Key Takeaways
1. Numbers can mislead: Context is crucial for interpretation
Numbers do not feel. Do not bleed or weep or hope. They do not know bravery or sacrifice. Love and allegiance. At the very apex of callousness, you will find only ones and zeros.
Numbers lack context. Without proper context, numbers can be misleading or meaningless. For example, reporting that 361 cyclists were killed on London roads between 1993 and 2017 sounds alarming. However, this number becomes less significant when you consider that during this period, there were approximately 437,000 daily cycling journeys in London. This context reveals that the risk of death per journey is actually very low.
Denominators matter. To properly interpret numbers, it's crucial to understand the denominator - the total population or base number from which a statistic is derived. For instance, if someone claims that 300 people are murdered by undocumented immigrants in the USA every year, it's essential to know:
- The total number of murders in the USA
- The total population of undocumented immigrants
- The murder rate for the general population
Without this context, it's impossible to determine whether this number is high or low relative to expectations.
2. Sample size matters: Larger samples yield more reliable results
Assuming that the null hypothesis is true and the study is repeated an infinite number times by drawing random samples from the same population(s), less than 5% of these results will be more extreme than the current result.
Bigger is better. Larger sample sizes generally provide more reliable and representative results. Small samples are more susceptible to random variations and outliers, which can lead to misleading conclusions. For example, a study claiming that swearing makes people stronger based on only 29 participants should be viewed with skepticism.
Statistical power. The ability to detect a true effect increases with sample size. This is particularly important when studying subtle effects. As a rule of thumb, be wary of studies with fewer than 100 participants, especially if they make surprising claims. However, keep in mind that:
- Some smaller studies can be robust if well-designed
- Even large studies can be flawed if poorly conducted or biased
- The appropriate sample size depends on the size of the effect being studied and the variability in the population
3. Correlation is not causation: Beware of confounding variables
Let's imagine that you're conducting a study looking at how fast people can run. You notice something: on average, the more grey hairs a person has, the slower their time for the mile.
Hidden factors. Correlation between two variables doesn't necessarily mean one causes the other. There may be hidden factors (confounding variables) influencing both. For example, the correlation between grey hair and slower running speed is likely due to age affecting both variables, rather than grey hair directly causing slower running.
Types of relationships:
- Causal: A directly causes B
- Reverse causality: B actually causes A
- Common cause: C causes both A and B
- Coincidence: No real relationship, just random chance
To establish causation, consider:
- Temporal sequence: The cause must precede the effect
- Strength of association: Stronger correlations are more suggestive of causation
- Dose-response relationship: Changes in the cause lead to proportional changes in the effect
- Consistency: The relationship is observed across different studies and populations
- Plausibility: There's a logical mechanism for the causal relationship
Randomized controlled trials (RCTs) are the gold standard for establishing causation, but they're not always possible or ethical.
4. Statistical significance doesn't equal practical importance
Statistical significance is confusing, even for scientists. A 2002 study found that 100 per cent of psychology undergraduates misunderstand significance – as, even more shockingly, did 90 per cent of their lecturers.
P-value limitations. Statistical significance (typically p < 0.05) only tells us that a result is unlikely to have occurred by chance. It doesn't indicate the size or practical importance of an effect. A tiny, inconsequential difference can be statistically significant with a large enough sample size.
Effect size matters. To understand the practical importance of a finding, we need to consider the effect size:
- How large is the difference between groups?
- What's the magnitude of the correlation?
- Is the effect meaningful in real-world terms?
For example, a study might find a statistically significant link between reading on screens before bed and reduced sleep. However, if the actual effect is only 10 minutes of lost sleep after 4 hours of reading, the practical importance may be minimal for most people.
5. Absolute vs. relative risk: Understand the true impact
If you tell me that eating burnt toast will raise my risk of a hernia by 50 per cent, that sounds worrying. But unless you tell me how common hernias are, it's meaningless.
Relative risk can mislead. Reporting only relative risk changes can exaggerate the importance of findings. A 50% increase in a very rare event is still a very rare event. Always look for the absolute risk to understand the true impact.
Interpreting risk:
- Baseline risk: How common is the outcome initially?
- Relative risk change: The percentage increase or decrease in risk
- Absolute risk change: The actual difference in risk
Example:
- Headline: "Eating bacon daily increases bowel cancer risk by 20%"
- Baseline risk: 6% lifetime risk for women
- Relative risk increase: 20%
- Absolute risk change: 6% to 7.2% (1.2 percentage point increase)
- Interpretation: The risk goes from about 1 in 17 to 1 in 14 - a real increase, but perhaps less dramatic than the headline suggests
6. Survivorship bias: Don't overlook hidden failures
The Navy was looking at a particular subset of planes – those planes which had returned to the carrier. The planes which had been hit a lot on the fuselage and wings tended to have made it back to base successfully. Those that had been hit on the engines, meanwhile, had predominantly fallen into the sea and not been counted in the statistics.
Hidden failures. Survivorship bias occurs when we focus only on successful examples, overlooking those that failed. This can lead to false conclusions about what contributes to success. For example, studying only successful businesses to determine factors for success ignores all the failed businesses that may have had similar characteristics.
Examples of survivorship bias:
- Self-help books by successful entrepreneurs
- Investment strategies based on past performance
- Medical studies that don't account for patients who dropped out
- Historical artifacts that survived due to durability, not representativeness
To avoid survivorship bias:
- Look for the "silent evidence" - what's missing from the data?
- Consider the full population, not just successful examples
- Be skeptical of success stories and "secrets to success"
- Look for studies that account for attrition and non-responders
7. Forecasting limitations: The future is inherently uncertain
As a reader, you need to be aware of how forecasts are made, and you need to know that they are not mystical insights into fate – but nor are they random guesses. They're the outputs of statistical models, which can be more or less accurate; and the very precise numbers (1.2 per cent, 50,000 deaths, whatever) are central estimates inside a much bigger range of uncertainty.
Models have limits. Forecasts are based on models, which are simplified representations of reality. While they can be useful, they're inherently uncertain and based on assumptions that may not hold true. Economic forecasts, election predictions, and climate models all come with significant uncertainty.
Key points about forecasts:
- They're based on past data and current assumptions
- Unexpected events can dramatically alter outcomes
- Longer-term forecasts are generally less reliable
- Point estimates (single numbers) can give a false sense of precision
- Always look for confidence intervals or ranges of possible outcomes
- Consider multiple models and scenarios for a more complete picture
When reporting or interpreting forecasts, it's crucial to communicate the underlying uncertainty and the range of possible outcomes, not just a single point estimate.
8. Goodhart's Law: When a measure becomes a target, it ceases to be a good measure
There's an old saying in economics, Goodhart's law, named for Charles Goodhart, a former economic adviser to the Bank of England: 'When a measure becomes a target, it ceases to be a good measure.'
Perverse incentives. When a metric is used as a target, people will find ways to optimize for that metric, often at the expense of the underlying goal. This can lead to unintended consequences and distorted behavior.
Examples of Goodhart's Law in action:
- Educational targets leading to teaching to the test rather than fostering genuine learning
- Healthcare metrics causing hospitals to refuse high-risk patients to maintain good statistics
- Business KPIs resulting in short-term thinking at the expense of long-term value
- Scientific publishing incentives leading to p-hacking and publication bias
To mitigate Goodhart's Law:
- Use multiple, diverse metrics to assess performance
- Regularly review and update metrics to prevent gaming
- Focus on the underlying goals, not just the numbers
- Be aware of potential unintended consequences
- Use qualitative assessments alongside quantitative metrics
9. Publication bias: Negative results often go unreported
There's a clever way of checking whether there is publication bias in a field, known as a funnel plot. A funnel plot plots the results of all the studies on a topic, with smaller, weaker studies towards the bottom of the chart and larger, better studies towards the top.
Missing evidence. Publication bias occurs when studies with positive or novel results are more likely to be published than those with negative or null results. This can lead to a skewed understanding of the evidence, potentially overestimating the effectiveness of treatments or the strength of relationships.
Consequences of publication bias:
- Overestimation of effect sizes in meta-analyses
- Waste of resources on research that has already been done but not published
- Potential harm to patients if ineffective treatments appear effective
- Difficulty in assessing the true state of knowledge in a field
Methods to detect and mitigate publication bias:
- Funnel plots: Visual tool to detect asymmetry in published results
- Pre-registration of studies: Committing to publish results regardless of outcome
- Registered Reports: Journals agreeing to publish based on methodology, not results
- Databases of unpublished studies
- Encouragement of publishing null results
As a reader or researcher, always consider the possibility of unpublished negative results when evaluating the evidence on a topic.
10. Critical thinking: Develop statistical literacy to navigate a data-driven world
This book can be read as just such a style guide: a sort of AP Style Book for statistical good practice. We hope that media outlets start to follow it, or (equally validly) see the need for one and then write their own. This is not just a book, in fact, but the start of a campaign for statistical literacy and responsibility in the media.
Statistical literacy. In a world increasingly driven by data and statistics, it's crucial to develop critical thinking skills and statistical literacy. This allows us to better understand and evaluate the information we encounter in the media, research, and everyday life.
Key skills for statistical literacy:
- Understanding basic statistical concepts (e.g., averages, variability, probability)
- Recognizing common statistical pitfalls and biases
- Evaluating the quality of data sources and methodologies
- Interpreting graphs and visualizations accurately
- Asking critical questions about claims based on statistics
Actions to promote statistical literacy:
- Encourage media outlets to adopt better practices for reporting statistics
- Support education initiatives that teach statistical thinking
- Be skeptical of sensational headlines based on numbers
- Look for original sources and context when encountering statistics
- Engage in discussions about the proper use and interpretation of data
By developing these skills, we can become more informed citizens, make better decisions, and contribute to a more statistically literate society.
Last updated:
Review Summary
How to Read Numbers is highly praised for its accessible explanation of statistics and data interpretation. Readers appreciate its clarity, real-world examples, and practical advice for critically examining numbers in media. The book covers common statistical pitfalls, biases, and misrepresentations, empowering readers to better understand and question data-driven claims. Many reviewers recommend it as essential reading for journalists and the general public alike, noting its potential to improve statistical literacy and critical thinking skills.
Similar Books
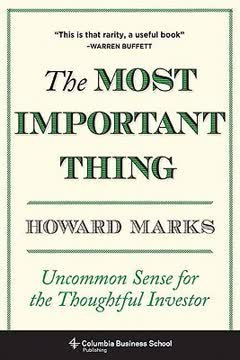
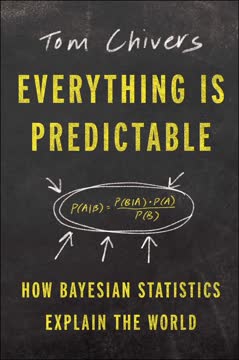
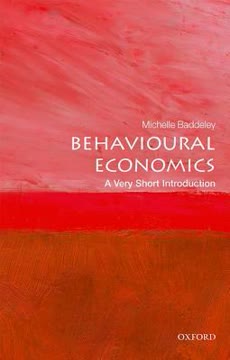
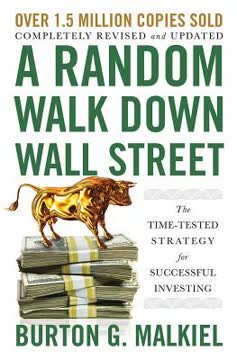

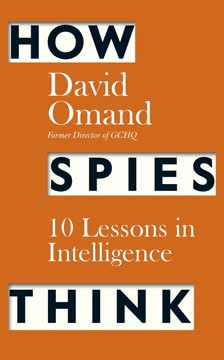
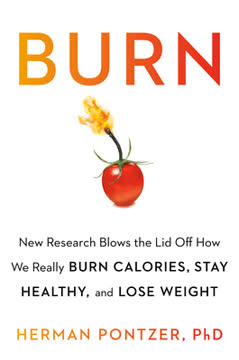
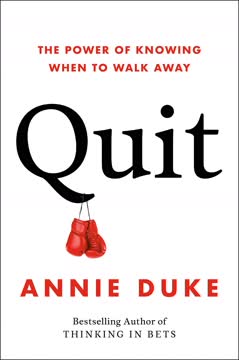
Download PDF
Download EPUB
.epub
digital book format is ideal for reading ebooks on phones, tablets, and e-readers.