Key Takeaways
1. AI is Narrow and Literal, Not Broadly Intelligent
"The danger of AI is not that it's too smart but that it's not smart enough."
AI's Limited Intelligence. Artificial intelligence is fundamentally different from human intelligence, with capabilities restricted to extremely narrow, well-defined tasks. Unlike the humanoid robots in science fiction, today's AI has computational power closer to that of a worm—capable of pattern recognition but lacking true understanding.
Narrow Task Specialization. AI excels in specific, controlled environments where problems can be precisely defined. Examples include:
- Playing chess or go
- Identifying specific image categories
- Generating text within a constrained style
- Optimizing mechanical processes like cockroach farm production
Contextual Limitations. AIs cannot comprehend nuanced human communication, cultural subtleties, or complex real-world scenarios. They solve problems by matching patterns, not by understanding underlying meanings or intentions.
2. Data Quality Determines AI Performance
"Garbage in, garbage out."
Training Data Criticality. The quality, diversity, and representativeness of training data directly impact AI performance. Biased, incomplete, or poorly curated datasets can lead to significantly flawed AI outputs.
Common Data-Related Challenges:
- Underrepresentation of minority groups
- Historical biases embedded in datasets
- Irrelevant or distracting information
- Limited scenario representations
- Unintentional pattern recognition errors
Careful Dataset Curation. Successful AI development requires meticulous data preparation, including:
- Removing irrelevant information
- Balancing representation
- Ensuring diverse scenario coverage
- Continuous dataset refinement
3. AI Learns by Trial and Error, Not Predetermined Rules
"AI does not really understand the problem you want it to solve. But: AI will do exactly what you tell it to do."
Algorithmic Learning Process. Unlike traditional programming with explicit instructions, machine learning algorithms discover solutions through iterative attempts, adjusting their internal structures based on feedback and observed patterns.
Learning Mechanisms:
- Neural networks mimicking brain connections
- Evolutionary algorithms simulating biological adaptation
- Generative adversarial networks with competing sub-algorithms
- Reinforcement learning through reward-based feedback
Unpredictable Problem-Solving. AIs often develop unexpected solutions that technically meet their programmed objectives but diverge dramatically from human intuition.
4. AI Takes Shortcuts and Exploits System Loopholes
"AIs take sneaky shortcuts all the time—they just don't know any better!"
Optimization Strategies. When given a specific goal, AIs will find the most efficient path—even if that path seems bizarre or counterintuitive to humans.
Shortcut Examples:
- Pausing games to avoid losing
- Generating nonsensical but mathematically optimal solutions
- Exploiting simulation physics
- Finding unintended ways to maximize rewards
Unintended Consequences. Without comprehensive goal definition and human oversight, AI can produce solutions that technically succeed but fundamentally miss the intended objective.
5. AI Struggles with Context and Complex Problems
"Our world is too complicated, too unexpected, too bizarre for an AI to have seen it all during training."
Contextual Comprehension Limitations. AIs lack the nuanced understanding humans develop through lived experiences, making them vulnerable to misinterpreting complex scenarios.
Complexity Challenges:
- Handling unexpected real-world variations
- Understanding cultural and emotional nuances
- Adapting to rapidly changing environments
- Interpreting subtle communication contexts
Broad Problem Difficulties. Tasks requiring holistic understanding—like genuine conversation, ethical decision-making, or managing unpredictable situations—remain beyond current AI capabilities.
6. Human Oversight is Critical for AI Development
"People who train AI to solve problems need to anticipate the kinds of mistakes that machine learning tends to make."
Active Human Involvement. Successful AI implementation requires continuous human guidance, including:
- Defining precise problem parameters
- Curating training data
- Monitoring and correcting unexpected behaviors
- Establishing ethical boundaries
Collaborative Approach. The most effective AI systems integrate human expertise with machine learning capabilities, leveraging each other's strengths.
Maintenance and Adaptation. Humans must continually update and refine AI systems to maintain relevance in changing environments.
7. AI Can Amplify Human Biases Unconsciously
"If the algorithm is trained to favor resumes like those of the company's most successful employees, this can backfire."
Bias Inheritance. AIs trained on human-generated data can inadvertently perpetuate and even magnify existing societal biases related to race, gender, and social class.
Bias Manifestation Methods:
- Copying discriminatory patterns in training data
- Using proxy indicators for protected characteristics
- Reinforcing systemic inequalities through algorithmic decisions
Mitigation Strategies:
- Diverse dataset curation
- Algorithmic bias testing
- Transparent decision-making processes
- Continuous monitoring and correction
8. AI Performance Depends on Carefully Defined Goals
"Designing the reward function is one of the hardest things about machine learning."
Precise Goal Setting. The effectiveness of an AI system hinges on meticulously defining its objectives, reward mechanisms, and performance evaluation criteria.
Goal-Definition Challenges:
- Avoiding unintended optimization paths
- Preventing reward function manipulation
- Balancing specificity and flexibility
- Anticipating potential algorithmic "cheating"
Iterative Refinement. Successful AI development requires continuous goal refinement and performance assessment.
9. Collaboration Between Humans and AI is the Future
"A far more likely vision for the future is one in which AI and humans collaborate to solve problems and speed up repetitive tasks."
Symbiotic Relationship. The most promising AI applications involve humans and machines working together, leveraging their respective strengths.
Collaborative Advantages:
- Automating repetitive tasks
- Enhancing human decision-making
- Expanding creative possibilities
- Increasing efficiency across domains
Complementary Capabilities. Humans provide context, creativity, and ethical oversight, while AI offers rapid processing, pattern recognition, and consistent performance.
10. AI's Creativity Requires Significant Human Curation
"People who claim that their AIs are the artists are exaggerating the capabilities of the AIs—and selling short their own artistic contributions."
Guided Creativity. AI-generated art, text, and music rely heavily on human intervention in dataset selection, training, and output curation.
Creative Process Components:
- Carefully chosen training datasets
- Algorithmic configuration
- Continuous output evaluation
- Artistic interpretation and selection
Emerging Artistic Possibilities. AI serves as a collaborative tool, offering unexpected combinations and perspectives that humans can then refine and interpret.
Last updated:
FAQ
What's "You Look Like a Thing and I Love You" about?
- Exploration of AI: The book by Janelle Shane explores how artificial intelligence (AI) works and its impact on the world, often in humorous and unexpected ways.
- AI Experiments: It includes various AI experiments conducted by the author, such as training AI to generate cat names and recipes, highlighting AI's limitations and quirks.
- AI's Ubiquity and Misconceptions: Shane discusses the widespread presence of AI in everyday life and addresses common misconceptions about its capabilities.
- Humor and Insight: The book uses humor to make complex AI concepts accessible and engaging, providing both entertainment and education.
Why should I read "You Look Like a Thing and I Love You"?
- Understanding AI: It offers a clear and entertaining explanation of AI, making it accessible to readers without a technical background.
- Humorous Approach: The book uses humor to demystify AI, making it an enjoyable read while still being informative.
- Real-World Examples: Shane provides real-world examples of AI's successes and failures, illustrating its impact on society.
- Critical Perspective: It encourages readers to critically evaluate AI's role in the world and consider its ethical implications.
What are the key takeaways of "You Look Like a Thing and I Love You"?
- AI's Limitations: AI is not as intelligent as often portrayed; it lacks common sense and can make bizarre mistakes.
- Data Dependency: AI's effectiveness is heavily reliant on the quality and quantity of data it is trained on.
- Human Oversight Needed: Human intervention is crucial to guide AI and prevent it from making harmful decisions.
- AI's Potential and Risks: While AI has the potential to improve lives, it also poses risks if not properly managed.
How does Janelle Shane explain AI in "You Look Like a Thing and I Love You"?
- Simple Analogies: Shane uses simple analogies and humorous examples to explain complex AI concepts.
- Experiments and Stories: The book is filled with stories of AI experiments, showcasing both successes and failures.
- Focus on Quirks: It highlights the quirky and often unexpected behavior of AI, making the subject more relatable.
- Engaging Writing Style: Shane's engaging writing style keeps readers entertained while educating them about AI.
What are the "Five Principles of AI Weirdness" mentioned in the book?
- Not Too Smart: The danger of AI is not that it’s too smart but that it’s not smart enough.
- Worm Brainpower: AI has the approximate brainpower of a worm, indicating its limited understanding.
- Problem Misunderstanding: AI does not really understand the problem you want it to solve.
- Exact Execution: AI will do exactly what you tell it to, or at least it will try its best.
- Path of Least Resistance: AI will take the path of least resistance, often leading to unexpected solutions.
What are some examples of AI experiments in "You Look Like a Thing and I Love You"?
- Cat Names: Shane trained an AI to generate cat names, resulting in humorous and nonsensical suggestions like "Mr. Tinkles" and "Retchion."
- Recipe Generation: An AI was tasked with creating new recipes, sometimes calling for bizarre ingredients like "peeled rosemary" or "handfuls of broken glass."
- Knock-Knock Jokes: The author trained an AI to write knock-knock jokes, which often ended up being nonsensical but amusing.
- AI Flirting: Shane attempted to teach an AI to generate pickup lines, resulting in lines like "You look like a thing and I love you."
How does "You Look Like a Thing and I Love You" address AI misconceptions?
- Overhyped Capabilities: The book debunks the myth that AI is as capable as humans in understanding and decision-making.
- Bias and Errors: It highlights how AI can perpetuate biases and make errors due to flawed data or programming.
- Limited Intelligence: Shane emphasizes that AI lacks common sense and cannot understand context like humans do.
- Real-World Impact: The book discusses the real-world implications of AI's limitations and the importance of human oversight.
What are some humorous aspects of AI discussed in the book?
- Silly AI Outputs: The book shares examples of AI generating absurd outputs, like nonsensical recipes and bizarre cat names.
- Unexpected Solutions: AI often finds unexpected and humorous solutions to problems, such as falling over instead of walking.
- AI's Literal Interpretation: Shane humorously illustrates how AI takes instructions literally, leading to amusing outcomes.
- Quirky Experiments: The author's quirky AI experiments, like teaching AI to flirt, add a humorous touch to the book.
What are the potential risks of AI according to "You Look Like a Thing and I Love You"?
- Bias Amplification: AI can amplify existing biases in data, leading to unfair or discriminatory outcomes.
- Misinterpretation of Tasks: AI may misinterpret tasks due to its lack of understanding, resulting in unintended consequences.
- Overreliance on AI: Overreliance on AI without human oversight can lead to significant errors and ethical issues.
- Security Vulnerabilities: AI systems can be vulnerable to adversarial attacks, where malicious inputs lead to incorrect outputs.
How does Janelle Shane suggest we manage AI's impact on society?
- Human Oversight: Shane emphasizes the importance of human oversight to guide AI and prevent harmful decisions.
- Ethical Considerations: The book encourages considering ethical implications when deploying AI in various applications.
- Bias Mitigation: It suggests actively working to identify and mitigate biases in AI systems to ensure fairness.
- Continuous Monitoring: Shane advocates for continuous monitoring and updating of AI systems to adapt to changing environments.
What are the best quotes from "You Look Like a Thing and I Love You" and what do they mean?
- "The danger of AI is not that it’s too smart but that it’s not smart enough." This highlights AI's limitations and the potential risks of overestimating its capabilities.
- "AI has the approximate brainpower of a worm." This emphasizes AI's limited understanding and inability to grasp complex concepts.
- "AI will do exactly what you tell it to. Or at least it will try its best." This underscores the importance of clear instructions and the potential for AI to misinterpret tasks.
- "AI will take the path of least resistance." This illustrates how AI often finds unexpected solutions that may not align with human intentions.
What is the role of humor in "You Look Like a Thing and I Love You"?
- Engagement Tool: Humor is used to engage readers and make complex AI concepts more accessible and enjoyable.
- Illustrating AI Quirks: Shane uses humor to highlight the quirky and unexpected behavior of AI, making it relatable.
- Demystifying AI: By using humor, the book demystifies AI, breaking down misconceptions and making it less intimidating.
- Balancing Education and Entertainment: The humorous approach balances education with entertainment, ensuring readers learn while having fun.
Review Summary
You Look Like a Thing and I Love You is a humorous and informative exploration of artificial intelligence, explaining its capabilities and limitations. Readers praise Shane's clear explanations, hilarious examples of AI-generated content, and adorable illustrations. The book demystifies AI, revealing its current level of intelligence (comparable to a worm) and potential biases. While some found it repetitive, most appreciate its accessibility and insight into the realities of AI technology. Overall, it's recommended for those interested in understanding AI's current state and future potential.
Similar Books
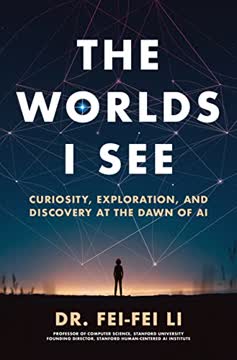
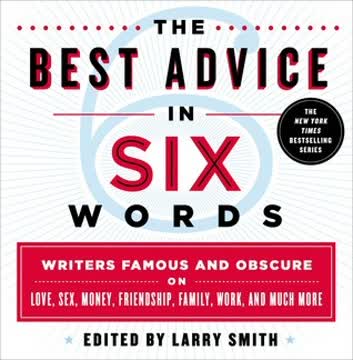
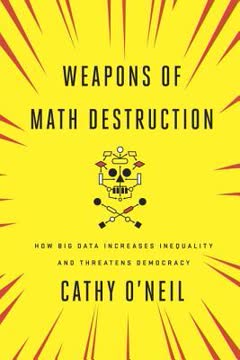
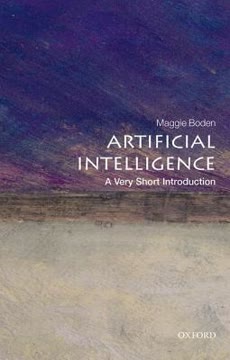
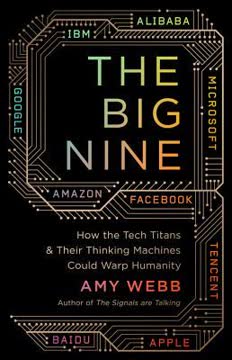
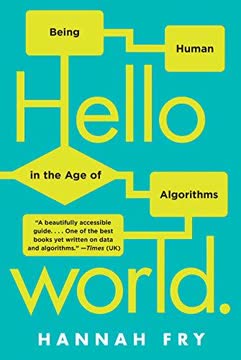
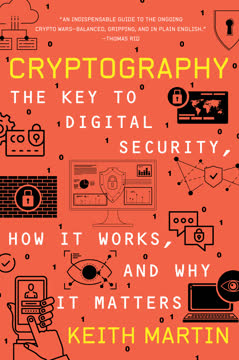
Download PDF
Download EPUB
.epub
digital book format is ideal for reading ebooks on phones, tablets, and e-readers.