Key Takeaways
1. Big Data algorithms can become Weapons of Math Destruction (WMDs)
"I came up with a name for these harmful kinds of models: Weapons of Math Destruction, or WMDs for short."
WMDs defined. Weapons of Math Destruction (WMDs) are mathematical models or algorithms that have the potential to cause significant harm to individuals and society. These models are characterized by three key features:
- Opacity: The inner workings of the model are hidden from those affected by it
- Scale: The model impacts a large number of people
- Damage: The model has negative consequences for individuals or groups
Real-world impact. WMDs can be found in various domains, including:
- Education (teacher evaluations)
- Criminal justice (recidivism prediction)
- Finance (credit scoring)
- Employment (automated hiring)
- Advertising (targeted ads)
These algorithms, while often created with good intentions, can perpetuate biases, reinforce inequalities, and make critical decisions about people's lives without proper oversight or accountability.
2. WMDs often punish the poor and reinforce inequality
"Being poor in a world of WMDs is getting more and more dangerous and expensive."
Feedback loops. WMDs often create pernicious feedback loops that disproportionately affect low-income individuals and communities. For example:
- Poor credit scores → Higher interest rates → More debt → Lower credit scores
- Living in high-crime areas → More policing → More arrests → Higher perceived crime rates
Proxies for poverty. Many WMDs use data points that serve as proxies for poverty, such as:
- Zip codes
- Education level
- Employment history
These proxies can lead to discriminatory outcomes, even when the model doesn't explicitly consider race or income.
Limited recourse. Low-income individuals often lack the resources to challenge or appeal decisions made by WMDs, further entrenching their disadvantaged position.
3. College rankings exemplify how WMDs can distort entire systems
"The U.S. News college ranking has great scale, inflicts widespread damage, and generates an almost endless spiral of destructive feedback loops."
Unintended consequences. The U.S. News & World Report college rankings, while intended to provide useful information to prospective students, have had far-reaching and often detrimental effects on higher education:
- Colleges prioritize factors that improve their ranking over educational quality
- Increased focus on standardized test scores and selectivity
- Inflated tuition costs as colleges invest in amenities to attract high-scoring students
Gaming the system. Some institutions have resorted to unethical practices to improve their rankings:
- Misreporting data
- Manipulating admissions processes
- Encouraging low-performing students to transfer before graduation
Reinforcing inequality. The rankings system tends to benefit wealthy institutions and students, while disadvantaging less resourced colleges and lower-income applicants.
4. Predatory for-profit colleges exploit vulnerable populations
"The for-profit colleges focused on the other, more vulnerable, side of the population. And the Internet gave them the perfect tool to do so."
Targeted marketing. For-profit colleges use sophisticated data analytics to target vulnerable individuals:
- Low-income communities
- Military veterans
- Single parents
- Unemployed individuals
Deceptive practices. These institutions often employ misleading tactics:
- Inflated job placement rates
- Unrealistic salary expectations
- Hidden costs and fees
Debt burden. Students at for-profit colleges often accumulate significant debt without gaining valuable credentials:
- Higher default rates on student loans
- Degrees that may not be recognized by employers
Data-driven exploitation. For-profit colleges use WMDs to:
- Identify potential students most likely to enroll
- Optimize recruitment strategies
- Maximize profit per student
5. Algorithmic hiring practices can perpetuate bias and unfairness
"Like many other WMDs, automatic systems can plow through credit scores with great efficiency and at enormous scale. But I would argue that the chief reason has to do with profits."
Proxy discrimination. Hiring algorithms often use proxies that can lead to discriminatory outcomes:
- Credit scores as a measure of responsibility
- Zip codes as indicators of reliability
- Social media activity as a predictor of job performance
Lack of context. Automated systems struggle to account for:
- Individual circumstances
- Potential for growth
- Unique qualities not captured by data points
Feedback loops. Algorithmic hiring can create self-reinforcing cycles:
- Candidates from certain backgrounds are consistently rejected
- These groups become less likely to apply or gain necessary experience
- The algorithm "learns" that these groups are less qualified
Limited recourse. Job applicants often have no way to know why they were rejected or how to improve their chances in an algorithmic system.
6. Predictive policing and sentencing models exacerbate racial disparities
"Even if a model is color blind, the result of it is anything but. In our largely segregated cities, geography is a highly effective proxy for race."
Biased inputs. Predictive policing models often rely on historical crime data, which reflects existing biases in policing practices:
- Over-policing of minority neighborhoods
- Higher arrest rates for people of color
Self-fulfilling prophecies. These models can create feedback loops:
- More policing in predicted "high-crime" areas → More arrests → Data showing more crime in those areas
Sentencing disparities. Risk assessment tools used in sentencing can perpetuate racial biases:
- Using socioeconomic factors as proxies for risk
- Failing to account for systemic inequalities
Lack of transparency. The opacity of these algorithms makes it difficult for defendants or their lawyers to challenge the assessments.
7. Targeted political advertising threatens democratic processes
"We cannot count on the free market itself to right these wrongs."
Microtargeting. Political campaigns use sophisticated data analytics to:
- Identify persuadable voters
- Tailor messages to specific demographics
- Suppress turnout among certain groups
Echo chambers. Targeted advertising can reinforce existing beliefs and polarize the electorate:
- Presenting different versions of a candidate to different voters
- Limiting exposure to diverse viewpoints
Lack of accountability. The personalized nature of targeted ads makes it difficult to:
- Track false or misleading claims
- Hold campaigns accountable for their messaging
Data privacy concerns. Campaigns collect and use vast amounts of personal data, often without voters' knowledge or consent.
8. Insurance and credit scoring systems can create damaging feedback loops
"As insurance companies learn more about us, they'll be able to pinpoint those who appear to be the riskiest customers and then either drive their rates to the stratosphere or, where legal, deny them coverage."
Individualized risk assessment. Insurance companies use increasingly granular data to assess risk:
- Driving habits (through telematics devices)
- Lifestyle choices (from social media and purchasing data)
- Genetic predispositions (from DNA tests)
Unintended consequences. These systems can lead to:
- Higher rates for vulnerable populations
- Denial of coverage for those who need it most
- Incentives for people to hide or misrepresent information
Erosion of risk pooling. The fundamental principle of insurance (spreading risk across a large group) is undermined when risk is highly individualized.
Credit score mission creep. Credit scores, originally designed for lending decisions, are now used for:
- Employment screening
- Housing applications
- Insurance pricing
This expanded use can create cycles of disadvantage for those with poor credit.
9. Workplace surveillance and optimization algorithms dehumanize workers
"When data scientists talk about 'data quality,' we're usually referring to the amount or cleanliness of the data—is there enough to train an algorithm? Are the numbers representing what we expect or are they random? But in this case we have no data quality issue; the data is available, and in fact it's plentiful. It's just wrong."
Efficiency at a cost. Workplace optimization algorithms prioritize:
- Maximum productivity
- Minimized labor costs
- Predictable staffing levels
Human impact. These systems often ignore:
- Worker well-being
- Work-life balance
- Job satisfaction
Surveillance creep. Increased monitoring of employees can lead to:
- Stress and anxiety
- Lack of autonomy
- Erosion of privacy
Algorithmic management. Workers increasingly answer to automated systems rather than human managers, leading to:
- Inflexible policies
- Lack of context in decision-making
- Difficulty in addressing unique situations or personal needs
10. Transparency and accountability are crucial for ethical use of algorithms
"To disarm WMDs, we also need to measure their impact and conduct algorithmic audits."
Algorithmic audits. Regular assessments of algorithmic systems should:
- Evaluate fairness and bias
- Test for unintended consequences
- Ensure compliance with legal and ethical standards
Explainable AI. Efforts should be made to develop algorithms that can:
- Provide clear explanations for their decisions
- Allow for human oversight and intervention
Data transparency. Individuals should have the right to:
- Access the data being used about them
- Correct inaccuracies in their data
- Understand how their data is being used
Regulatory framework. Development of laws and guidelines to govern the use of algorithmic decision-making in sensitive areas such as:
- Employment
- Criminal justice
- Financial services
- Healthcare
11. We must embed human values into algorithmic systems
"We have to explicitly embed better values into our algorithms, creating Big Data models that follow our ethical lead. Sometimes that will mean putting fairness ahead of profit."
Ethical design. Algorithms should be designed with consideration for:
- Fairness and non-discrimination
- Transparency and accountability
- Privacy protection
- Human rights
Diverse perspectives. Include a wide range of voices in the development and implementation of algorithmic systems:
- Ethicists
- Social scientists
- Community representatives
- Those affected by the algorithms
Continuous evaluation. Regularly assess the impact of algorithmic systems on:
- Individual rights and freedoms
- Social equity
- Democratic processes
Education and awareness. Promote digital literacy and understanding of algorithmic decision-making among:
- Policymakers
- Business leaders
- General public
By prioritizing these ethical considerations, we can harness the power of Big Data and algorithms while mitigating their potential for harm and ensuring they serve the broader interests of society.
Last updated:
FAQ
What's Weapons of Math Destruction about?
- Focus on Algorithms: The book examines how algorithms and mathematical models are used in decision-making processes that impact people's lives, often negatively.
- Concept of WMDs: Cathy O'Neil introduces "Weapons of Math Destruction" (WMDs) as algorithms that are opaque, unregulated, and harmful, reinforcing biases and inequalities.
- Real-World Examples: It provides case studies from sectors like education, criminal justice, and employment to illustrate the detrimental effects of these algorithms.
Why should I read Weapons of Math Destruction?
- Understanding Big Data's Impact: The book helps readers grasp the influence of big data and algorithms on modern society, highlighting their potential to undermine democracy and fairness.
- Awareness of Bias: It encourages critical evaluation of algorithms that affect daily life, from job applications to credit scores, emphasizing the importance of recognizing embedded biases.
- Call to Action: O'Neil urges readers to advocate for transparency and accountability in algorithm use, stressing the need for ethical considerations in data science and policy-making.
What are the key takeaways of Weapons of Math Destruction?
- WMD Characteristics: WMDs are defined by their opacity, scalability, and damaging effects, allowing them to operate without accountability and disproportionately affect disadvantaged groups.
- Feedback Loops: The book discusses how WMDs create self-reinforcing feedback loops that perpetuate inequality, using biased data to make decisions that entrench those biases.
- Need for Reform: O'Neil calls for reform in algorithm development and use, emphasizing fairness and transparency, and advocating for ethical data practices.
What are the best quotes from Weapons of Math Destruction and what do they mean?
- “Models are opinions embedded in mathematics.”: This quote highlights that mathematical models reflect the biases and assumptions of their creators, stressing the need to scrutinize data-driven decisions.
- “The most dangerous [algorithms] are also the most secretive.”: O'Neil warns about the risks of relying on opaque systems without understanding their workings, emphasizing the need for transparency.
- “WMDs tend to punish the poor.”: This statement underscores the disproportionate harm algorithms cause to marginalized communities, highlighting the social justice implications of data-driven policies.
What is a "Weapon of Math Destruction" (WMD) according to Cathy O'Neil?
- Definition of WMD: A WMD is an algorithm that is opaque, unregulated, and harmful, often operating without accountability and perpetuating existing inequalities.
- Characteristics of WMDs: They are opaque (difficult to understand), scalable (affecting large populations), and damaging (causing harm to individuals and communities).
- Examples of WMDs: The book cites algorithms in hiring, credit scoring, and predictive policing as examples of WMDs leading to unjust outcomes for vulnerable populations.
How do WMDs create feedback loops?
- Self-Reinforcing Mechanisms: WMDs generate data that reinforces their conclusions, creating cycles of harm, such as biased hiring practices leading to more biased data.
- Impact on Individuals: Affected individuals may find themselves trapped in cycles of disadvantage, with models penalizing them based on flawed assumptions.
- Examples in Society: Feedback loops are prevalent in sectors like education and criminal justice, exacerbating inequalities and making it difficult for individuals to escape their circumstances.
What role does bias play in WMDs?
- Embedded Bias: Biases are often embedded in the data used to train algorithms, leading to unfair outcomes and perpetuating societal prejudices.
- Consequences of Bias: Bias in WMDs can result in discriminatory practices in hiring, lending, and law enforcement, disproportionately affecting marginalized groups.
- Need for Awareness: Recognizing bias in algorithms is crucial for advocating fairer practices, emphasizing transparency and accountability in data-driven decision-making.
How does Weapons of Math Destruction address the education system?
- Teacher Evaluations: O'Neil discusses value-added models in teacher evaluations, which can lead to the firing of effective teachers based on flawed data.
- Impact on Students: WMDs in education can harm students by removing qualified teachers and perpetuating inequities in underfunded schools.
- Call for Reform: The book advocates for reevaluating educational data use, emphasizing models that prioritize student outcomes and fairness.
What are the implications of WMDs in the criminal justice system?
- Predictive Policing: Algorithms in predictive policing often target marginalized communities based on historical crime data, leading to over-policing and systemic biases.
- Recidivism Models: Recidivism models can unfairly penalize individuals based on backgrounds, perpetuating cycles of incarceration with biased data.
- Need for Ethical Considerations: O'Neil stresses the importance of ethical considerations in algorithm development and implementation in the justice system.
How does Weapons of Math Destruction illustrate the concept of feedback loops?
- Cycle of Inequality: WMDs create feedback loops that reinforce existing inequalities, such as low credit scores leading to higher costs and perpetuating poverty.
- Education and Employment: Flawed evaluation models can lead to job losses for teachers, affecting their ability to support students effectively.
- Criminal Justice: Biased algorithms can lead to harsher sentences, further entrenching individuals in cycles of crime and poverty.
What solutions does Cathy O'Neil propose for addressing WMDs?
- Regulatory Frameworks: O'Neil advocates for regulations governing algorithm use, ensuring fairness and accountability similar to other industries.
- Transparency and Audits: The book emphasizes the need for transparency in algorithmic processes and regular audits to assess their impact on populations.
- Public Awareness and Advocacy: O'Neil encourages readers to become informed about algorithms affecting their lives and to advocate for equitable changes.
What is the significance of the term "Simpson's Paradox" in Weapons of Math Destruction?
- Statistical Misinterpretation: Simpson's Paradox illustrates how aggregated data can present misleading pictures, masking true trends within subgroups.
- Example in Education: The book references the A Nation at Risk report's misinterpretation of SAT scores, leading to flawed conclusions about educational quality.
- Implications for Policy: This concept underscores the importance of disaggregating data to understand real issues, particularly when crafting policies affecting vulnerable populations.
Review Summary
Weapons of Math Destruction exposes the dark side of big data algorithms, highlighting how they can reinforce inequality and bias. While some praise O'Neil's accessible writing and important message, others find her arguments oversimplified. The book covers various sectors where algorithms impact lives, from education to criminal justice. Readers appreciate O'Neil's expertise and timely insights, though some desire more technical depth. Overall, the book sparks crucial discussions about the ethical implications of data-driven decision-making in modern society.
Similar Books
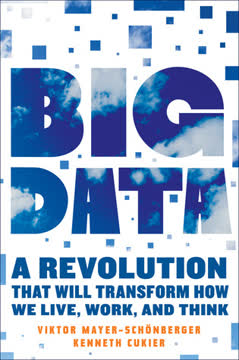
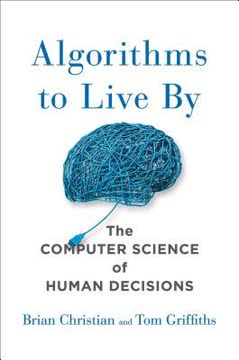
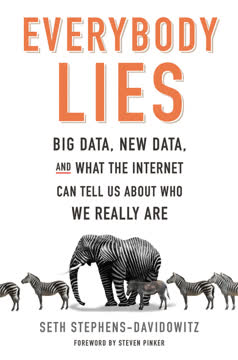
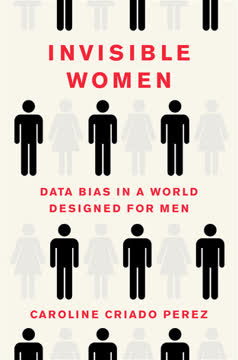
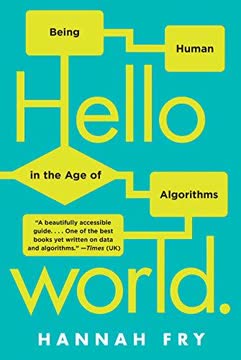
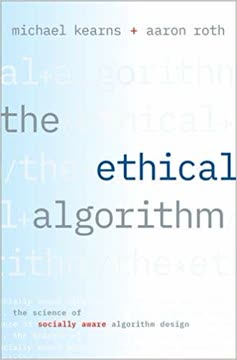
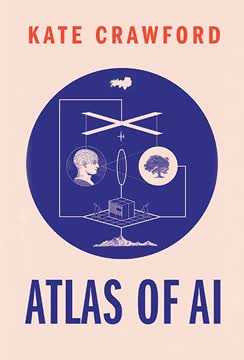
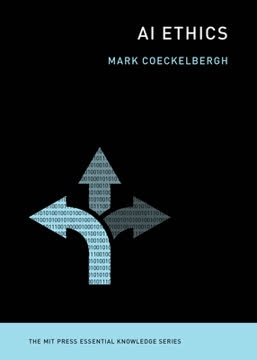
Download PDF
Download EPUB
.epub
digital book format is ideal for reading ebooks on phones, tablets, and e-readers.