Key Takeaways
1. Graph databases excel at managing highly connected data
Graphs are truly remarkable structures. Our understanding of them is rooted in hundreds of years of mathematical and scientific study. And yet we're only just beginning to understand how to apply them to our personal, social, and business lives.
Connected data challenges: Traditional relational databases struggle with highly interconnected data, facing performance issues as the number of joins increases. Graph databases address this by using a network model that naturally represents and efficiently queries relationships.
Use cases: Graph databases shine in domains with complex relationships:
- Social networks
- Recommendation engines
- Fraud detection
- Network and IT operations
- Master data management
Performance advantages: For queries involving multiple connections, graph databases can be orders of magnitude faster than relational databases, especially as data size and complexity grow.
2. Property graphs offer a flexible and intuitive data model
The labeled property graph model (in Appendix A, we discuss alternative graph data models in more detail). A labeled property graph has the following characteristics:
Key components:
- Nodes: Represent entities
- Relationships: Connect nodes, are named and directional
- Properties: Key-value pairs on both nodes and relationships
- Labels: Categorize nodes (multiple labels per node allowed)
Advantages:
- Intuitive: Closely mirrors how we naturally think about and sketch data
- Flexible: Easily accommodates changing requirements and data structures
- Expressive: Captures complex scenarios with clarity
Whiteboard friendly: The property graph model often allows developers to directly implement data structures sketched during analysis and design phases.
3. Cypher: A powerful declarative query language for graphs
Cypher is an expressive (yet compact) graph database query language. Although currently specific to Neo4j, its close affinity with our habit of representing graphs as diagrams makes it ideal for programmatically describing graphs.
ASCII art patterns: Cypher uses intuitive ASCII art to describe graph patterns, making queries easy to read and write.
Key components:
- MATCH: Specify patterns to find in the graph
- WHERE: Filter results
- RETURN: Specify what data to retrieve
- CREATE/MERGE: Add new data to the graph
Declarative nature: Developers describe what they want to find, not how to find it, allowing the database to optimize query execution.
4. Graph modeling focuses on relationships between entities
Graph modeling naturally fits with the way we tend to abstract details from a domain using circles and boxes, and then describe the connections between these things by joining them with arrows and lines.
Domain-driven modeling: Graph data models closely reflect the structure of the problem domain, reducing the semantic gap between conceptual models and database implementation.
Iterative development: Graph models are easily evolved as requirements change:
- Add new node types and relationships
- Extend existing structures without disrupting current queries
- Refactor by introducing or removing intermediate nodes
Best practices:
- Model for query patterns, not just data storage
- Use meaningful relationship names
- Consider bidirectional relationships when appropriate
- Use node labels to categorize entities
5. Native graph processing enables high-performance traversals
A database engine that utilizes index-free adjacency is one in which each node maintains direct references to its adjacent nodes. Each node, therefore, acts as a micro-index of other nearby nodes, which is much cheaper than using global indexes.
Index-free adjacency: Nodes directly reference their neighbors, eliminating the need for index lookups during traversals.
Performance implications:
- Traversal time is proportional to the number of nodes visited, not the total size of the graph
- Enables extremely fast navigation of relationships
- Particularly efficient for localized queries (e.g., finding friends-of-friends)
Contrast with relational model: Joins in relational databases become increasingly expensive as data size grows, while graph traversals maintain consistent performance.
6. Graph databases support ACID transactions and scalability
Transactions in Neo4j are semantically identical to traditional database transactions. Writes occur within a transaction context, with write locks being taken for consistency purposes on any nodes and relationships involved in the transaction.
ACID compliance: Many graph databases, including Neo4j, provide full ACID (Atomicity, Consistency, Isolation, Durability) transaction support.
Scalability options:
- Read scaling: Distribute read queries across multiple replicas
- Write scaling: Some graph databases offer sharding for write scalability
- Cache sharding: Optimize for graph-local queries across cluster
High availability: Clustering and replication strategies ensure continuous operation and data consistency.
7. Graph theory algorithms unlock predictive insights
Graph theory techniques are broadly applicable to a wide range of problems. They are especially useful when we first want to gain some insight into a new domain — or even understand what kind of insight it's possible to extract from a domain.
Key algorithms:
- Shortest path (e.g., Dijkstra's algorithm)
- Centrality measures
- Community detection
- Similarity calculations
Predictive power: Graph algorithms can reveal hidden patterns and connections:
- Identify influencers in social networks
- Detect potential fraud rings
- Optimize supply chains and logistics
- Improve recommendation systems
Integration with machine learning: Graph features and algorithms can enhance traditional machine learning models, providing additional context and relationship information.
Last updated:
FAQ
What’s Graph Databases by Ian Robinson about?
- Core introduction: Graph Databases by Ian Robinson introduces the fundamentals of graph databases, focusing on the labeled property graph model and how it represents and queries connected data.
- Scope and approach: The book covers graph theory basics, graph database technologies, data modeling, application development, and real-world use cases, using Neo4j and Cypher as primary examples.
- Industry impact: It highlights how graph databases transform industries like social networking, IoT, retail, and telecommunications by enabling better performance and flexibility.
- Audience: The book is aimed at developers, database professionals, and decision-makers seeking practical understanding and implementation guidance.
Why should I read Graph Databases by Ian Robinson?
- Understanding connected data: The book explains why traditional databases struggle with connected data and how graph databases excel at modeling and querying complex relationships.
- Practical guidance: Readers receive hands-on advice for graph data modeling, querying with Cypher, application architecture, and testing strategies, making it suitable for real-world projects.
- Future-proofing skills: As graph databases become mainstream, the book prepares readers to participate in this shift and innovate with graph technology.
- Industry relevance: Real-world examples and case studies demonstrate the business value and agility that graph databases bring to enterprises.
What are the key takeaways from Graph Databases by Ian Robinson?
- Graph model advantages: Graph databases offer performance, flexibility, and agility for connected data, outperforming relational and other NoSQL databases in these areas.
- Native graph processing: Concepts like index-free adjacency and native storage enable constant-time traversals and efficient querying.
- Iterative modeling: The book emphasizes iterative, test-driven development and evolving data models to match changing business needs.
- Real-world impact: Case studies show how graph databases power social recommendations, access control, logistics, and more, delivering business responsiveness and innovation.
What is the labeled property graph model in Graph Databases by Ian Robinson?
- Definition: The labeled property graph model consists of nodes (entities) and relationships (connections), both of which can have properties (key-value pairs) and labels.
- Expressiveness: Relationships are directed and named, allowing for rich semantic modeling and supporting complex, variably structured data.
- Schema flexibility: The model is schema-free and additive, enabling easy evolution of the data model without disrupting existing applications.
- Foundation for querying: This model underpins the book’s examples and is central to the power and flexibility of graph databases.
How does Graph Databases by Ian Robinson compare graph databases to relational and NoSQL databases?
- Relational limitations: Relational databases require expensive joins for connected data, leading to poor performance and rigid schemas.
- NoSQL differences: Other NoSQL stores (key-value, document, column family) lack first-class relationships, making connected data management complex and inefficient.
- Graph database strengths: Graph databases treat relationships as first-class citizens, enabling fast traversals and efficient querying of highly connected data.
- Consistency and transactions: Graph databases like Neo4j maintain ACID transactions, providing strong consistency, unlike many NoSQL stores that relax consistency for scalability.
What are the main benefits of using graph databases according to Graph Databases by Ian Robinson?
- Performance: Graph databases deliver millisecond query responses for connected data, with execution time based on the traversed subgraph rather than the entire dataset.
- Flexibility: The schema-free, additive nature allows for easy evolution of the data model, reducing migration and maintenance overhead.
- Agility: Graph databases support agile and test-driven development, enabling rapid iteration and alignment with business needs.
- Business impact: Organizations benefit from accelerated development cycles, extreme responsiveness, and the ability to model complex domains naturally.
How does Graph Databases by Ian Robinson explain graph data modeling best practices?
- Modeling approach: Entities are modeled as nodes, and their connections as relationships, closely reflecting real-world semantics.
- Iterative development: The book advocates for iterative, incremental modeling, testing with representative data, and evolving the model as requirements change.
- Avoiding anti-patterns: It warns against encoding entities as relationships and stresses explicit modeling for clarity and queryability.
- Handling complexity: Techniques for representing complex values and time (e.g., versioned graphs, timeline trees) are discussed to support evolving schemas.
How does Graph Databases by Ian Robinson teach querying with Cypher?
- Cypher introduction: Cypher is presented as an expressive, declarative query language that uses ASCII-art patterns to match graph structures.
- Core clauses: The book covers essential Cypher clauses like MATCH, WHERE, RETURN, CREATE, and MERGE, with practical examples.
- Idiomatic queries: Readers are encouraged to write idiomatic queries that start from known nodes and traverse local neighborhoods for performance.
- Optimization tips: The book advises on restricting variable-length paths and precomputing relationships for performance-critical queries.
What application architectures and development practices are recommended in Graph Databases by Ian Robinson?
- Deployment modes: Neo4j can run embedded within applications (JVM-only, low latency) or as a standalone server (platform-independent, REST API).
- Server extensions: For complex logic, server extensions allow custom Java code to run inside Neo4j, enabling domain-specific RESTful services.
- Test-driven development: The book emphasizes test-driven modeling and query development, using small graphs and unit tests for continuous validation.
- Performance testing: Strategies for application and server extension performance testing are discussed to ensure regression safety and scalability.
How does Graph Databases by Ian Robinson address production planning, performance, and scaling?
- Capacity planning: The book discusses balancing cost, performance, redundancy, and load, with advice on JVM heap sizing, page cache allocation, and disk speed.
- Clustering: Neo4j supports master-slave clustering for high availability and horizontal read scaling, with recommendations for directing writes and balancing reads.
- Cache strategies: Techniques like cache sharding and consistent routing are suggested to improve cache locality and performance.
- Consistency management: Strategies for ensuring read-your-own-write consistency in eventually consistent clusters are explained.
What real-world use cases and case studies are highlighted in Graph Databases by Ian Robinson?
- Social recommendations: Talent.net uses graph queries to infer social relations and recommend experts based on shared interests and work history.
- Authorization and access control: TeleGraph Communications manages complex access control for millions of users and resources using fine-grained permission models in a graph.
- Geospatial logistics: Global Post models parcel delivery networks as graphs with timestamped relationships, supporting route calculation and schema evolution.
- Business impact: These case studies illustrate how graph databases enable real-time analytics, agility, and business innovation.
What are the best quotes from Graph Databases by Ian Robinson and what do they mean?
- On graph power: “Graphs are truly remarkable structures... we’re only just beginning to understand how to apply them to our personal, social, and business lives.” This highlights the untapped potential of graph technology.
- On native processing: “A database engine that utilizes index-free adjacency... acts as a micro-index of other nearby nodes, which is much cheaper than using global indexes.” This explains the performance advantage of native graph databases.
- On triadic closure: “The empirical evidence... suggests that the tendency toward triadic closure is real and substantial.” This underscores the importance of social graph theory in understanding network evolution.
- On idiomatic queries: “Idiomatic queries have beneficial side effects... tend to exploit caches better and run faster than nonidiomatic queries.” This emphasizes the value of writing queries that align with graph database architecture for optimal performance.
Review Summary
Graph Databases receives mixed reviews, with an average rating of 3.63/5. Readers appreciate its introduction to graph database concepts and Neo4j, but criticize its focus on Neo4j rather than graph databases in general. The book is praised for clear explanations and real-world examples, but some find it overly enthusiastic and lacking in technical depth. Many reviewers note its value as a starter guide for those new to graph databases, while more experienced readers may find it too basic. The writing style and structure receive both positive and negative comments.
Similar Books
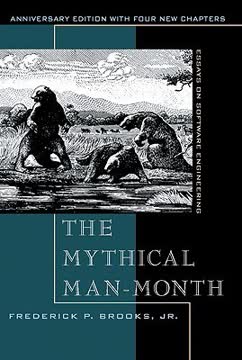
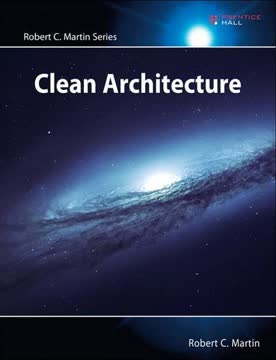
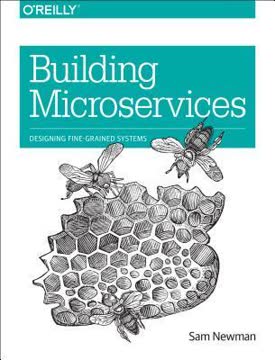
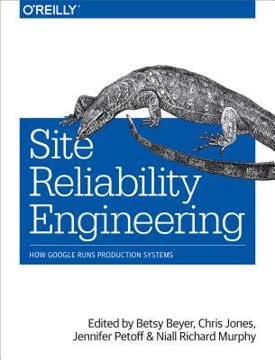
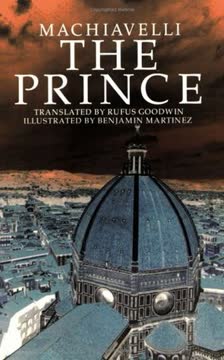
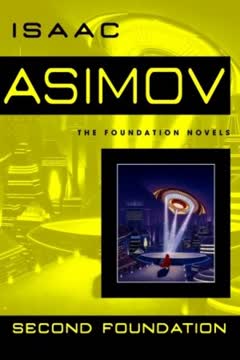
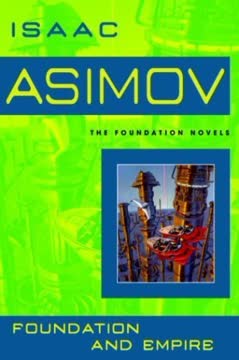
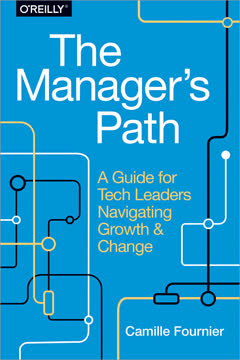
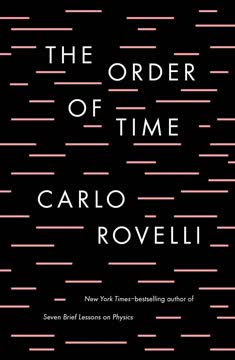
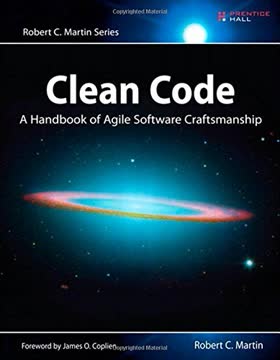
Download PDF
Download EPUB
.epub
digital book format is ideal for reading ebooks on phones, tablets, and e-readers.