Key Takeaways
1. Probability is more than just chance: It's a powerful tool for decision-making
Pascal's rule simply applies the same reasoning down to each individual bet. And in doing so, it gives us a number – the expected value – that allows us to decide whether any bet is worth taking, ahead of time.
Expected value guides decisions. Pascal's rule for calculating expected value provides a framework for making rational decisions under uncertainty. By multiplying the potential consequences of an action by their probability of occurring, we can determine whether a particular course of action is worthwhile.
This concept applies far beyond gambling:
- Insurance decisions: Weighing premiums against potential losses
- Business investments: Evaluating potential returns against risks
- Personal choices: Assessing the value of preventive health measures
Understand the limits. While expected value is a powerful tool, it's important to recognize its limitations:
- It assumes we can accurately estimate probabilities and outcomes
- It doesn't account for individual risk tolerance or psychological factors
- In some cases, like Pascal's Wager about belief in God, the concept can be pushed to extremes
2. The Law of Averages is often misunderstood but crucial for interpreting data
The Law of Averages tells us that when we know – or suspect – we're dealing with events that involve an element of chance, we should focus not on the events themselves, but on their relative frequency – that is, the number of times each event comes up as a proportion of the total number of opportunities to do so.
Focus on relative frequencies. The true Law of Averages doesn't guarantee that things will "even out" in small samples. Instead, it tells us to look at the proportion of outcomes over many trials.
Key points to remember:
- Short-term results can deviate significantly from long-term averages
- The law applies to relative frequencies, not raw numbers
- It requires a large number of independent events to be reliable
Practical applications:
- Gambling: Understanding why casinos always win in the long run
- Scientific studies: Interpreting results from clinical trials
- Market analysis: Evaluating the performance of investment strategies over time
3. Bayes' Theorem: A revolutionary approach to updating beliefs with evidence
New level of belief about something = old level of belief + weight of new evidence
Updating beliefs rationally. Bayes' Theorem provides a mathematical framework for incorporating new evidence into our existing beliefs. This approach is particularly powerful in fields where direct experimentation is difficult or impossible.
Key components of Bayesian reasoning:
- Prior belief: Your initial estimate of probability
- Likelihood: How well the evidence fits different hypotheses
- Posterior belief: Updated probability after considering the evidence
Real-world applications:
- Medical diagnosis: Interpreting test results in light of disease prevalence
- Criminal investigations: Evaluating the strength of forensic evidence
- Spam filters: Continuously improving email classification
Bayesian methods encourage us to:
- Explicitly state our prior beliefs and assumptions
- Quantify the strength of new evidence
- Gradually refine our understanding as more information becomes available
4. Correlation doesn't imply causation: The dangers of misinterpreting data
Quite why it took so long to emerge is an interesting question in the sociology of science, as it was clear from the outset that some researchers were doing extreme violence to several of the 'terms and conditions' of linear regression.
Beware spurious correlations. The ability to find patterns in data is both a strength and a weakness of human cognition. While correlations can provide valuable insights, they can also lead us astray if we're not careful.
Common pitfalls in interpreting correlations:
- Confusing correlation with causation
- Overlooking hidden variables that might explain the relationship
- Failing to consider reverse causality
- Ignoring the possibility of chance correlations in large datasets
Critical thinking is essential. When evaluating claims based on correlations:
- Look for plausible mechanisms that could explain the relationship
- Consider alternative explanations and potential confounding factors
- Examine the strength and consistency of the correlation across different studies
- Be especially skeptical of correlations that confirm pre-existing biases
Remember: The more data we analyze, the more likely we are to find seemingly significant but meaningless correlations by chance alone.
5. The Normal Distribution: Its power and limitations in real-world applications
Pairing off just ten variables against each other while hunting for 'real' correlations carries a 90 per cent risk of finding at least one that's statistically significant purely by chance.
The ubiquitous bell curve. The Normal Distribution is a powerful mathematical tool that accurately describes many natural phenomena. Its prevalence in statistics and data analysis stems from the Central Limit Theorem, which shows that the sum of many independent random variables tends to follow a normal distribution.
Key properties of the Normal Distribution:
- Symmetrical around the mean
- Described by only two parameters: mean and standard deviation
- Approximately 68% of data falls within one standard deviation of the mean
Limitations and dangers. While the Normal Distribution is incredibly useful, assuming normality when it doesn't apply can lead to serious errors:
- Many real-world phenomena have "fat tails" that the normal distribution underestimates
- Financial returns, natural disasters, and other extreme events often follow different distributions
- Assuming normality can lead to underestimating rare but catastrophic risks
When working with data:
- Test for normality before applying methods that assume it
- Be aware of alternative distributions that might better fit your data
- Consider using robust statistical methods that don't rely on normality assumptions
6. Extreme events follow their own rules: The importance of tail risk
Extreme Value Theory (EVT).
Understanding the extraordinary. Extreme Value Theory provides a framework for analyzing and predicting rare events that fall outside the realm of normal experience. This is crucial in fields like finance, engineering, and environmental science, where catastrophic events can have outsized impacts.
Key concepts in EVT:
- The Generalized Extreme Value (GEV) distribution
- Return periods: Estimating how often extreme events are likely to occur
- Threshold exceedances: Focusing on events beyond a certain level of severity
Applications of EVT:
- Financial risk management: Estimating potential losses in market crashes
- Flood protection: Designing structures to withstand rare but severe weather events
- Insurance: Pricing policies for catastrophic risks
Challenges in applying EVT:
- Limited historical data on extreme events
- Difficulty in estimating parameters accurately
- Potential non-stationarity in extreme event frequency due to factors like climate change
7. Financial models are not infallible: The limitations of mathematical approaches to finance
The financial crisis had many causes, political, regulatory and psychological among them. Yet they all have their roots in the same phenomenon: humans trying to deal with uncertainty.
Models are simplifications. Financial models, no matter how sophisticated, are ultimately simplifications of a complex and dynamic reality. They can provide valuable insights, but they should never be treated as infallible predictors of future events.
Limitations of financial models:
- Assumption of normality when real-world distributions have fat tails
- Difficulty in accounting for regime changes and structural shifts
- Inability to capture irrational human behavior and market psychology
- Tendency to underestimate the impact of rare but extreme events
Lessons for investors and policymakers:
- Diversification remains crucial, but be aware that correlations can change in crises
- Stress-test portfolios and strategies against a wide range of scenarios
- Recognize that past performance does not guarantee future results
- Combine quantitative models with qualitative judgment and common sense
Remember: The map is not the territory. Financial models are tools to aid decision-making, not crystal balls that can predict the future with certainty.
Last updated:
FAQ
What's Chancing It: The Laws of Chance and How They Can Work for You about?
- Exploring Probability: The book delves into the principles of probability and their impact on everyday life, decision-making, and scientific research.
- Real-Life Applications: It illustrates how understanding probability can aid in contexts like gambling, insurance, medical diagnoses, and investment strategies.
- Engaging Narrative: Robert Matthews combines personal anecdotes, historical examples, and scientific insights to create an engaging narrative for both specialists and non-specialists.
Why should I read Chancing It by Robert Matthews?
- Practical Decision-Making: The book equips readers with tools to make rational decisions in uncertain situations, relevant in today's complex world.
- Clear Explanations: Matthews explains complex mathematical concepts in an accessible manner, making it suitable for readers without a strong math background.
- Timely Insights: It provides a framework for evaluating claims and making informed decisions based on evidence and probability, crucial in an era of misinformation.
What are the key takeaways of Chancing It?
- Law of Averages: The book clarifies misconceptions about the Law of Averages, emphasizing relative frequencies over raw numbers.
- Bayesian Methods: Matthews highlights the importance of Bayesian probability for updating beliefs based on new evidence.
- Critical Thinking: It encourages questioning common assumptions about chance and risk, promoting a more analytical approach to decision-making.
How does Chancing It explain Bayesian methods?
- Bayesian Probability Defined: Matthews introduces Bayesian methods as a way to update beliefs based on new evidence, contrasting with traditional probability.
- Practical Applications: The book illustrates applications in fields like medicine and finance, showing how Bayesian methods improve decision-making.
- Likelihood Ratio: Emphasizes the importance of the Likelihood Ratio in Bayesian analysis for quantifying evidence strength.
What is the Law of Averages as explained in Chancing It?
- Misconceptions Addressed: Matthews clarifies that the Law of Averages refers to relative frequencies becoming predictable with more trials, not outcomes balancing out.
- Focus on Frequencies: The key takeaway is to concentrate on event frequencies relative to opportunities, essential for interpreting probabilistic scenarios.
- Real-World Examples: Uses experiments like coin tosses to demonstrate how the Law of Averages operates, showing randomness can lead to surprising short-term outcomes.
How does Chancing It critique traditional statistical methods?
- Limitations of Significance Testing: Matthews argues that significance testing often leads to false conclusions and lacks consideration of prior knowledge.
- Frequentist vs. Bayesian: Contrasts frequentist methods with Bayesian approaches, highlighting the latter's incorporation of prior beliefs for more reliable conclusions.
- Call for Change: Advocates for a shift towards Bayesian methods in research and decision-making for more accurate insights.
What are some real-world applications of Bayes's Theorem discussed in Chancing It?
- Medical Diagnoses: Illustrates how Bayes's Theorem improves diagnostic accuracy by updating disease probabilities based on test results.
- Search Operations: Uses the US Coast Guard's search for a missing fisherman to demonstrate decision-making guided by Bayesian updates.
- Cryptography: Discusses Alan Turing's use of Bayes's Theorem in code-breaking during WWII, showcasing its practical significance.
What is the "Problem of Priors" in Bayesian analysis?
- Definition: Refers to the challenge of establishing initial beliefs or probabilities before considering new evidence.
- Impact on Analysis: Without well-defined priors, Bayesian analysis results can be subjective and misleading.
- Addressing the Problem: Discusses strategies like using historical data or expert opinions to inform initial beliefs, enhancing analysis reliability.
What are some common misconceptions about probability discussed in Chancing It?
- Gambler’s Fallacy: The belief that past events influence future outcomes in independent trials is debunked; probabilities remain constant.
- Diagnostic Test Misinterpretation: Many overestimate condition likelihood based on accuracy rates without considering base rates; context is crucial.
- Randomness Equals Patternlessness: Clarifies that randomness can produce patterns over short sequences, misleading individuals into seeing trends.
What are the best quotes from Chancing It and what do they mean?
- Empowerment Through Understanding: "While we can never be free of chance, risk, and uncertainty, they all follow rules which can be turned to our advantage."
- Focus on Frequencies: "When trying to make sense of chance events, ignore the raw numbers. Focus instead on their relative frequency."
- Limitations of Certainty: "The dirty secret lurking in Bernoulli’s Golden Theorem is that when trying to gauge the effects of chance, God-like certainty is unattainable."
How does the author use historical examples to illustrate concepts in Chancing It?
- Alan Turing's Work: Turing's application of Bayes's Theorem in WWII code-breaking highlights its practical significance.
- US Coast Guard Search: Demonstrates the iterative process of updating beliefs with new evidence in real-world scenarios.
- Medical Case Studies: References medical studies to show Bayes's Theorem's impact on improving diagnostic accuracy.
How can I apply the concepts from Chancing It in my daily life?
- Evaluate Risks and Rewards: Use expected value and probability principles to assess decisions, from investments to health choices.
- Question Assumptions: Challenge common beliefs about chance and risk, seeking to understand underlying probabilities for better decision-making.
- Embrace Bayesian Thinking: Apply Bayesian methods to update beliefs with new information, allowing for continuous learning and adaptation.
Review Summary
Chancing it the laws of chance and how they can work for you receives mostly positive reviews, praised for its accessible introduction to statistics and probability. Readers appreciate its clear explanations of complex concepts, historical context, and real-world applications. Some find it challenging or too technical, while others value its insights into statistical pitfalls and Bayesian methods. The book is compared favorably to similar works and recommended for those interested in understanding probability and its implications in various fields, from science to finance.
Similar Books
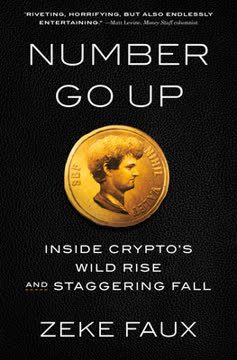
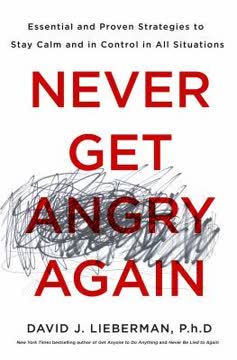
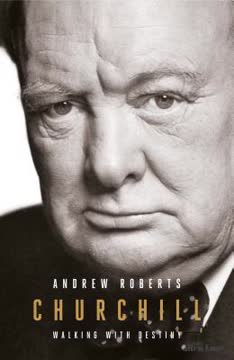
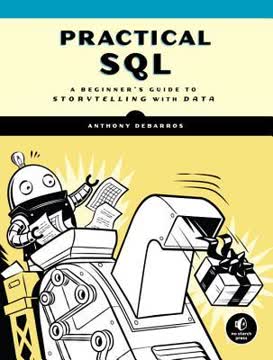
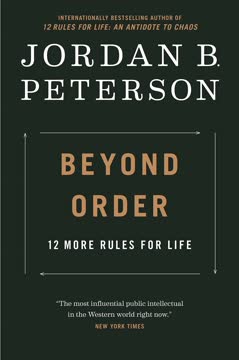
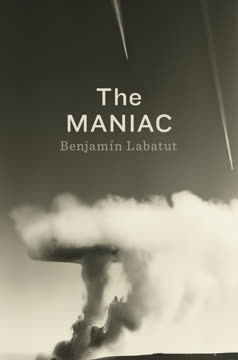
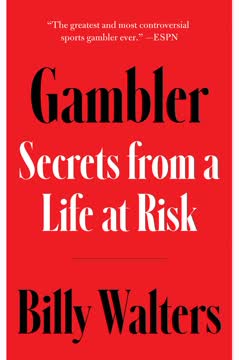
Download PDF
Download EPUB
.epub
digital book format is ideal for reading ebooks on phones, tablets, and e-readers.