重点摘要
1. 一切皆可测量,即使是无形的事物
只要你能以任何方式观察到它,它就适合某种测量方法。
测量是减少不确定性。 与普遍看法相反,测量并不需要完美的精确度或确定性。它只是意味着减少对感兴趣数量的不确定性。这适用于有形的事物,如物理对象,也适用于无形的概念,如客户满意度或项目风险。
可观察的后果。 任何重要的无形事物都必须有可观察的后果。例如,如果你声称员工士气影响生产力,那么必须有某种方法通过生产力指标检测士气的变化。通过识别这些可观察的效果,我们可以间接测量无形事物。
存在实用方法。 许多看似无法测量的事物已经被某些人测量过,通常使用令人惊讶的简单方法。例子包括:
- 在不排干湖水的情况下估算鱼类数量
- 测量品牌损害的经济影响
- 为政策决策量化人类生命的价值
2. 测量减少不确定性以做出更好的决策
测量很重要,因为它必须对决策和行为产生某种可想象的影响。
决策驱动的测量。 测量的目的是为决策提供信息。在测量之前,明确界定所涉及的决策以及额外信息将如何影响它。这有助于优先考虑测量什么以及如何精确测量。
不确定性和风险。 决策涉及不确定性,这会产生风险。测量减少不确定性,从而减轻风险。关键概念:
- 不确定性:缺乏完全确定性;存在多种可能性
- 风险:一种不确定状态,其中一些可能性涉及损失或不良结果
信息的价值。 并非所有测量都同等有价值。计算完美信息的预期价值(EVPI)以确定测量的价值:
- 确定决策和可能的结果
- 估计每个结果的概率和后果
- 计算有无完美信息的预期价值
- 差值即为EVPI,这是你应该在测量上花费的最大金额
3. 校准你的估计以提高准确性
成功是坚持不懈和顽强努力的函数,愿意花二十二分钟弄清楚大多数人在三十秒后就会放弃的事情。
过度自信很常见。 大多数人对他们的估计过于自信,提供的范围太窄。这导致基于不现实期望的糟糕决策。
校准训练。 通过练习和反馈,人们可以学会提供更准确的概率估计。技术包括:
- 等价赌注测试:将你的估计与已知赔率的赌注进行比较
- 考虑相反情况:积极寻找你可能出错的原因
- 使用参考类:与类似的已知数量进行比较
- 通过反馈练习:参加校准测试并查看结果
校准的好处。 校准良好的估计者:
- 为决策模型提供更可靠的输入
- 更愿意接受新信息并改变他们的想法
- 在各个领域做出更好的预测
4. 使用五规则快速了解人口特征
在任何随机抽取的五个样本中,人口中位数在最小值和最大值之间的概率为93.75%。
简单但强大。 五规则允许在最少数据的情况下快速估计人口特征。它适用于任何类型的人口,从果冻豆的重量到客户满意度评分。
如何应用:
- 从人口中随机抽取五个样本
- 记录样本中的最小值和最大值
- 你可以93.75%地确信人口中位数在这两个值之间
限制和扩展:
- 提供关于中位数的信息,而不是平均值
- 为了更精确的估计,增加样本量(参见书中的无数学表)
- 结合其他方法进行更全面的分析
5. 将复杂问题分解为可测量的组件
如果你不知道测量什么,那就测量吧。你会学到该测量什么。
分解它。 当面对一个看似无法测量的问题时,将其分解为更小、更易管理的组件。这通常会揭示更容易测量或估计的方面。
费米问题。 以物理学家恩里科·费米命名,这种方法通过将难以测量的数量分解为更容易估计的因素来进行粗略估计。例子:
估算芝加哥的钢琴调音师数量:
- 估算芝加哥的人口
- 估算有钢琴的家庭比例
- 估算钢琴需要调音的频率
- 估算调音师每天可以服务的钢琴数量
- 结合这些估计得出最终答案
分解的好处:
- 减少整体估计误差
- 揭示哪些组件对不确定性贡献最大
- 确定哪些特定领域的额外数据最有价值
6. 应用贝叶斯思维用新数据更新信念
当你能测量你所谈论的东西,并用数字表达它时,你就对它有所了解;但当你不能用数字表达它时,你的知识是贫乏和不令人满意的。
先验和后验概率。 贝叶斯分析提供了一个基于新证据更新信念的框架:
- 从先验概率(初始信念)开始
- 收集新数据
- 计算在不同假设下数据的可能性
- 使用贝叶斯定理将先验更新为后验概率
贝叶斯方法的优势:
- 包含现有知识
- 允许随着新信息的到来进行增量更新
- 提供一种自然的方式来表达不确定性
实际应用:
- 医学诊断:根据测试结果更新疾病概率
- 质量控制:通过检查数据细化缺陷率估计
- 项目管理:随着工作进展调整时间表和预算
7. 小样本可以提供有价值的信息
如果你几乎一无所知,几乎任何事情都会告诉你一些东西。
初始数据的价值。 当从高度不确定的状态开始时,即使是小样本也能提供显著的见解。最初的几次观察通常每个数据点提供最多的信息。
收益递减。 随着样本量的增加,每个额外观察的边际价值通常会减少。这一原则有助于指导高效的数据收集:
- 从小样本开始以快速获取见解
- 根据信息价值逐步增加样本量
- 当额外数据的成本超过其收益时停止
小样本的方法:
- 学生t分布:用于估计样本量小至2的总体参数
- 非参数方法:不假设特定人口分布的技术
- 贝叶斯更新:结合先验知识充分利用有限数据
8. 量化额外信息的价值
顿悟方程:信息价值如何改变一切。
信息的预期价值(EVI)。 在收集信息之前计算其价值:
- 建模决策和可能的结果
- 估计当前的概率和收益
- 计算当前信息的预期价值
- 计算完美信息的预期价值
- 差值即为完美信息的预期价值(EVPI)
- 估计测量将减少多少不确定性
- 将EVPI乘以减少的不确定性比例得到EVI
测量倒置。 通常,最有价值的测量是那些很少被考虑的,而常规测量的项目提供的决策价值很小。原因包括:
- 熟悉偏见:测量容易或传统的东西
- 对已知领域的过度自信
- 忽视高影响、高不确定性因素
迭代方法。 从粗略估计开始,根据信息价值进行细化:
- 确定决策中的关键不确定性
- 估计每个不确定性的信
最后更新日期:
FAQ
What's How to Measure Anything about?
- Measuring Intangibles: The book by Douglas W. Hubbard focuses on the idea that anything, including intangibles like employee morale or the value of information, can be measured.
- Decision-Oriented Framework: It presents a universal approach to measurement that emphasizes the importance of measurements in informing decisions.
- Practical Applications: Hubbard shares real-world examples from various fields to illustrate how measurement can lead to better decision-making.
Why should I read How to Measure Anything?
- Overcoming Measurement Myths: The book is essential for anyone who believes that certain aspects of their business or life cannot be quantified.
- Improving Decision-Making: By learning the methods outlined, readers can enhance their decision-making processes.
- Accessible to All: Hubbard's writing is designed to be accessible to non-experts, making complex statistical concepts understandable.
What are the key takeaways of How to Measure Anything?
- Measurement is Possible: Hubbard emphasizes that even the most elusive intangibles can be quantified with the right approach.
- Applied Information Economics (AIE): Introduces a structured approach to measurement focusing on defining decisions and assessing uncertainty.
- Calibration of Estimates: Discusses the importance of calibrating estimates to improve accuracy in decision-making.
What are the best quotes from How to Measure Anything and what do they mean?
- “Anything can be measured.”: Encourages readers to rethink their assumptions about what can and cannot be quantified.
- “It’s better to be approximately right than to be precisely wrong.”: Highlights the importance of making informed estimates rather than waiting for perfect data.
- “If you understand it, you can model it.”: Emphasizes the connection between understanding a concept and the ability to measure it.
What is the Applied Information Economics (AIE) method in How to Measure Anything?
- Structured Measurement Approach: AIE is a systematic method for measuring uncertainties and making decisions based on those measurements.
- Five-Step Process: Consists of defining the decision, determining current knowledge, computing the value of additional information, applying relevant measurement instruments, and making a decision.
- Focus on Uncertainty Reduction: Treats measurement as a way to reduce uncertainty, allowing for more informed choices.
How does Douglas W. Hubbard define measurement in How to Measure Anything?
- Reduction of Uncertainty: Measurement is defined as "a quantitatively expressed reduction of uncertainty based on one or more observations."
- Probabilistic Nature: Acknowledges that measurements do not need to eliminate uncertainty entirely; they simply need to reduce it.
- Observable Consequences: Tied to observable outcomes, meaning that if something can be detected in any way, it can be measured.
What is the Monte Carlo method mentioned in How to Measure Anything?
- Simulation Technique: A statistical technique that uses random sampling to estimate the probability of different outcomes in a process that cannot easily be predicted.
- Application in Risk Analysis: Allows decision-makers to model uncertainty and assess risks by simulating thousands of scenarios based on varying inputs.
- Historical Context: Named after the Monte Carlo Casino due to its reliance on random sampling, similar to games of chance.
What is the Expected Value of Information (EVI) in How to Measure Anything?
- Definition of EVI: The reduction in expected opportunity loss (EOL) that results from obtaining additional information before making a decision.
- Importance in Decision-Making: Helps prioritize measurement efforts by quantifying the potential benefits of reducing uncertainty.
- Calculation: Calculated as the difference between the EOL before and after obtaining new information.
How does How to Measure Anything address biases in decision-making?
- Human Biases: Discusses common human biases, such as overconfidence and selection bias, that can affect decision-making.
- Calibration Techniques: Introduces calibration techniques to help individuals better assess their own uncertainty and improve their estimates.
- Importance of Training: Emphasizes the need for training to mitigate biases and enhance the accuracy of decision-making processes.
What is the significance of Bayesian analysis in How to Measure Anything?
- Updating Beliefs: Allows decision-makers to update their beliefs based on new evidence, integrating prior knowledge with new information.
- Practical Applications: Provides examples of how Bayesian methods can be applied to real-world problems, enhancing the understanding of uncertainty.
- Comparison to Traditional Methods: Contrasts Bayesian methods with traditional statistical approaches, highlighting their flexibility and applicability in uncertain situations.
How does Hubbard define the value of information in How to Measure Anything?
- Expected Value of Perfect Information (EVPI): Defined as the maximum amount a decision-maker should be willing to pay for information that would eliminate uncertainty.
- Cost-Benefit Analysis: Assessed through a cost-benefit lens, weighing the potential benefits of reducing uncertainty against the costs of obtaining that information.
- Real-World Examples: Provides case studies where the value of information was calculated, demonstrating its practical significance.
How can I apply the concepts from How to Measure Anything in my work?
- Identify Key Decisions: Start by clearly defining the decisions you need to make and the uncertainties involved.
- Use Bayesian Methods: Implement Bayesian analysis to refine your estimates as new data becomes available.
- Measure Incrementally: Apply the principle of incremental measurement by starting with small, manageable data collection efforts.
评论
《如何衡量一切》评价褒贬不一。许多人称赞其在量化无形资产和减少决策不确定性方面的见解。读者欣赏书中提供的实用工具、统计概念和历史实例。然而,有些人觉得这本书内容密集、重复且过于注重数学。批评者认为它过于简化复杂问题,并过度嘲讽管理者。尽管有些人认为这是商业决策者必读之书,但另一些人则觉得它乏味且缺乏深度。总体而言,这本书因其在衡量方面的独特视角而受到重视,但其写作风格和呈现方式受到批评。
Similar Books
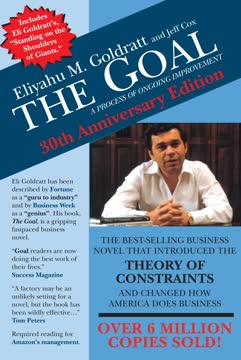
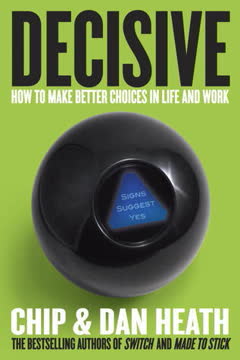
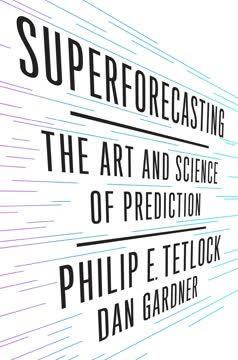
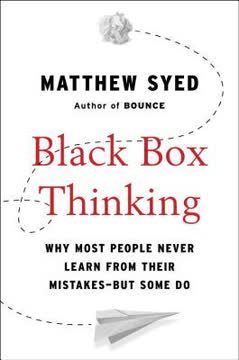
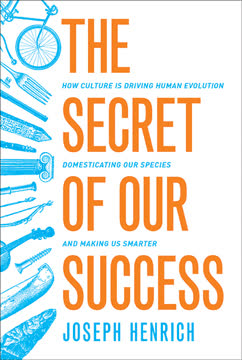
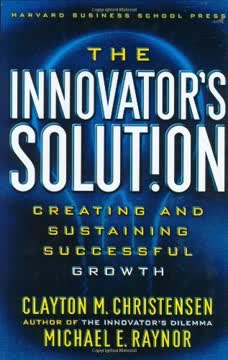
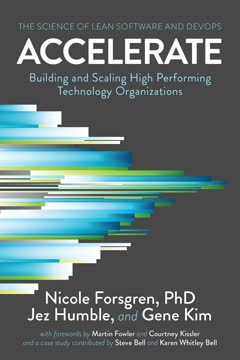
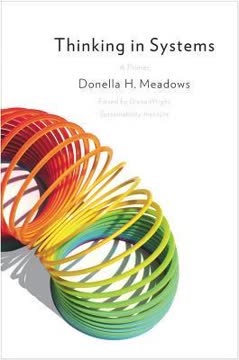
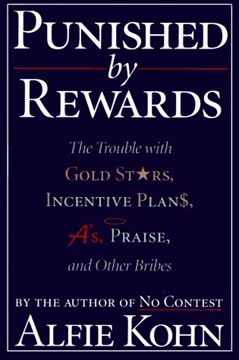
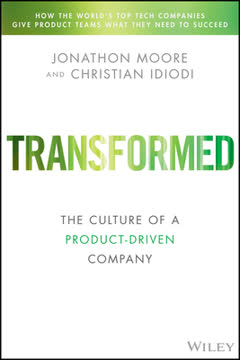