Key Takeaways
1. AI is not true intelligence, but a powerful tool for specific tasks
AI can't actually understand anything because it relies on machine processes to manipulate data using pure math in a strictly mechanical fashion.
AI mimics intelligence. Current AI systems excel at specific tasks through pattern recognition and data processing, but lack true understanding or general intelligence. They are incredibly powerful tools for solving well-defined problems, but cannot replicate human-level reasoning or adaptability across diverse situations.
Narrow vs. general AI. Today's AI applications are examples of narrow or weak AI, designed for particular purposes like image recognition or language translation. General AI that matches human-level intelligence across all domains remains a distant goal. Understanding this distinction helps set realistic expectations for AI capabilities and limitations.
Examples of narrow AI:
- Chess engines
- Spam filters
- Voice assistants
- Recommendation systems
2. Data quality and quantity are crucial for effective AI systems
With the present AI solutions, more data equates to more intelligence.
Data fuels AI. The performance of machine learning models depends heavily on the quality and quantity of training data. Large, diverse, and well-curated datasets enable AI systems to recognize patterns and make accurate predictions. However, biased or low-quality data can lead to flawed outputs and reinforce existing inequalities.
Data challenges. Acquiring appropriate data for AI training poses significant challenges. Privacy concerns, data ownership issues, and the need for labeled datasets create barriers to AI development. Organizations must invest in data collection, cleaning, and management processes to build effective AI systems.
Key data considerations for AI:
- Volume: Sufficient quantity for pattern recognition
- Variety: Diverse examples to cover edge cases
- Veracity: Accuracy and reliability of data sources
- Velocity: Ability to process real-time data streams
- Value: Relevance to the problem being solved
3. Machine learning algorithms power modern AI applications
Machine learning works with activities that require intelligence from a human point of view but that aren't easy to formalize as a precise sequence of steps.
Learning from data. Machine learning algorithms enable computers to improve their performance on a task through experience, without being explicitly programmed. This approach has revolutionized AI, allowing systems to tackle complex problems that were previously intractable.
Types of machine learning. Different machine learning paradigms suit various problem types. Supervised learning uses labeled data to train models for classification or prediction tasks. Unsupervised learning discovers patterns in unlabeled data. Reinforcement learning enables agents to learn optimal behaviors through trial and error in interactive environments.
Key machine learning algorithms:
- Linear and logistic regression
- Decision trees and random forests
- Support vector machines
- k-means clustering
- Neural networks
4. Deep learning enables breakthroughs in computer vision and language processing
Deep learning is the technology powering the new wave of AI applications that reaches the news headlines almost every day.
Neural network resurgence. Deep learning, based on artificial neural networks with many layers, has dramatically improved AI performance in areas like image and speech recognition. These models can automatically learn hierarchical features from raw data, reducing the need for manual feature engineering.
Transformative applications. Deep learning has enabled breakthroughs in natural language processing, computer vision, and robotics. Technologies like convolutional neural networks for image analysis and transformer models for language understanding have revolutionized fields from healthcare diagnostics to autonomous vehicles.
Notable deep learning architectures:
- Convolutional Neural Networks (CNNs)
- Recurrent Neural Networks (RNNs)
- Long Short-Term Memory (LSTM) networks
- Transformer models (e.g., BERT, GPT)
5. AI is transforming industries from healthcare to space exploration
AI is great at automation. It never deviates from the procedure, never gets tired, and never makes mistakes as long as the initial procedure is correct.
Healthcare advancements. AI is improving medical diagnosis, drug discovery, and personalized treatment plans. Machine learning models can analyze medical images, predict patient outcomes, and identify potential drug candidates faster and more accurately than traditional methods.
Space exploration. AI enables autonomous spacecraft operations, data analysis from space missions, and planning for future exploration. Machine learning algorithms help process vast amounts of astronomical data, detect exoplanets, and optimize resource utilization in space.
Examples of AI in various industries:
- Finance: Fraud detection, algorithmic trading
- Manufacturing: Predictive maintenance, quality control
- Agriculture: Crop monitoring, precision farming
- Energy: Grid optimization, renewable energy forecasting
- Transportation: Route optimization, traffic management
6. Self-driving cars showcase AI's potential and challenges
Self-driving cars aren't a disruptive invention simply because they'll radically change how people perceive cars, but also because their introduction will have a significant impact on society, economics, and urbanization.
Complex AI systems. Autonomous vehicles integrate multiple AI technologies, including computer vision, sensor fusion, and decision-making algorithms. They represent a real-world test bed for AI's ability to handle complex, dynamic environments with safety-critical requirements.
Technological and societal hurdles. While progress in self-driving technology has been rapid, significant challenges remain. These include improving performance in adverse weather conditions, navigating complex urban environments, and addressing ethical dilemmas in decision-making. Additionally, legal frameworks, insurance models, and public acceptance must evolve to accommodate autonomous vehicles.
Key components of self-driving car AI:
- Perception: Sensors and computer vision
- Localization and mapping
- Path planning and decision making
- Control systems
- V2X communication (vehicle-to-everything)
7. Ethical considerations are vital as AI becomes more prevalent
Ethical considerations of potential uses, both positive and negative, always accompany creating a technology such as AI.
AI ethics frameworks. As AI systems increasingly impact human lives, developing ethical guidelines and governance structures becomes crucial. Key concerns include fairness, accountability, transparency, and privacy. Organizations and governments are working to establish principles for responsible AI development and deployment.
Bias and fairness. AI systems can perpetuate or amplify existing biases present in training data or algorithm design. Ensuring fairness in AI applications, particularly in high-stakes domains like hiring, lending, and criminal justice, is an ongoing challenge requiring interdisciplinary collaboration.
Ethical considerations in AI:
- Algorithmic bias and discrimination
- Privacy and data protection
- Transparency and explainability
- Accountability and liability
- Job displacement and economic impacts
- Autonomous weapons and AI in warfare
8. AI will augment human capabilities rather than replace most jobs
Looking for a future in which AI acts as an assistant to humans. In fact, you'll see this use of AI more and more as time goes on.
Human-AI collaboration. Rather than wholesale job replacement, AI is more likely to augment human capabilities in many fields. This symbiosis can enhance productivity, creativity, and decision-making by combining human intuition and contextual understanding with AI's data processing and pattern recognition abilities.
Evolving job landscape. While AI will automate certain tasks, it will also create new job categories and shift the focus of existing roles. Adaptability and continuous learning will be crucial for workers to thrive in an AI-driven economy. Skills like creativity, emotional intelligence, and complex problem-solving will become increasingly valuable.
Areas where human skills remain crucial:
- Strategic thinking and leadership
- Emotional intelligence and empathy
- Creative and innovative thinking
- Ethical decision-making
- Complex problem-solving in novel situations
9. The future of AI depends on advances in hardware and software
To truly begin to simulate human thought requires specialized hardware, and even the best specialized hardware isn't up to the task today.
Hardware innovations. Advancements in specialized AI hardware, such as GPUs, TPUs, and neuromorphic chips, are crucial for improving AI performance and energy efficiency. These technologies enable faster training of large models and more efficient inference in real-world applications.
Software breakthroughs. Novel algorithms, architectures, and training techniques continue to push the boundaries of AI capabilities. Areas like few-shot learning, transfer learning, and federated learning aim to make AI systems more adaptable and data-efficient.
Emerging AI technologies:
- Quantum computing for AI
- Edge AI and distributed intelligence
- Neuromorphic computing
- Hybrid AI systems (symbolic + neural)
- Explainable AI (XAI) techniques
10. Preparing for an AI-driven future requires understanding its strengths and limitations
Humans will always do certain things better than an AI, and you can be certain that we'll continue to make a place for ourselves in society.
AI literacy. As AI becomes more pervasive, developing a basic understanding of AI concepts, capabilities, and limitations is crucial for individuals and organizations. This knowledge enables informed decision-making about AI adoption and helps set realistic expectations for AI performance.
Lifelong learning. The rapid pace of AI development necessitates a commitment to continuous learning and skill development. Individuals should focus on cultivating uniquely human skills while also gaining familiarity with AI tools and techniques relevant to their fields.
Steps to prepare for an AI-driven future:
- Develop critical thinking and problem-solving skills
- Cultivate creativity and emotional intelligence
- Learn basic programming and data analysis
- Stay informed about AI developments and applications
- Embrace lifelong learning and adaptability
Last updated:
Review Summary
Artificial Intelligence For Dummies receives mixed reviews, with an average rating of 3.5/5. Readers find it a good introduction to AI concepts, covering history, applications, and limitations. Some praise its accessibility and breadth, while others criticize outdated information and repetitiveness. The book is recommended for beginners but may not satisfy those seeking in-depth technical knowledge. Positive aspects include clear explanations and real-world examples, while drawbacks include occasional technical jargon and uneven depth across chapters.
Similar Books
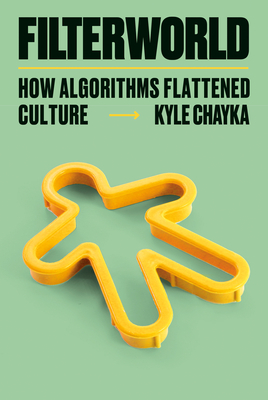







Download PDF
Download EPUB
.epub
digital book format is ideal for reading ebooks on phones, tablets, and e-readers.