Key Takeaways
1. Statistics permeate everyday life, influencing decisions and perceptions
"Statistics is really the business of using the scientific method to answer research questions about the world."
Ubiquitous influence. Statistics shape our understanding of the world, from news headlines to product claims. They inform policy decisions, medical treatments, and consumer choices. However, not all statistics are created equal. Many are misused, misinterpreted, or simply incorrect.
Critical evaluation. To navigate this statistical landscape, it's essential to develop a critical eye. Question the source of statistics, understand how they were collected, and consider potential biases. Look for key elements like sample size, margin of error, and methodology. Remember that a single statistic rarely tells the whole story.
Real-world applications. Statistics play a role in:
- Weather forecasts and climate predictions
- Economic indicators and financial markets
- Medical research and treatment efficacy
- Political polling and election forecasts
- Quality control in manufacturing
- Sports analytics and performance metrics
2. Understanding data displays is crucial for accurate interpretation
"Data displays, such as charts and graphs, appear often in everyday life."
Visual representation. Charts, graphs, and tables condense large amounts of data into digestible formats. However, they can also be manipulated to mislead. Understanding common types of data displays is essential for accurate interpretation.
Key types of displays:
- Bar graphs: Compare quantities across categories
- Pie charts: Show parts of a whole
- Line graphs: Display trends over time
- Scatter plots: Illustrate relationships between variables
- Histograms: Show distribution of numerical data
Critical analysis. When examining data displays:
- Check the scale on both axes
- Look for missing or truncated information
- Be wary of 3D effects that can distort proportions
- Consider whether the chosen display type is appropriate for the data
- Verify that percentages in pie charts sum to 100%
3. Measures of central tendency and variability reveal data's true nature
"The standard deviation is an important statistic, but it is often absent when statistical results are reported."
Central tendency. Measures like mean, median, and mode provide a central value around which data clusters. Each has strengths and weaknesses:
- Mean: Sensitive to outliers, best for symmetric distributions
- Median: Resistant to outliers, ideal for skewed data
- Mode: Useful for categorical data or multimodal distributions
Variability. Measures of spread complement central tendency:
- Range: Simplest measure, but sensitive to outliers
- Standard deviation: Most common, indicates typical distance from the mean
- Interquartile range: Robust measure, focuses on middle 50% of data
Comprehensive picture. Always consider both central tendency and variability when interpreting data. A dataset with the same mean can have vastly different distributions. Standard deviation provides context for how tightly clustered or widely dispersed the data is around the central value.
4. Probability concepts guide decision-making under uncertainty
"The laws of probability often go against your intuition and your own beliefs about what you think can happen (that's why casinos stay in business)."
Foundations of uncertainty. Probability quantifies the likelihood of events occurring. It underpins statistical inference, risk assessment, and many real-world decisions. Key concepts include:
- Sample space: All possible outcomes
- Events: Subsets of the sample space
- Conditional probability: Likelihood given prior information
- Independence: When events don't influence each other
Common misconceptions:
- Gambler's fallacy: Believing past outcomes influence future independent events
- Base rate neglect: Ignoring prior probabilities when assessing likelihood
- Conjunction fallacy: Assuming specific conditions are more probable than general ones
Practical applications. Probability informs:
- Insurance risk assessment
- Medical diagnosis and treatment decisions
- Weather forecasting
- Quality control in manufacturing
- Financial modeling and investment strategies
5. Sampling techniques and margin of error determine result reliability
"The margin of error is the only way to measure how close the sample information is to the actual population you're interested in."
Sampling fundamentals. Proper sampling is crucial for drawing valid conclusions about a population. Key considerations:
- Random sampling: Ensures each member has an equal chance of selection
- Sample size: Larger samples generally yield more precise estimates
- Stratified sampling: Divides population into subgroups for better representation
- Cluster sampling: Selects groups rather than individuals
Margin of error. This measure indicates the range within which the true population parameter likely falls. Factors affecting margin of error:
- Sample size: Larger samples reduce margin of error
- Confidence level: Higher confidence widens the margin
- Population variability: More diverse populations increase uncertainty
Interpreting results. Always consider the margin of error when evaluating statistics, especially in polls and surveys. A "lead" within the margin of error may not be statistically significant. Be wary of results presented without this crucial context.
6. Hypothesis testing enables evidence-based conclusions
"Hypothesis tests are similar to jury trials, in a sense. In a jury trial, Ho is similar to the not-guilty verdict, and Ha is the guilty verdict."
Framework for inference. Hypothesis testing provides a structured approach to evaluate claims about populations based on sample data. Key components:
- Null hypothesis (H0): Assumption of no effect or difference
- Alternative hypothesis (Ha): Claim being investigated
- Test statistic: Measure of evidence against H0
- p-value: Probability of obtaining results as extreme as observed, assuming H0 is true
Decision process:
- Set significance level (α) before conducting the test
- Calculate test statistic and p-value
- If p-value < α, reject H0; otherwise, fail to reject H0
Interpretation caveats:
- Failing to reject H0 doesn't prove it true
- Statistical significance doesn't always imply practical importance
- Multiple testing increases the risk of false positives
7. Correlation and causation are distinct but often confused concepts
"Of all of the misunderstood statistical issues, the most problematic is the misuse of the concepts of correlation and causation."
Correlation basics. Correlation measures the strength and direction of a linear relationship between two variables. Key points:
- Ranges from -1 to +1
- Positive correlation: Variables move in same direction
- Negative correlation: Variables move in opposite directions
- Correlation of 0 indicates no linear relationship
Causation complexities. Establishing causation requires more than correlation:
- Temporal precedence: Cause must precede effect
- Non-spuriousness: Relationship not explained by third variable
- Mechanism: Plausible explanation for how cause produces effect
Common pitfalls:
- Assuming correlation implies causation
- Reverse causation: Mistaking effect for cause
- Confounding variables: Overlooking factors influencing both variables
Critical thinking. When presented with correlational claims, consider alternative explanations and look for supporting experimental evidence before inferring causation.
8. Well-designed experiments are key to establishing causal relationships
"Even if you never conduct a hypothesis test of your own, just knowing how they are supposed to be done can sharpen your critiquing skills."
Experimental design principles:
- Random assignment: Distribute participants to groups by chance
- Control group: Provides baseline for comparison
- Blinding: Participants and/or researchers unaware of group assignments
- Replication: Repeat experiments to verify results
- Large sample sizes: Increase statistical power and reliability
Control for confounding:
- Randomization: Balances unknown factors across groups
- Blocking: Groups similar subjects to reduce variability
- Matched pairs: Compare similar individuals across treatments
Evaluating experiments:
- Check for proper controls and randomization
- Assess sample size and representativeness
- Look for potential sources of bias
- Consider ethical implications
Limitations. Not all questions can be answered experimentally due to ethical or practical constraints. In these cases, well-designed observational studies may be the best available evidence.
9. Surveys and polls require careful design and interpretation
"Not all surveys are as accurate and reliable as some may want you to think."
Survey design considerations:
- Clear, unbiased questions
- Appropriate sampling method
- Adequate sample size
- Minimize non-response bias
- Pilot testing to identify issues
Common pitfalls:
- Leading questions that bias responses
- Convenience sampling that's not representative
- Low response rates skewing results
- Reporting margin of error for non-probability samples
Interpreting poll results:
- Consider the source and potential biases
- Check sample size and methodology
- Look for trends across multiple polls
- Be wary of outliers or surprising results
Critical evaluation. When encountering survey results:
- Who conducted the survey and why?
- How were participants selected?
- What was the exact wording of questions?
- What was the response rate?
- Are results consistent with other reputable surveys?
10. Statistical literacy empowers critical thinking and informed choices
"The purpose of this book is to get you ready to sort through and evaluate the incredible amount of statistical information that comes to you on a daily basis."
Everyday relevance. Statistical literacy is crucial for:
- Evaluating news and media claims
- Making informed personal and financial decisions
- Understanding scientific and medical research
- Critically assessing marketing and advertising
- Participating in informed civic discourse
Key skills:
- Recognizing potential sources of bias
- Understanding margins of error and confidence intervals
- Distinguishing between correlation and causation
- Identifying misleading graphs and data presentations
- Asking critical questions about methodology and sample selection
Ongoing development. Statistical literacy is a lifelong process. Stay curious, question claims, and seek out reliable sources of information. Recognize that statistics are tools for understanding the world, but they require careful interpretation and context.
Empowerment through knowledge. By developing statistical literacy, you become a more discerning consumer of information, better equipped to make evidence-based decisions and contribute to meaningful discussions on complex issues facing society.
Last updated:
FAQ
What's Statistics for Dummies about?
- Comprehensive Guide: Statistics for Dummies by Deborah J. Rumsey is an accessible introduction to statistical concepts and methods, covering everything from basic definitions to complex topics like hypothesis testing.
- Practical Applications: The book emphasizes real-world applications, making statistics relevant for understanding everyday life, with examples from business, healthcare, and social sciences.
- User-Friendly Format: Structured to break down complex ideas into digestible parts, it ensures a smooth learning curve for beginners, with each chapter building on the previous one.
Why should I read Statistics for Dummies?
- Foundation in Statistics: Ideal for building a solid foundation in statistics, whether for academic purposes or personal interest, demystifying statistical jargon and concepts.
- Enhance Critical Thinking: Understanding statistics improves critical thinking skills, enabling data analysis and informed decision-making in a data-driven world.
- Practical Examples: Includes practical examples and exercises that reinforce learning, allowing readers to apply concepts to real-life situations.
What are the key takeaways of Statistics for Dummies?
- Understanding Variability: Discusses the variability of sample results and its impact on statistical conclusions, emphasizing the central limit theorem.
- Margin of Error: Highlights the significance of the margin of error in surveys and polls, teaching readers how to calculate and interpret it.
- Hypothesis Testing: Provides a framework for conducting hypothesis tests, including setting up null and alternative hypotheses and interpreting p-values.
How does Statistics for Dummies explain the concept of probability?
- Basic Definition: Probability is defined as the likelihood of an event occurring, expressed as a number between 0 and 1.
- Rules of Probability: Outlines fundamental rules, such as the sum of probabilities for all possible outcomes equaling 1.
- Real-Life Applications: Provides examples of how probability is used in everyday situations, such as weather forecasting and gambling.
What is the margin of error, as described in Statistics for Dummies?
- Definition: The margin of error measures how much a sample result is expected to differ from the actual population parameter.
- Importance in Surveys: Contextualizes survey results, indicating the range within which the true population value likely falls.
- Calculation Steps: Explains how to calculate it using sample proportion, sample size, and Z-value for confidence levels.
What is hypothesis testing in Statistics for Dummies?
- Purpose: Hypothesis testing is used to make decisions about population parameters based on sample data, determining evidence against a null hypothesis.
- Setting Up Hypotheses: Explains how to set up null (Ho) and alternative (Ha) hypotheses, such as testing average times or proportions.
- Interpreting Results: Teaches calculation of test statistics and p-values, with a small p-value indicating strong evidence against the null hypothesis.
Can you explain the difference between correlation and causation as per Statistics for Dummies?
- Correlation Defined: Indicates a relationship between two variables, where changes in one are associated with changes in another.
- Causation Explained: Implies one variable directly affects another, requiring rigorous testing to establish.
- Common Misunderstandings: Warns against assuming causation from correlation alone, highlighting the need for careful analysis and experimentation.
What are some common statistical mistakes mentioned in Statistics for Dummies?
- Biased Data: Highlights that biased data can lead to incorrect conclusions, stressing the need for random sampling in surveys.
- Misleading Graphs: Warns about graphs that distort data representation, advising checks for proper scales and accurate percentages.
- Overstating Results: Encourages critical evaluation of claims and looking for margins of error to understand the reliability of findings.
How does Statistics for Dummies address the importance of sample size?
- Impact on Accuracy: Larger sample sizes generally lead to more accurate results, with a rough formula for estimating margin of error.
- Determining Adequate Size: Discusses how to determine an adequate sample size, recommending at least 30 for statistical validity.
- Diminishing Returns: Notes that increasing sample size yields diminishing returns in accuracy, balancing cost and effort with desired precision.
What is the role of statistical process control (SPC) in quality management as described in Statistics for Dummies?
- Definition of SPC: A method to monitor and control a process through statistical analysis, ensuring consistency within specified limits.
- Control Charts: Used to visualize process data over time, identifying when a process is out of control and requires intervention.
- Quality Improvement: Integral to total quality management (TQM), allowing continuous process improvement, leading to higher customer satisfaction and reduced costs.
What are the measures of relative standing discussed in Statistics for Dummies?
- Percentiles: Explained as a way to understand an individual's position relative to others, indicating the percentage of scores below a given score.
- Standard Scores: Introduces standard scores (Z-scores) to compare scores from different distributions, expressed in terms of standard deviations from the mean.
- Interpreting Results: Emphasizes the importance of these measures in making sense of statistical results and understanding individual standings.
What are the best quotes from Statistics for Dummies and what do they mean?
- "Statistics means never having to say you're certain!": Highlights that results are probabilistic rather than definitive, encouraging a critical mindset.
- "Garbage in equals garbage out.": Emphasizes the importance of data quality, as flawed data leads to unreliable conclusions.
- "You can’t measure bias.": Cautions that while variability and error can be quantified, bias cannot, urging vigilance about potential biases.
Review Summary
Statistics for Dummies receives mostly positive reviews, with readers praising its accessibility for beginners and its ability to simplify complex statistical concepts. Many find it helpful for students and those looking to refresh their knowledge. The book's practical examples and hands-on approach are appreciated. Some readers note that it can be repetitive if read cover-to-cover, and a few mention it lacks depth for advanced topics. Overall, it's recommended as a solid introduction to statistics for those with little to no prior experience.
Similar Books
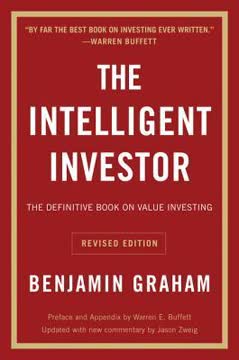
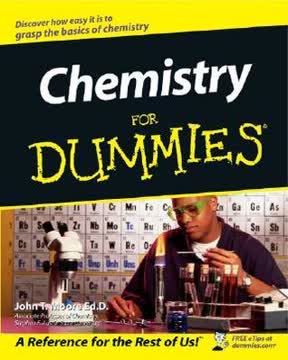
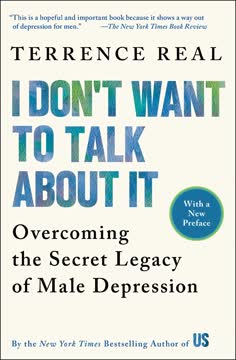
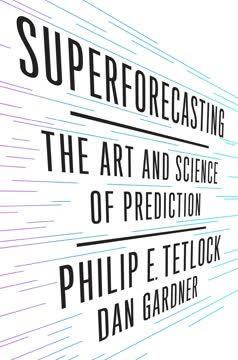
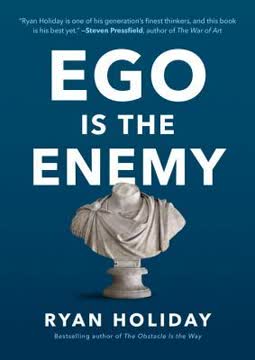
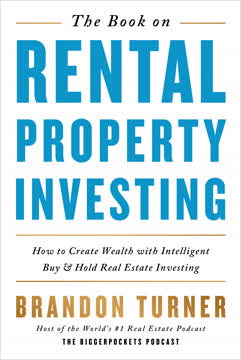
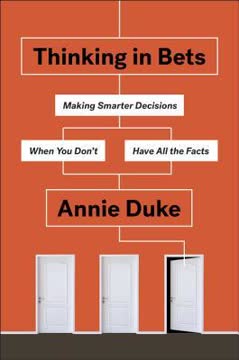
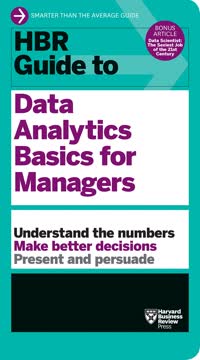
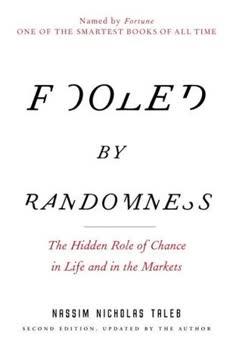
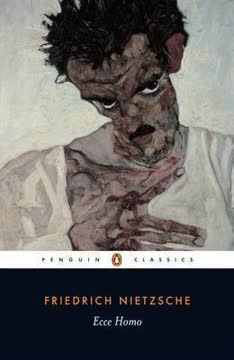
Download PDF
Download EPUB
.epub
digital book format is ideal for reading ebooks on phones, tablets, and e-readers.