نکات کلیدی
1. انتخاب مدل نیازمند تعادل بین عملکرد، پیچیدگی و محدودیتهای عملی است
"سادگی سه هدف را دنبال میکند. اول، مدلهای سادهتر راحتتر پیادهسازی میشوند و پیادهسازی زودهنگام مدل شما به شما این امکان را میدهد که تأیید کنید که خط لوله پیشبینی شما با مجموعه دادههای آموزشیتان سازگار است. دوم، شروع با چیزی ساده و افزودن اجزای پیچیدهتر بهصورت مرحلهای، درک مدل شما و اشکالزدایی آن را آسانتر میکند. سوم، سادهترین مدل بهعنوان یک مبنای مقایسه برای مدلهای پیچیدهتر شما عمل میکند."
تجارت عملکرد در مقابل پیچیدگی. هنگام انتخاب یک مدل، به موارد زیر توجه کنید:
- عملکرد مدل (دقت، نمره F1 و غیره)
- نیازهای محاسباتی (زمان آموزش، سرعت استنتاج)
- قابلیت تفسیر
- مقدار دادههای آموزشی مورد نیاز
- سهولت در پیادهسازی و نگهداری
با مدلهای سادهای مانند رگرسیون لجستیک یا درختهای تصمیمگیری شروع کنید قبل از اینکه به شبکههای عصبی پیچیدهتر بروید. این به شما این امکان را میدهد که:
- یک معیار عملکرد تعیین کنید
- خط لوله دادهها و چارچوب مسئله خود را تأیید کنید
- درک کنید که چگونه ویژگیها بر پیشبینیها تأثیر میگذارند
از دام مدلهای پیشرفته بپرهیزید. جدیدترین مدلها ممکن است برای مورد استفاده خاص شما بهینه نباشند. به موارد زیر توجه کنید:
- ویژگیها و حجم دادههای شما
- محدودیتهای محاسباتی
- نیاز به توضیحپذیری
- محیط پیادهسازی
2. روشهای تجمیع میتوانند بهطور قابلتوجهی عملکرد مدل را افزایش دهند
"در ۲۰ مورد از ۲۲ راهحل برنده در مسابقات کگل در سال ۲۰۲۱، تا آگوست ۲۰۲۱، از روشهای تجمیع استفاده شده است."
انواع تجمیع:
- باگینگ: آموزش مدلها بر روی مجموعه دادههای بوتاسترپ شده (مانند جنگلهای تصادفی)
- تقویت: آموزش مدلها بهصورت متوالی و تمرکز بر روی نمونههای اشتباه طبقهبندی شده (مانند XGBoost)
- استکینگ: استفاده از پیشبینیهای مدلهای پایه بهعنوان ورودی برای یک یادگیرنده متا
تجمیعها با ترکیب مدلهای متنوع برای کاهش تعصب و واریانس کار میکنند. نکات کلیدی:
- از مدلهای پایه غیرهمبسته برای حداکثر بهرهوری استفاده کنید
- افزایش عملکرد را در مقابل پیچیدگی بیشتر متعادل کنید
- در مسابقات محبوب است اما ممکن است در تولید چالشبرانگیز باشد
روشهای تجمیع مانند XGBoost و جنگلهای تصادفی معمولاً عملکرد قویای را بهطور پیشفرض برای بسیاری از مسائل، بهویژه با دادههای ساختاریافته، ارائه میدهند.
3. AutoML و تنظیم هایپرپارامترها، بهینهسازی مدل را خودکار میکنند
"بهجای اینکه به یک گروه از ۱۰۰ محقق/مهندس ML پول بدهید تا با مدلهای مختلف بازی کنند و در نهایت یک مدل زیر بهینه را انتخاب کنند، چرا این پول را برای جستجوی مدل بهینه صرف نکنید؟"
سطوح AutoML:
- تنظیم هایپرپارامترها: بهینهسازی هایپرپارامترهای مدل
- جستجوی معماری عصبی: کشف معماریهای بهینه شبکههای عصبی
- بهینهسازهای یادگرفته شده: استفاده از ML برای یادگیری الگوریتمهای بهینهسازی
تنظیم هایپرپارامترها، قابلدسترسترین و رایجترین شکل AutoML است. رویکردهای متداول:
- جستجوی تصادفی
- جستجوی شبکهای
- بهینهسازی بیزی
مزایای AutoML:
- کاهش تلاش دستی در توسعه مدل
- میتواند پیکربندیهای غیرقابلانتظار مدل را کشف کند
- به غیرمتخصصان این امکان را میدهد که بهطور مؤثر از ML استفاده کنند
چالشها شامل هزینههای بالای محاسباتی و احتمال بیشبرازش به مجموعه اعتبارسنجی است. از AutoML با احتیاط استفاده کنید و همیشه عملکرد نهایی را بر روی یک مجموعه آزمایشی جداگانه ارزیابی کنید.
4. آموزش توزیعشده امکان مقیاسپذیری به مجموعه دادهها و مدلهای بزرگ را فراهم میکند
"با بزرگتر و منابعبرتر شدن مدلها، شرکتها بیشتر به آموزش در مقیاس اهمیت میدهند."
چالشهای آموزش توزیعشده:
- دادههایی که در حافظه جا نمیشوند
- هماهنگی بهروزرسانیها در چندین ماشین
- تعادل بین محاسبات و هزینههای ارتباطی
تکنیکهای آموزش در مقیاس بزرگ:
- نقطهچکانی گرادیان: مبادله محاسبات برای صرفهجویی در حافظه
- آموزش با دستههای بزرگ: استفاده مؤثر از چندین GPU/TPU
- دقت مختلط: استفاده از حسابهای با دقت پایینتر برای تسریع آموزش
آموزش توزیعشده نیازمند توجه دقیق به موارد زیر است:
- تنظیمات سختافزاری (GPUها، ارتباطات پرسرعت)
- چارچوبهای نرمافزاری (PyTorch Distributed، Horovod)
- الگوریتمهای بهینهسازی (SGD همزمان در مقابل غیرهمزمان)
با رشد مدلها (مانند مدلهای زبانی بزرگ مانند GPT-3)، آموزش توزیعشده برای پیشبرد مرزهای قابلیتهای ML ضروری میشود.
5. ردیابی آزمایش و نسخهبندی برای تکرارپذیری حیاتی است
"این سناریو را تصور کنید. شما و تیمتان چند هفته گذشته را صرف تنظیم مدل خود کردهاید و یکی از اجراها در نهایت نتایج امیدوارکنندهای نشان داد. شما میخواستید از آن برای آزمایشهای گستردهتر استفاده کنید، بنابراین سعی کردید آن را با استفاده از مجموعهای از هایپرپارامترهایی که جایی یادداشت کرده بودید، تکرار کنید، فقط برای اینکه متوجه شوید نتایج چندان یکسان نیستند."
عناصر کلیدی برای ردیابی:
- نسخههای کد
- نسخههای داده و مراحل پیشپردازش
- معماری مدل و هایپرپارامترها
- محیط آموزشی (سختافزار، نسخههای نرمافزاری)
- معیارها و آثار ارزیابی (نمودارهای از دست دادن، نقاط چک مدل)
ابزارهای ردیابی آزمایش:
- MLflow
- Weights & Biases
- DVC (کنترل نسخه داده)
مزایای ردیابی دقیق:
- تکرارپذیری نتایج
- همکاری آسانتر درون تیمها
- توانایی مقایسه آزمایشها و شناسایی تغییرات مؤثر
چالشها شامل نسخهبندی مجموعه دادههای بزرگ و مدیریت عدمقطعیت در آموزش است. پروتکلهای واضحی برای مستندسازی آزمایشها درون تیم خود ایجاد کنید.
6. موازیسازی داده و موازیسازی مدل رویکردهای متفاوتی برای آموزش توزیعشده ارائه میدهند
"رایجترین روش موازیسازی، موازیسازی داده است: شما دادههای خود را در چندین ماشین تقسیم میکنید، مدل خود را بر روی همه آنها آموزش میدهید و گرادیانها را جمعآوری میکنید."
موازیسازی داده:
- هر ماشین یک نسخه کامل از مدل را دارد
- دادهها در بین ماشینها تقسیم میشوند
- گرادیانها برای بهروزرسانی مدل جهانی تجمیع میشوند
مزایا:
- پیادهسازی آسانتر
- مقیاسپذیری خوب با اندازه مجموعه داده
معایب:
- محدود به اندازه مدل که بر روی یک دستگاه جا میشود
- هزینههای ارتباطی برای همگامسازی گرادیان
موازیسازی مدل:
- مدل در بین چندین دستگاه تقسیم میشود
- هر دستگاه بخشی از مدل را پردازش میکند
مزایا:
- میتواند مدلهای بسیار بزرگ را مدیریت کند
- نیاز به حافظه در هر دستگاه را کاهش میدهد
معایب:
- پیادهسازی پیچیدهتر
- ممکن است منجر به استفاده ناکافی از دستگاه شود
رویکردهای ترکیبی مانند موازیسازی خط لوله عناصر هر دو را برای بهینهسازی استفاده از منابع ترکیب میکنند. بهترین رویکرد بستگی به معماری خاص مدل و محدودیتهای سختافزاری شما دارد.
7. سیستمهای مؤثر ML معمولاً با سادگی شروع میشوند و بهتدریج در طول زمان پیچیدهتر میشوند
"چهار مرحله برای پذیرش ML وجود دارد. راهحلهای یک مرحله میتوانند بهعنوان مبنایی برای ارزیابی راهحلهای مرحله بعدی استفاده شوند."
چهار مرحله پذیرش ML:
- قبل از ML: استفاده از هورستیکها و سیستمهای مبتنی بر قوانین
- سادهترین مدلهای ML: پیادهسازی الگوریتمهای پایه (مانند رگرسیون لجستیک)
- بهینهسازی مدلهای ساده: مهندسی ویژگی، تجمیع، تنظیم هایپرپارامترها
- سیستمهای پیچیده: یادگیری عمیق، AutoML، معماریهای سفارشی
مزایای این رویکرد تدریجی:
- مبنای مقایسهای برای ارزیابی ایجاد میکند
- زیرساختهای لازم را بهتدریج میسازد
- میوههای پایین را برای بهبود شناسایی میکند
با تعریف واضح مشکل و معیارهای موفقیت خود شروع کنید. یک مدل ساده که ۸۰٪ از مشکل شما را حل میکند ممکن است در ابتدا کافی باشد. با جمعآوری دادهها و بینشهای بیشتر، بهتدریج پیچیدگی مدل را افزایش دهید تا چالشهای باقیمانده را برطرف کنید.
به یاد داشته باشید که ML همیشه پاسخ نیست – گاهی اوقات هورستیکهای ساده یا رویکردهای مهندسی نرمافزار سنتی ممکن است مناسبتر و قابلنگهداریتر باشند.
آخرین بهروزرسانی::
FAQ
What's "Designing Machine Learning Systems" about?
- Comprehensive Guide: "Designing Machine Learning Systems" by Chip Huyen is a comprehensive guide to building production-ready machine learning applications. It covers the entire lifecycle of machine learning systems, from data engineering to deployment and monitoring.
- Iterative Process: The book emphasizes an iterative process for developing machine learning systems, highlighting the importance of continuous improvement and adaptation to changing environments and requirements.
- Real-World Challenges: It addresses real-world challenges in deploying machine learning systems, such as data management, scalability, and ethical considerations, providing practical solutions and insights.
- Focus on Production: Unlike many other resources, this book focuses on the practical aspects of deploying machine learning systems in production, making it a valuable resource for practitioners in the field.
Why should I read "Designing Machine Learning Systems"?
- Practical Insights: The book offers practical insights into the challenges and solutions of deploying machine learning systems in production, which are often overlooked in academic settings.
- Comprehensive Coverage: It covers a wide range of topics, from data engineering and feature engineering to model development and deployment, providing a holistic view of the machine learning lifecycle.
- Real-World Examples: The author uses real-world examples and case studies to illustrate key concepts, making the content relatable and applicable to real-world scenarios.
- Expert Author: Chip Huyen is an experienced engineer and educator, bringing her expertise in machine learning systems design to the book, making it a credible and authoritative resource.
What are the key takeaways of "Designing Machine Learning Systems"?
- Iterative Development: Machine learning systems should be developed iteratively, with continuous monitoring and updates to adapt to changing data and requirements.
- Data is Crucial: The success of machine learning systems heavily depends on the quality and quantity of data, emphasizing the importance of data engineering and management.
- System Design: Designing machine learning systems involves considering various components, including algorithms, data, infrastructure, and hardware, to meet specified requirements.
- Ethical Considerations: The book highlights the importance of addressing ethical and societal challenges, such as fairness and interpretability, in deploying machine learning systems.
What are the best quotes from "Designing Machine Learning Systems" and what do they mean?
- "Data is profoundly dumb." This quote emphasizes the importance of intelligent design and the limitations of relying solely on data for machine learning systems.
- "Machine learning is not a magic tool that can solve all problems." It highlights the need for careful consideration of when and how to use machine learning, rather than viewing it as a one-size-fits-all solution.
- "Complex ML systems are made up of simpler building blocks." This underscores the importance of understanding and mastering the fundamental components of machine learning systems to build more complex applications.
- "The vast majority of ML-related jobs will be, and already are, in productionizing ML." This quote reflects the growing demand for skills in deploying and maintaining machine learning systems in production environments.
How does "Designing Machine Learning Systems" approach data engineering?
- Data Importance: The book emphasizes the critical role of data in building successful machine learning systems, highlighting the need for effective data management and processing.
- Data Sources: It discusses various data sources, including user input, system-generated data, and third-party data, and their implications for machine learning systems.
- Data Formats: The book covers different data serialization formats, such as JSON, CSV, and Parquet, and their suitability for different use cases.
- ETL Process: It explains the Extract, Transform, Load (ETL) process, which is essential for preparing data for machine learning applications.
What is the iterative process for designing machine learning systems in "Designing Machine Learning Systems"?
- Project Scoping: The process begins with scoping the project, defining goals, objectives, constraints, and evaluation criteria.
- Data Engineering: It involves processing and manipulating data to create training datasets, which is crucial for model development.
- Model Development: This step includes generating features, training models, optimizing them, and evaluating their performance.
- Deployment and Monitoring: After deployment, models need to be monitored for performance decay and updated to adapt to changing environments and requirements.
How does "Designing Machine Learning Systems" address model development and evaluation?
- Model Selection: The book discusses how to select the best model for a problem, considering factors like performance, interpretability, and computational requirements.
- Training Techniques: It covers training techniques, including distributed training and experiment tracking, to handle large-scale models and datasets.
- Evaluation Methods: The book emphasizes the importance of robust evaluation methods, such as perturbation tests and slice-based evaluation, to ensure model reliability and fairness.
- AutoML: It explores the use of automated machine learning (AutoML) for hyperparameter tuning and architecture search to optimize model performance.
What are the challenges of deploying machine learning systems in production according to "Designing Machine Learning Systems"?
- Scalability: Machine learning systems must be scalable to handle large volumes of data and traffic, requiring efficient resource management and infrastructure.
- Reliability: The systems should be reliable, performing correctly even in the face of hardware or software faults and human errors.
- Ethical Challenges: Deploying machine learning systems involves addressing ethical challenges, such as fairness, interpretability, and bias, to ensure responsible AI use.
- Data Management: Effective data management is crucial, as data in production is often messy, constantly shifting, and subject to privacy and regulatory concerns.
How does "Designing Machine Learning Systems" handle feature engineering?
- Feature Importance: The book discusses the importance of feature engineering in improving model performance and provides techniques for handling missing values, scaling, and encoding categorical features.
- Data Leakage: It highlights the risk of data leakage, where information from the future or test data leaks into the training process, and provides strategies to detect and prevent it.
- Feature Generalization: The book emphasizes the need for features that generalize well to unseen data, considering factors like feature coverage and distribution.
- Cross Features: It covers the creation of cross features to model non-linear relationships between variables, which can enhance model performance.
What is the role of AutoML in "Designing Machine Learning Systems"?
- Hyperparameter Tuning: AutoML is used for hyperparameter tuning, automating the process of finding the optimal set of hyperparameters for a given model.
- Architecture Search: The book explores neural architecture search (NAS), which automates the design of model architectures by searching for the best configuration of building blocks.
- Learned Optimizers: It discusses the concept of learned optimizers, which replace traditional learning algorithms with neural networks to improve model training.
- Efficiency and Performance: AutoML techniques, such as EfficientNets, demonstrate significant improvements in model accuracy and efficiency, making them valuable for production systems.
How does "Designing Machine Learning Systems" address ethical considerations in machine learning?
- Fairness: The book emphasizes the importance of fairness in machine learning systems, highlighting the need to address biases and ensure equitable treatment of all users.
- Interpretability: It discusses the need for interpretability in machine learning models, enabling users to understand and trust the decisions made by the system.
- Bias Detection: The book provides methods for detecting and mitigating biases in machine learning models, such as invariance tests and slice-based evaluation.
- Societal Impact: It highlights the potential societal impact of machine learning systems, urging practitioners to consider the ethical implications of their work.
What are the best practices for deploying machine learning systems in production according to "Designing Machine Learning Systems"?
- Iterative Development: The book advocates for an iterative development process, with continuous monitoring and updates to adapt to changing data and requirements.
- Scalable Infrastructure: It emphasizes the need for scalable infrastructure to handle large volumes of data and traffic, ensuring efficient resource management.
- Robust Evaluation: The book highlights the importance of robust evaluation methods, such as perturbation tests and model calibration, to ensure model reliability and fairness.
- Ethical Considerations: It stresses the importance of addressing ethical challenges, such as fairness and interpretability, to ensure responsible AI use in production systems.
نقد و بررسی
کتاب طراحی سیستمهای یادگیری ماشین به خاطر پوشش جامع خود از MLOps و پیادهسازی عملی سیستمهای ML مورد تحسین فراوان قرار گرفته است. خوانندگان به تمرکز آن بر مفاهیم به جای ابزارهای خاص توجه دارند و این ویژگی آن را برای هم مبتدیان و هم متخصصان با تجربه ارزشمند میسازد. این کتاب به مباحث مهندسی داده، مهندسی ویژگیها، استقرار مدل و نظارت میپردازد و بینشهایی درباره چالشهای دنیای واقعی ارائه میدهد. بسیاری از منتقدان آن را خواندنی ضروری برای دانشمندان داده و مهندسان ML میدانند و به ارتباط آن با شیوههای جاری صنعت و تأکیدش بر طراحی سیستمهای ML از ابتدا تا انتها اشاره میکنند.
Similar Books
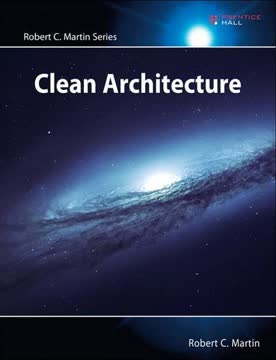
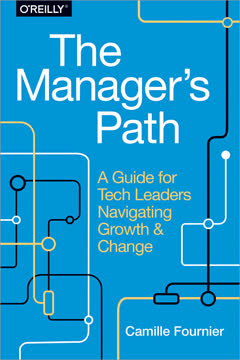
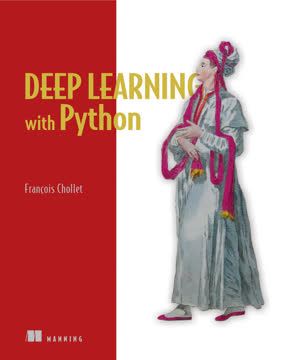
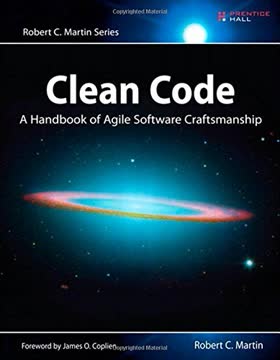
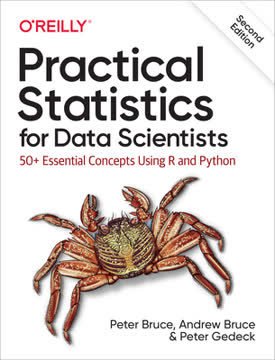
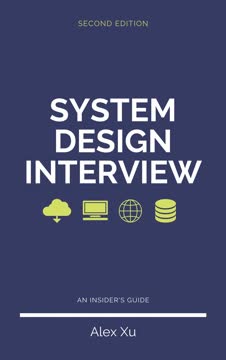
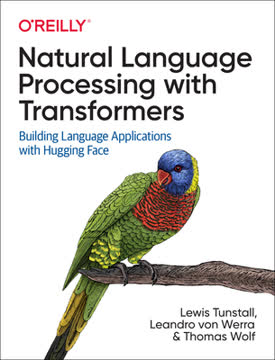
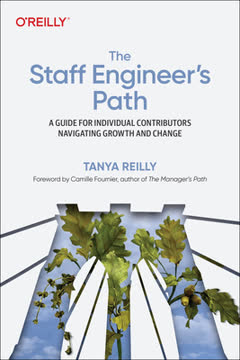