Strategic outposts. AlphaZero excels at identifying and occupying key outposts for its pieces, particularly knights. It is willing to invest time and even material to maneuver pieces to squares where they cannot be easily dislodged by enemy pawns, allowing them to exert long-term pressure. Examples include:
- Knights on central or kingside squares near the opponent's king.
- Bishops on long, open diagonals.
- Even rooks on advanced ranks (5th or 6th) or open files.
Restricting the opponent. AlphaZero actively seeks to limit the mobility of the opponent's pieces, especially the king. By restricting the king's movement, AlphaZero reduces its defensive capabilities and makes it a more vulnerable target for attack, a factor it values highly in both middlegames and endgames.
6. The Power of the Rook's Pawn Advance
AlphaZero frequently advances its rook’s pawn as part of its attack and plants it close to the opponent’s king.
Aggressive flank play. A signature move of AlphaZero is the early advance of a rook's pawn (typically the h-pawn) on the side where the opponent has castled. This is done to:
- Weaken the pawn structure around the enemy king.
- Create targets for subsequent attacks.
- Restrict the king's movement.
Creating weaknesses. Pushing the h-pawn to h6 (for White) or h3 (for Black) forces the opponent to react, often by advancing their own g-pawn. This creates dark-square weaknesses and limits the king's escape squares, setting the stage for attacks along newly opened lines or diagonals.
Beyond opening files. While the h-pawn can be used to open the h-file, AlphaZero often prefers to push it further to h6, using it as an advanced attacking unit and a long-term threat in the endgame. This approach is seen even in quiet openings and opposite-side castling scenarios, demonstrating AlphaZero's consistent application of this aggressive theme.
7. Exploiting Color Complexes and Opposite Bishops
Matthew explains AlphaZero’s fondness for positions with opposite-coloured bishops.
Unopposed attack channels. AlphaZero demonstrates a strong understanding of color complexes, particularly in positions with opposite-colored bishops. In such scenarios, its bishop can move freely along squares of its color without being challenged by an opponent's bishop, creating an unopposed channel for attack.
Targeting weak squares. AlphaZero actively seeks to create and exploit weak complexes of same-colored squares around the opponent's king. This is often achieved by:
- Exchanging the opponent's bishop of that color.
- Forcing pawn moves that leave holes on squares of that color.
- Combining pressure from pieces (bishops, queens, knights) on those squares.
Sacrifice for control. AlphaZero is willing to sacrifice material (pawns or even exchanges) to gain control over a critical color complex near the enemy king. This allows its pieces to infiltrate and exert pressure that the opponent cannot easily block, leading to decisive advantages even with a material deficit.
8. Strategic Sacrifices for Dynamic Advantage
AlphaZero makes many brilliant sacrifices for long-term positional advantage.
Beyond tactical gain. AlphaZero's sacrifices are not always aimed at immediate checkmate or regaining material through forced variations. They are often strategic investments made to achieve dynamic advantages, such as:
- Sacrifices for time: Giving up material to gain tempi for an attack on the opposite flank.
- Sacrifices for space: Opening lines (files or diagonals) towards the enemy king.
- Sacrifices for damage: Destroying the opponent's king's pawn cover.
Cumulative effect. These sacrifices often work in combination, building a cumulative advantage in piece activity, open lines, and king vulnerability. AlphaZero's willingness to sacrifice multiple pawns or even pieces for these positional and dynamic factors is a hallmark of its aggressive style.
Confidence in compensation. AlphaZero's probabilistic evaluation allows it to assess the long-term potential of positions arising from sacrifices. It is confident in its ability to convert dynamic advantages, even if the immediate material balance is unfavorable, leading to bold and creative attacking sequences.
9. AlphaZero's Active, Complicating Defense
AlphaZero defends by creating confusion and introducing tactics into the game.
Avoiding passive defense. While Stockfish excels at absorbing pressure and finding precise, often "ugly," defensive moves, AlphaZero's primary defensive strategy is to avoid being in a passive position in the first place. Its opening choices and middlegame play are geared towards maintaining activity and initiative.
Complicate when worse. When forced into a difficult or passive position (often in TCEC openings it didn't choose), AlphaZero tends to seek complications. It is willing to sacrifice material to introduce tactical possibilities and disrupt the opponent's plans, aiming to turn a clearly worse position into a messy, unclear one where the opponent might err.
Contrast with Stockfish. This contrasts with Stockfish's preference for precise, calculated defenses that aim to hold the balance even in seemingly lost positions. AlphaZero's active defense is more intuitive and human-like, prioritizing dynamic chances over static material preservation when under pressure.
10. AlphaZero's Classical Yet Sharp Opening Repertoire
AlphaZero’s opening play with both colours is strictly classical, favouring central control and simple development.
Self-taught repertoire. Despite learning from scratch, AlphaZero developed a classical opening repertoire focused on central control and rapid development. As White, it primarily plays 1.d4 and 1.♘f3, often transposing into solid Queen's Pawn structures like the Queen's Indian or Semi-Slav. As Black, it consistently meets 1.e4 with 1...e5 (often the Berlin Defense) and 1.d4 with 1...♘f6 followed by 2...e6 (aiming for Nimzo/Ragozin).
Strategic choices. AlphaZero's opening choices are not random; they steer the game towards positions where its strengths can be leveraged:
- Fixed or stable centers that allow for wing attacks.
- Opportunities for piece activity and mobility.
- Potential for creating weaknesses around the opponent's king.
Sharpness within solidity. While the initial moves are classical, AlphaZero injects sharpness through aggressive follow-ups, including early pawn sacrifices (e.g., in the Queen's Indian or Semi-Slav) and immediate flank attacks (e.g., the h-pawn push). This combines the positional soundness of classical openings with the dynamic aggression characteristic of AlphaZero's style.
11. AlphaZero as a Glimpse into General AI's Promise
Ultimately the whole point of building general learning systems like AlphaZero is so they can be applied in all sorts of ways to creating solutions for real world problems that will be of huge benefit to everyone in society.
Beyond the game. DeepMind's ambition is to build general intelligent systems that can learn to solve any complex task. Games like chess serve as ideal testbeds due to their complexity, clear objectives, and efficient simulation capabilities. AlphaZero's ability to master multiple games from scratch demonstrates a significant step towards this goal of generality.
Accelerating science. The techniques developed for AlphaZero, particularly reinforcement learning and neural networks, hold immense potential for applications beyond games. DeepMind envisions using these systems to accelerate scientific discovery in critical areas like:
- Climate science
- Material science
- Drug discovery
- Medical diagnostics
Human-machine collaboration. AlphaZero's unique insights into chess, developed independently of human knowledge, suggest that AI can discover novel and superior strategies. This points towards a future where humans collaborate with AI systems as "shepherds," overseeing AI experts to solve problems, rather than simply using AI as tools.
Last updated:
Review Summary
Game Changer is highly praised for its in-depth analysis of AlphaZero's revolutionary chess-playing style. Readers appreciate the book's exploration of AI's potential and its impact on chess strategy. Many find the game analyses fascinating, though some note it requires advanced chess knowledge to fully appreciate. The book is commended for its clear explanations of AlphaZero's approach and historical comparisons. While some readers wanted more focus on AI technology, most chess enthusiasts and AI enthusiasts find it an enlightening and thought-provoking read.
Similar Books
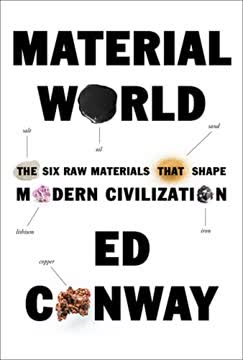
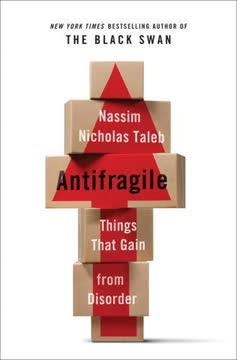
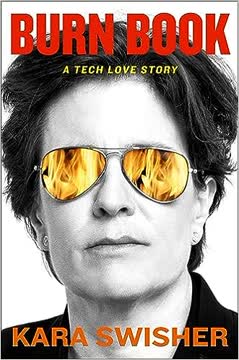
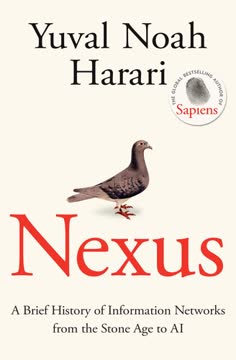
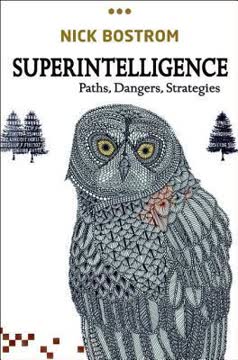
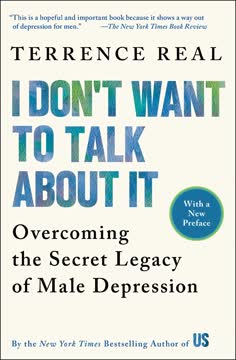
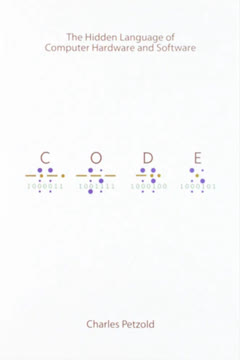
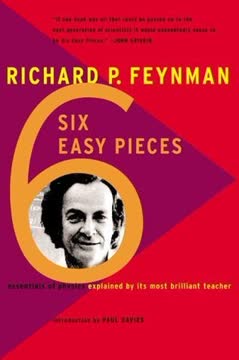
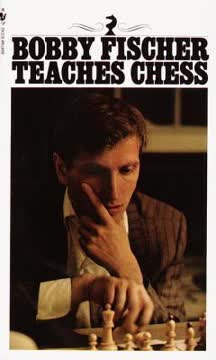
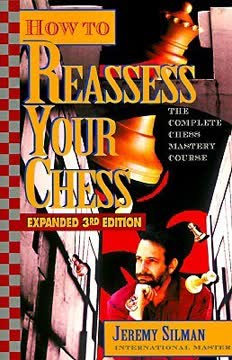
Download PDF
Download EPUB
.epub
digital book format is ideal for reading ebooks on phones, tablets, and e-readers.