Key Takeaways
1. Time series data is ubiquitous and requires specialized analysis techniques
Time series analysis is the endeavor of extracting meaningful summary and statistical information from points arranged in chronological order.
Pervasive data type. Time series data appears in numerous fields, including:
- Medicine: ECG, EEG, patient vitals
- Weather: Temperature, precipitation, air quality
- Economics: Stock prices, GDP, unemployment rates
- Astronomy: Stellar brightness, radio signals
- Internet of Things: Sensor readings, network traffic
Unique challenges. Time series analysis differs from traditional data analysis due to:
- Temporal dependencies between data points
- Presence of trends, seasonality, and cycles
- Need for specialized forecasting techniques
- Importance of maintaining chronological order
2. Proper data preparation is crucial for accurate time series analysis
Cleaning and properly processing data is often the most important step of a timestamp pipeline. Fancy techniques can't fix messy data.
Data cleaning essentials:
- Handling missing values: imputation, interpolation, or deletion
- Addressing outliers and anomalies
- Ensuring consistent time intervals and handling irregular sampling
- Dealing with time zones and daylight saving time changes
Preprocessing techniques:
- Detrending: removing long-term trends
- Differencing: creating stationary series
- Smoothing: reducing noise in the data
- Aggregation: combining data points over specific time periods
Avoiding pitfalls:
- Preventing data leakage from future to past
- Maintaining temporal order during train/test splits
- Properly handling seasonality and cyclical patterns
3. Traditional statistical models provide a solid foundation for time series forecasting
ARIMA models continue to deliver near state-of-the-art performance, particularly in cases of small data sets where more sophisticated machine learning or deep learning models are not at their best.
Key statistical models:
- Autoregressive (AR) models
- Moving Average (MA) models
- Autoregressive Integrated Moving Average (ARIMA) models
- Vector Autoregression (VAR)
- Exponential Smoothing methods
Advantages of statistical models:
- Interpretability: clear understanding of model components
- Well-established theoretical foundations
- Ability to capture linear relationships and seasonality
- Effectiveness with limited data
Limitations:
- Assumption of linear relationships
- Difficulty handling complex, non-linear patterns
- Limited ability to incorporate external variables
4. Machine learning approaches offer new possibilities for complex time series problems
Feature generation is the process of finding a quantitative way to encapsulate the most important traits of time series data into just a few numeric values and categorical labels.
Popular machine learning techniques:
- Random Forests
- Gradient Boosting (XGBoost, LightGBM)
- Support Vector Machines (SVM)
- k-Nearest Neighbors (k-NN)
Advantages of machine learning:
- Ability to capture non-linear relationships
- Handling of high-dimensional data
- Automatic feature importance ranking
- Often outperform traditional models on complex datasets
Considerations:
- Need for careful feature engineering
- Risk of overfitting, especially with limited data
- Importance of cross-validation and regularization
- Balance between model complexity and interpretability
5. Deep learning models show promise but require careful implementation
Deep learning for time series is a relatively new endeavor, but it's a promising one. Because deep learning is a highly flexible technique, it can be advantageous for time series analysis.
Key deep learning architectures:
- Recurrent Neural Networks (RNN)
- Long Short-Term Memory (LSTM)
- Gated Recurrent Units (GRU)
- Convolutional Neural Networks (CNN) for time series
- Transformer models
Advantages of deep learning:
- Ability to automatically learn features from raw data
- Handling of very long sequences
- Capturing complex temporal dependencies
- Potential for transfer learning across similar tasks
Challenges:
- Require large amounts of data for effective training
- Computationally intensive and time-consuming
- Difficulty in interpreting model decisions
- Need for careful hyperparameter tuning
6. Feature engineering and selection are critical for effective time series modeling
The purpose of feature generation is to compress as much information about the full time series as possible into a few metrics or, alternately, to use those metrics to identify the most important information about the time series and discard the rest.
Common time series features:
- Statistical measures: mean, variance, skewness, kurtosis
- Trend indicators: slope, intercept of linear fit
- Seasonal components: Fourier terms, seasonal dummies
- Autocorrelation and partial autocorrelation coefficients
- Spectral features: dominant frequencies, power spectral density
Feature selection techniques:
- Correlation-based methods
- Mutual information
- Recursive feature elimination
- Lasso and Ridge regression
- Tree-based feature importance
Importance of domain knowledge:
- Incorporating field-specific indicators
- Understanding relevance of different time scales
- Identifying meaningful patterns and anomalies
7. Evaluating time series models demands rigorous and time-aware methodologies
The most important element of generating a forecast is to make sure that you are building it solely with data you could access sufficiently in advance for that data to be used in generating the forecast.
Key evaluation metrics:
- Mean Absolute Error (MAE)
- Mean Squared Error (MSE)
- Root Mean Squared Error (RMSE)
- Mean Absolute Percentage Error (MAPE)
- Symmetric Mean Absolute Percentage Error (SMAPE)
Time-aware evaluation strategies:
- Rolling window validation
- Temporal cross-validation
- Backtesting on historical data
Considerations for model comparison:
- Accounting for different forecast horizons
- Assessing performance across multiple time series
- Evaluating uncertainty and confidence intervals
- Comparing against simple baseline models (e.g., naive forecast)
8. Performance optimization is essential for large-scale time series applications
Time series data sets get so large that analyses can't be done at all—or can't be done properly—because they are too intensive in their demands on available computing resources.
Optimization strategies:
- Data downsampling and aggregation
- Efficient data storage formats (e.g., Apache Parquet)
- Parallelization of computations
- GPU acceleration for deep learning models
- Incremental learning for streaming data
Balancing accuracy and speed:
- Trade-offs between model complexity and computational requirements
- Identifying bottlenecks in the analysis pipeline
- Caching intermediate results for faster recomputation
- Using approximate algorithms for large-scale problems
Considerations for production deployment:
- Scalability of the chosen modeling approach
- Real-time prediction requirements
- Resource constraints of the deployment environment
- Monitoring and updating models over time
Last updated:
FAQ
1. What is Practical Time Series Analysis: Prediction with Statistics and Machine Learning by Aileen Nielsen about?
- Comprehensive time series guide: The book provides a thorough overview of time series analysis, covering the entire workflow from data collection and preprocessing to modeling and forecasting.
- Bridges traditional and modern methods: It uniquely combines classical statistical models (like ARIMA) with modern machine learning and deep learning techniques, offering practical advice and code examples.
- Real-world applications: Case studies span domains such as healthcare, finance, and government, demonstrating the versatility of time series methods.
- Focus on practical implementation: The author emphasizes hands-on coding, performance considerations, and common pitfalls, making it a valuable resource for practitioners.
2. Why should I read Practical Time Series Analysis by Aileen Nielsen?
- Fills a knowledge gap: Time series analysis is often underrepresented in data science resources; this book provides a centralized, accessible guide.
- Balanced theory and practice: It offers both mathematical foundations and practical coding examples, suitable for readers who want to understand both the "why" and "how."
- Addresses real-world challenges: The book discusses issues like data leakage, lookahead bias, and causality, helping readers avoid common mistakes.
- Covers modern techniques: It introduces deep learning models and advanced machine learning methods, which are often missing from traditional time series texts.
3. What are the key takeaways from Practical Time Series Analysis by Aileen Nielsen?
- End-to-end workflow: The book covers the full pipeline, from data handling and preprocessing to modeling, evaluation, and deployment.
- Model diversity: Readers learn about statistical models, machine learning, and deep learning approaches, understanding their strengths and limitations.
- Feature engineering importance: The book highlights the critical role of domain-informed feature generation and selection in time series modeling.
- Practical pitfalls and solutions: It provides actionable advice on avoiding lookahead bias, handling missing data, and optimizing performance for real-world applications.
4. What background knowledge is recommended for reading Practical Time Series Analysis by Aileen Nielsen?
- Coding familiarity: Some experience with R and Python, especially libraries like NumPy, Pandas, scikit-learn, and data.table, is helpful.
- Statistics and ML basics: Understanding of variance, correlation, probability distributions, clustering, decision trees, and neural networks is recommended.
- Supplementary resources: The book offers brief overviews and links to free online tutorials for readers needing to strengthen foundational knowledge.
- No advanced prerequisites: While some background is useful, the book is designed to be accessible to data scientists and supervisors alike.
5. How does Practical Time Series Analysis by Aileen Nielsen define and explain time series data?
- Chronological data focus: Time series analysis is about extracting meaningful information from data points arranged in chronological order.
- Broad interpretation of time: The book notes that time series methods apply to any ordered data, not just temporal, such as frequency-ordered spectrographs.
- Causality and trends: Time series uniquely address questions of causality, trends, and future event likelihood, which cross-sectional data cannot.
- Importance in forecasting: The book emphasizes the role of time series in diagnosing past behavior and predicting future outcomes.
6. What are the main statistical models for time series covered in Practical Time Series Analysis by Aileen Nielsen?
- Autoregressive (AR) models: These predict future values based on past observations, with detailed explanations of AR(1) and AR(p) processes.
- Moving Average (MA) models: MA models use past error terms to model current values, with guidance on parameter selection and limitations.
- ARIMA and extensions: The book explains ARIMA models, which combine AR and MA components with differencing to handle nonstationarity, and discusses automated fitting methods.
- State space models: It covers Kalman filters, Hidden Markov Models (HMMs), and Bayesian Structural Time Series (BSTS) for modeling hidden states and system dynamics.
7. How does Practical Time Series Analysis by Aileen Nielsen explain stationarity and its importance?
- Stationarity defined: A stationary time series has stable statistical properties (mean, variance) over time; nonstationary series exhibit trends or changing variance.
- Testing for stationarity: The book introduces the Augmented Dickey–Fuller (ADF) test and explains mathematical conditions for stationarity in AR models.
- Modeling implications: Many time series models require stationarity; the book discusses transformations like differencing and log transforms to achieve it.
- Weak vs. strong stationarity: It clarifies the difference, with weak stationarity requiring constant mean and variance, and strong stationarity demanding the entire distribution remains unchanged.
8. What are the key machine learning and deep learning methods for time series in Practical Time Series Analysis by Aileen Nielsen?
- Tree-based models: Random forests and gradient boosted trees (e.g., XGBoost) are used for classification and forecasting, with practical examples.
- Clustering and distance metrics: The book covers clustering with feature-based and raw time series distances, introducing dynamic time warping (DTW) and alternatives to Euclidean distance.
- Deep learning architectures: It discusses feedforward networks, convolutional neural networks (CNNs), recurrent neural networks (RNNs), and attention mechanisms, explaining their suitability for temporal data.
- Hybrid and advanced models: The book explores hybrid models like LSTNet, combining convolutional, recurrent, and autoregressive components for complex patterns.
9. How does Practical Time Series Analysis by Aileen Nielsen approach feature generation and selection for time series?
- Domain knowledge emphasis: The book stresses the importance of features informed by domain expertise, such as time-of-day or seasonal effects.
- Automated feature extraction: Tools like tsfresh, Cesium, and tsfeatures are reviewed for generating thousands of statistical and periodicity features.
- Time-aware features: Lagged values, rolling statistics, and categorical time indicators are recommended to capture temporal dynamics.
- Feature selection techniques: Methods like recursive feature elimination and FRESH are discussed to identify the most predictive features and avoid overfitting.
10. What are the main challenges and solutions for handling missing data, lookahead bias, and model validation in Practical Time Series Analysis by Aileen Nielsen?
- Missing data strategies: The book covers imputation methods like forward fill, moving averages, and interpolation, with cautions about their pitfalls.
- Lookahead bias warning: It emphasizes avoiding future information leakage into training or evaluation, which can invalidate models.
- Time-aware validation: Rolling window backtesting is recommended to mimic real forecasting scenarios, rather than random splits that violate temporal order.
- Model-specific considerations: The book notes that even models trained chronologically can suffer from data leakage if not carefully managed.
11. How does Practical Time Series Analysis by Aileen Nielsen address performance optimization and practical implementation?
- Data overlap inefficiency: The book discusses how overlapping time windows can inflate dataset size and suggests non-overlapping windows or generator-based feeding.
- Precomputation for speed: Precomputing parts of models, such as unrolling RNNs for known past data, can reduce prediction latency in real-time applications.
- Efficient data storage: Using binary formats (e.g., pickling in Python) improves I/O speed and reduces storage size compared to CSVs.
- Production considerations: The book prepares readers for real-world challenges, including performance bottlenecks and deployment issues.
12. What tools, packages, and resources does Practical Time Series Analysis by Aileen Nielsen recommend for time series forecasting and anomaly detection?
- Automated forecasting frameworks: Google’s in-house system and Facebook’s Prophet package are highlighted for handling seasonality, holidays, and level changes.
- Anomaly detection tools: Twitter’s AnomalyDetection package is recommended for identifying outliers in seasonal time series.
- Feature extraction and modeling: The book points to R and Python packages like tsfeatures, tsfresh, and bsts for feature extraction and state space modeling.
- Encouragement to explore: Readers are encouraged to leverage the extensive R and Python ecosystems for their time series projects.
Review Summary
Practical Time Series Analysis receives mixed reviews. Some readers find it a good introductory book covering basics and best practices, while others criticize it for lacking practical examples and containing errors. Positive aspects include its overview of techniques, data sources, and references. Critics note poor modeling results and undigested material. Some readers suggest it's better as a reference companion to hands-on practice. The book's mathematical explanations are considered weak, with readers recommending online alternatives for deeper understanding. Overall, it's seen as a broad overview rather than a practical guide.
Similar Books
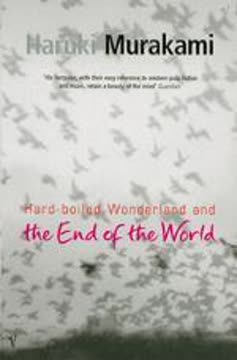
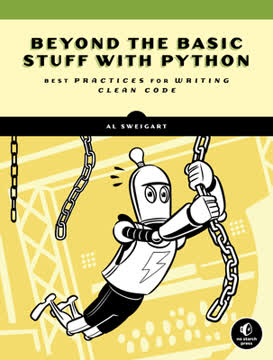
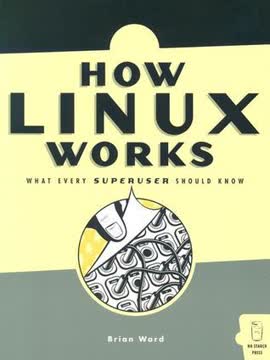
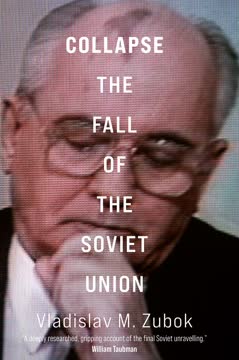
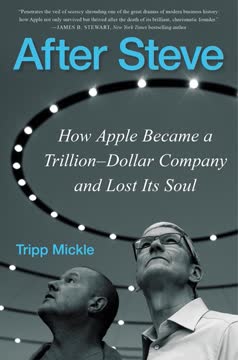
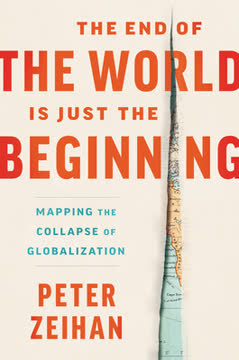
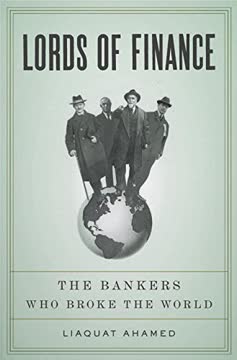
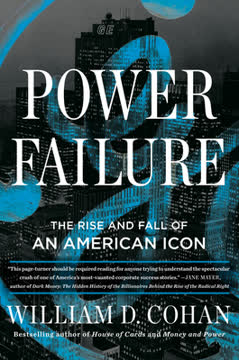
Download PDF
Download EPUB
.epub
digital book format is ideal for reading ebooks on phones, tablets, and e-readers.