Key Takeaways
1. AI's progress is impressive but still far from human-level intelligence
Easy things are hard.
AI's recent achievements are remarkable, from beating humans at complex games like Go to impressive object recognition in images. However, AI still struggles with many tasks that humans find trivial, like understanding context or applying common sense reasoning. This paradox - AI outperforming humans on some complex tasks while failing at seemingly simple ones - highlights how far we still are from achieving human-level artificial general intelligence (AGI).
The gap between narrow and general AI remains vast. Current AI systems are designed for specific tasks and lack the flexibility and adaptability of human intelligence. While AI can process massive amounts of data and recognize patterns, it doesn't truly understand the meaning or implications of the information it processes. This fundamental lack of understanding limits AI's ability to generalize knowledge or transfer skills between domains.
- Areas where AI excels:
- Complex game playing (chess, Go)
- Image recognition and classification
- Speech recognition and synthesis
Areas where AI struggles:
- Understanding context and nuance
- Applying common sense reasoning
- Generalizing knowledge across domains
2. Deep learning revolutionized AI but has significant limitations
Like supervised-learning systems, these deep Q-learning systems are vulnerable to adversarial examples of the kind I described in chapter 6.
Deep learning breakthrough: The development of deep learning techniques, particularly convolutional neural networks (ConvNets), led to dramatic improvements in AI performance on tasks like image recognition and natural language processing. These systems can learn complex patterns from large datasets, often matching or exceeding human performance on specific benchmarks.
However, deep learning systems have crucial weaknesses. They require enormous amounts of labeled training data, are computationally intensive, and their decision-making processes are often opaque "black boxes." Most critically, these systems are vulnerable to adversarial attacks - carefully crafted inputs designed to fool the AI. For example, minor alterations to an image that are imperceptible to humans can cause an AI to misclassify it entirely.
Limitations of deep learning:
- Reliance on massive labeled datasets
- Lack of true understanding or reasoning
- Vulnerability to adversarial examples
- Difficulty in explaining decision-making processes
- Poor generalization to new scenarios
3. Machine learning requires massive datasets and careful human guidance
Machine learning is still as much an art as a science.
Big data fuels AI: Modern machine learning systems, particularly deep learning models, require enormous amounts of high-quality, labeled data to achieve their impressive performance. Companies like Google, Facebook, and Amazon have a significant advantage in AI development due to their access to vast user-generated datasets.
However, data alone is not enough. Human expertise is crucial in designing AI systems, from choosing the right model architecture to fine-tuning hyperparameters. The process of creating effective AI systems still relies heavily on the intuition and experience of AI researchers and engineers. This "art" of machine learning means that progress in AI is not simply a matter of throwing more data or computing power at problems.
Key aspects of machine learning development:
- Collecting and curating large, high-quality datasets
- Designing appropriate model architectures
- Tuning hyperparameters through trial and error
- Balancing exploration and exploitation in training
- Interpreting results and iterating on the model design
4. AI excels at narrow tasks but lacks general intelligence and common sense
A pile of narrow intelligences will never add up to a general intelligence.
Narrow AI success: AI systems have achieved remarkable results in specific domains, often surpassing human performance. Examples include image recognition, game playing, and certain types of data analysis. These narrow AI systems are increasingly being deployed in real-world applications, from recommendation systems to autonomous vehicles.
However, AI lacks general intelligence. Current AI systems cannot transfer knowledge between domains or apply common sense reasoning to new situations. They struggle with tasks that require understanding context, making analogies, or dealing with ambiguity - all of which are hallmarks of human intelligence. This fundamental limitation means that AI systems often make errors that would be obvious to humans, particularly when faced with scenarios that differ from their training data.
Characteristics of general intelligence AI lacks:
- Transfer learning between domains
- Common sense reasoning
- Understanding context and nuance
- Dealing with ambiguity and uncertainty
- Creativity and abstract thinking
5. Language understanding remains a major challenge for AI systems
Language relies on commonsense knowledge and understanding of the world.
NLP progress: Natural Language Processing (NLP) has seen significant advancements, with AI systems achieving impressive results in tasks like machine translation, speech recognition, and question-answering. These improvements have led to the widespread adoption of virtual assistants and chatbots.
However, true language understanding eludes AI. Current NLP systems rely heavily on statistical patterns in text rather than a deep understanding of meaning. They struggle with context, sarcasm, metaphors, and implicit information - all of which humans navigate effortlessly. This limitation becomes apparent when AI systems make nonsensical errors or fail to grasp the underlying meaning in complex language tasks.
Challenges in AI language understanding:
- Grasping context and subtext
- Interpreting sarcasm and humor
- Understanding metaphors and idioms
- Reasoning about implicit information
- Maintaining coherence in longer conversations
6. AI's brittleness and vulnerability to attacks raise concerns about deployment
When AI can't determine what 'it' refers to in a sentence, it's hard to believe that it will take over the world.
AI vulnerabilities: Despite their impressive performance on benchmarks, AI systems often prove brittle when deployed in real-world scenarios. They can make unexpected errors when faced with inputs that differ slightly from their training data. More alarmingly, researchers have demonstrated that AI systems, particularly in computer vision, can be fooled by carefully crafted adversarial examples.
These vulnerabilities raise serious concerns about AI deployment in critical applications like autonomous vehicles or medical diagnosis. The potential for malicious actors to exploit these weaknesses could have severe consequences. Additionally, the lack of interpretability in many AI systems makes it difficult to predict or explain when and why they might fail.
Types of AI vulnerabilities:
- Sensitivity to small changes in input
- Adversarial attacks (e.g., altered images)
- Bias from training data
- Unexpected failures in edge cases
- Lack of robustness in real-world conditions
7. Ethical considerations are crucial as AI becomes more prevalent in society
Should the industry be aiming for partial autonomy, in which the car does all the driving in "certain circumstances" but the human driver still needs to pay attention and take over if needed? Or should the sole aim be full autonomy, in which the human can completely trust the car's driving and never needs to pay attention?
AI ethics importance: As AI systems become more prevalent in society, ethical considerations surrounding their development and deployment are increasingly crucial. Issues range from privacy concerns and algorithmic bias to the potential for job displacement and the use of AI in warfare.
The AI ethics debate is complex and multifaceted. It involves questions about transparency, accountability, fairness, and the long-term impacts of AI on society. As AI systems take on more critical roles, from healthcare to criminal justice, ensuring they are developed and used ethically becomes paramount. This requires collaboration between technologists, policymakers, ethicists, and the public.
Key ethical considerations in AI:
- Algorithmic bias and fairness
- Privacy and data protection
- Transparency and explainability of AI decisions
- Accountability for AI-caused harm
- Long-term societal impacts of AI deployment
8. True AI progress requires advances in abstraction, analogy, and embodied cognition
I am finding the embodiment argument increasingly compelling.
Cognitive foundations: To achieve genuine progress towards human-level AI, researchers must tackle fundamental aspects of cognition that current systems lack. These include the ability to form abstractions, make analogies, and understand the world through embodied experience.
The embodiment hypothesis suggests that true intelligence requires a physical body interacting with the world. This idea challenges the traditional AI approach of trying to create disembodied intelligence through software alone. Advances in robotics and AI may need to be combined to create systems that can learn and understand the world in ways similar to humans. Additionally, developing AI systems that can form abstractions and make analogies could lead to more flexible and generalizable intelligence.
Key areas for advancing AI cognition:
- Abstraction: Forming general concepts from specific examples
- Analogy: Recognizing similarities between different domains
- Embodied cognition: Learning through physical interaction
- Common sense reasoning: Applying everyday knowledge to new situations
- Causal understanding: Grasping cause-and-effect relationships in the world
Last updated:
FAQ
What's Artificial Intelligence: A Guide for Thinking Humans about?
- Exploration of AI: The book provides a comprehensive overview of artificial intelligence, examining its history, current capabilities, and limitations.
- Human vs. Machine Intelligence: It highlights the differences between human intelligence and AI, emphasizing AI's proficiency in narrow tasks but its lack of general understanding.
- Complexity of Intelligence: The author delves into the complexities of human intelligence, comparing it to AI and raising questions about creativity, emotions, and consciousness.
Why should I read Artificial Intelligence: A Guide for Thinking Humans?
- Informed Perspective: The book offers a balanced view of AI, discussing both its potential benefits and ethical concerns.
- Accessible Language: Written in an engaging manner, it makes complex topics understandable for readers without a technical background.
- Critical Thinking: It encourages readers to think critically about AI's implications in society, prompting discussions about trust, ethics, and future human-AI interactions.
What are the key takeaways of Artificial Intelligence: A Guide for Thinking Humans?
- AI's Limitations: AI has made significant strides but still lacks the general intelligence and common sense that humans possess.
- Human-AI Relationship: The book explores the evolving relationship between humans and AI, emphasizing the need for ethical considerations.
- Future of AI: It discusses the potential for superhuman intelligence and the societal impacts that may arise from AI advancements.
What are the best quotes from Artificial Intelligence: A Guide for Thinking Humans and what do they mean?
- "AI was harder than we thought.": This quote underscores the complexity of replicating human-like intelligence in machines.
- "We tend to overestimate AI advances...": It serves as a caution against the hype surrounding AI, reminding readers of the complexity of human intelligence.
- "Machine stupidity creates a tail risk.": This highlights the potential dangers of relying on AI systems that lack true understanding.
How does Artificial Intelligence: A Guide for Thinking Humans define artificial intelligence?
- Symbolic vs. Subsymbolic AI: The book discusses symbolic AI, which uses human-understandable symbols, and subsymbolic AI, which relies on neural networks.
- Complexity of Intelligence: Intelligence is defined as encompassing various dimensions, including emotional, verbal, and logical intelligence.
- AI's Goals: AI aims to replicate or simulate aspects of human intelligence, but achieving true general intelligence remains a challenge.
What are the main methods of AI discussed in Artificial Intelligence: A Guide for Thinking Humans?
- Deep Learning: This method uses deep neural networks to learn from vast amounts of data, dominating current AI research.
- Reinforcement Learning: Involves training agents to make decisions based on rewards and penalties, allowing them to learn optimal behaviors.
- Monte Carlo Tree Search: Used in game-playing AI, it simulates possible future moves to evaluate the best action.
How does Artificial Intelligence: A Guide for Thinking Humans address the ethical implications of AI?
- Bias in AI Systems: The book discusses how AI can perpetuate societal biases, particularly in applications like facial recognition.
- Need for Regulation: It advocates for thoughtful regulation to ensure AI technologies are developed and used responsibly.
- Moral Machines: Raises questions about whether machines can possess moral reasoning and how to align AI systems' values with human values.
What are the challenges of achieving general AI according to Artificial Intelligence: A Guide for Thinking Humans?
- Complexity of Human Intelligence: Human intelligence involves understanding context, emotions, and social interactions, which AI struggles to replicate.
- Transfer Learning Limitations: Current AI systems cannot easily apply knowledge from one task to another, unlike humans.
- Long-Tail Problem: AI systems often fail in rare or unexpected situations, highlighting the need for robust learning methods.
How does Artificial Intelligence: A Guide for Thinking Humans illustrate the difference between human and machine learning?
- Learning from Few Examples: Humans can learn new concepts from few examples, while AI requires vast amounts of labeled data.
- Exploration vs. Exploitation: Humans naturally balance exploring new options with exploiting known strategies, a balance AI struggles to achieve.
- Common Sense Knowledge: Humans possess a wealth of common sense knowledge that informs decisions, a quality current AI lacks.
What is the significance of reinforcement learning in Artificial Intelligence: A Guide for Thinking Humans?
- Learning from Interaction: Reinforcement learning allows AI systems to make decisions by interacting with their environment.
- Applications in Gaming: Demonstrated by AlphaGo, it shows the potential of reinforcement learning in mastering complex tasks.
- Challenges in Real-World Applications: Transferring reinforcement learning from games to real-world applications remains challenging.
How does Artificial Intelligence: A Guide for Thinking Humans address natural language processing (NLP)?
- Complexity of Language: NLP systems face challenges in understanding human language due to its ambiguity and context-dependence.
- Advancements and Limitations: While deep learning has advanced NLP, these systems often lack true understanding.
- Future Directions: Improvement requires incorporating commonsense knowledge and reasoning abilities for human-like responses.
What role does common sense play in AI according to Artificial Intelligence: A Guide for Thinking Humans?
- Foundation of Understanding: Common sense is critical for human intelligence, which AI currently lacks.
- Challenges in AI Development: Instilling common sense in AI is difficult as much of this knowledge is subconscious.
- Research Efforts: Ongoing efforts aim to develop AI systems with commonsense reasoning capabilities.
Review Summary
Artificial Intelligence: A Guide for Thinking Humans provides a balanced overview of AI's current state and future potential. Readers appreciate Mitchell's clear explanations of complex concepts, her skepticism towards AI hype, and her exploration of AI's limitations. The book covers AI history, recent breakthroughs, and ethical concerns. While some find certain sections challenging, most praise Mitchell's accessible writing style. Some readers note that parts of the book are already outdated due to rapid AI advancements, particularly in language models, but still find value in its foundational content.
Pelican Books Series Series
Similar Books
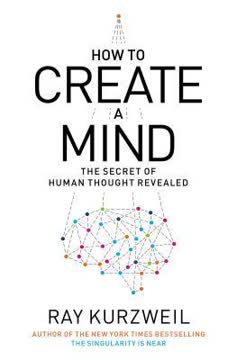
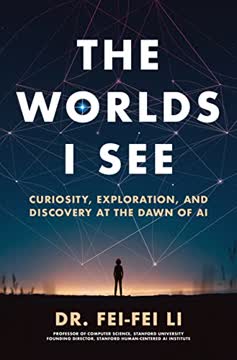
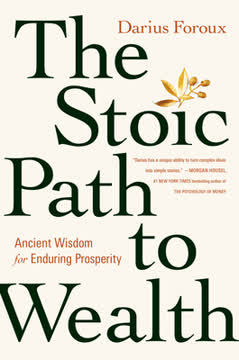
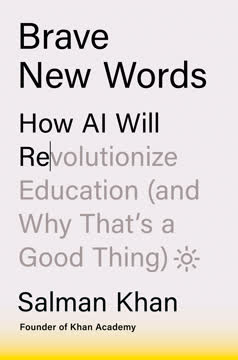
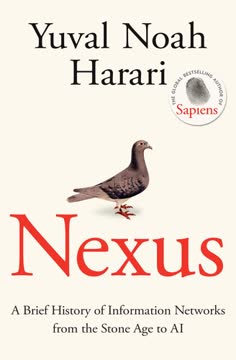
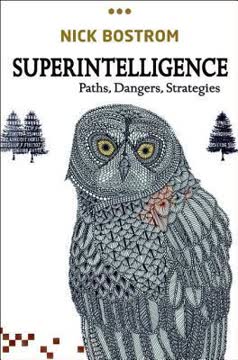
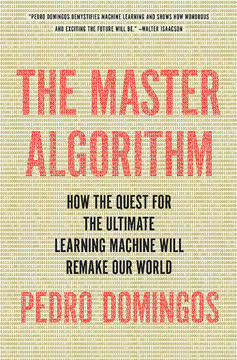
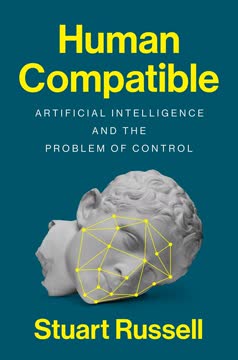
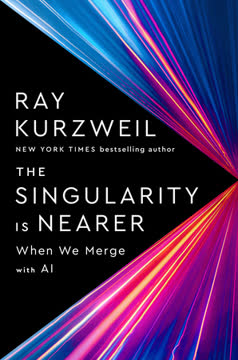
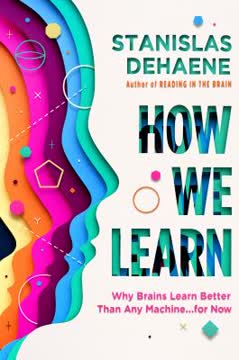
Download PDF
Download EPUB
.epub
digital book format is ideal for reading ebooks on phones, tablets, and e-readers.