نکات کلیدی
1. یادگیری ماشین به کامپیوترها این امکان را میدهد که از دادهها بدون برنامهنویسی صریح یاد بگیرند
یادگیری ماشین تنها کاربرد تجاری روشها برای استخراج اطلاعات از دادهها نیست؛ یادگیری همچنین یکی از الزامات هوش است.
هوش مبتنی بر داده. الگوریتمهای یادگیری ماشین به کامپیوترها این امکان را میدهند که عملکرد خود را در وظایف مختلف از طریق تجربه بهبود بخشند، نه با پیروی از قوانین از پیش برنامهریزی شده. این رویکرد بهویژه برای مسائلی که نوشتن الگوریتمهای صریح دشوار یا غیرممکن است، مانند شناسایی چهرهها یا درک گفتار، بسیار مؤثر است. با تحلیل مجموعههای داده بزرگ، مدلهای یادگیری ماشین میتوانند الگوها را کشف کرده و پیشبینیهایی انجام دهند بدون اینکه بهطور صریح به آنها گفته شود چگونه این کار را انجام دهند.
کاربردهای کلیدی:
- فیلتر کردن هرزنامه
- توصیههای محصول
- امتیازدهی اعتباری
- تشخیص پزشکی
- وسایل نقلیه خودران
یادگیری ماشین به نیروی محرکه بسیاری از پیشرفتهای اخیر در هوش مصنوعی تبدیل شده و این حوزه را پس از ناامیدیهای قبلی با سیستمهای خبره مبتنی بر قوانین احیا کرده است. با افزایش فراوانی دادهها و قدرت محاسباتی، تکنیکهای یادگیری ماشین احتمالاً نقش بیشتری در شکلدهی به آینده هوش مصنوعی و کاربردهای آن در صنایع مختلف ایفا خواهند کرد.
2. یادگیری نظارتشده ورودیها را به خروجیها متصل میکند، در حالی که یادگیری بدون نظارت الگوها را پیدا میکند
در یادگیری ماشین، هدف ساخت برنامهای است که با دادههای دادهشده مطابقت داشته باشد.
انواع یادگیری. یادگیری نظارتشده شامل آموزش مدلها بر روی دادههای برچسبگذاری شده است، جایی که هم ورودیها و هم خروجیهای مورد نظر ارائه میشوند. هدف این است که یک تابع یاد بگیریم که بتواند ورودیهای جدید را به خروجیهای صحیح بهطور دقیق متصل کند. وظایف رایج در یادگیری نظارتشده شامل طبقهبندی (تخصیص ورودیها به دستههای مجزا) و رگرسیون (پیشبینی مقادیر پیوسته) است.
یادگیری بدون نظارت، در مقابل، با دادههای بدون برچسب کار میکند تا ساختارها یا الگوهای پنهان را کشف کند. تکنیکهای کلیدی یادگیری بدون نظارت شامل:
- خوشهبندی: گروهبندی نقاط داده مشابه
- کاهش ابعاد: یافتن نمایشهای با ابعاد پایینتر از دادهها
- شناسایی ناهنجاری: شناسایی نقاط داده غیرمعمول یا پرت
هر دو رویکرد نقاط قوت خود را دارند و اغلب بهصورت ترکیبی استفاده میشوند. یادگیری نظارتشده در وظایف پیشبینی خاص برتری دارد، در حالی که یادگیری بدون نظارت میتواند بینشهای غیرمنتظرهای را فاش کند و بهویژه زمانی که دادههای برچسبگذاری شده کمیاب یا گرانقیمت هستند، مفید است.
3. شبکههای عصبی و یادگیری عمیق پیشرفتهای هوش مصنوعی را به حرکت در میآورند
روشهای یادگیری عمیق بهویژه بهخاطر نیاز کمتر به دخالت دستی جذاب هستند.
محاسبات الهامگرفته از مغز. شبکههای عصبی مصنوعی، که از ساختار مغزهای بیولوژیکی الهام گرفتهاند، شامل لایههای متصل به هم از واحدهای پردازش ساده هستند. یادگیری عمیق این مفهوم را با لایههای متعدد گسترش میدهد و به یادگیری خودکار ویژگیهای سلسلهمراتبی از دادههای خام اجازه میدهد. این رویکرد منجر به بهبودهای چشمگیری در زمینههایی مانند بینایی کامپیوتری، شناسایی گفتار و پردازش زبان طبیعی شده است.
مزایای کلیدی یادگیری عمیق:
- استخراج خودکار ویژگیها از دادههای خام
- توانایی درک روابط پیچیده و غیرخطی
- مقیاسپذیری به مجموعههای داده و اندازههای مدل بسیار بزرگ
معماریهای یادگیری عمیق قابل توجه:
- شبکههای عصبی کانولوشنی (CNN) برای پردازش تصویر
- شبکههای عصبی بازگشتی (RNN) برای دادههای توالی
- ترنسفورمرها برای وظایف پردازش زبان طبیعی
موفقیت یادگیری عمیق علاقه به رویکردهای شبکههای عصبی را دوباره زنده کرده و سرمایهگذاریهای قابل توجهی را در تحقیق و توسعه هوش مصنوعی در دانشگاهها و صنعت به همراه داشته است.
4. یادگیری تقویتی به سیستمها این امکان را میدهد که از طریق آزمون و خطا یاد بگیرند
اگرچه الگوریتمهای یادگیری تقویتی کندتر از الگوریتمهای یادگیری نظارتشده هستند، اما واضح است که آنها دامنه وسیعتری از کاربردها را دارند و پتانسیل ساخت ماشینهای یادگیری بهتری را دارند.
یادگیری از طریق عمل. یادگیری تقویتی به عاملها این امکان را میدهد که رفتارهای بهینه را از طریق تعامل با یک محیط یاد بگیرند. بر خلاف یادگیری نظارتشده، هیچ پاسخ "درست" از پیش تعیینشدهای وجود ندارد؛ در عوض، عامل یاد میگیرد که سیگنال پاداش را در طول زمان به حداکثر برساند. این رویکرد بهویژه برای مسائل تصمیمگیری توالیدار مناسب است و منجر به پیشرفتهایی در هوش مصنوعی بازی و رباتیک شده است.
اجزای کلیدی یادگیری تقویتی:
- عامل: یادگیرنده یا تصمیمگیرنده
- محیط: دنیایی که عامل با آن تعامل دارد
- حالت: وضعیت کنونی
- عمل: آنچه که عامل میتواند انجام دهد
- پاداش: بازخورد از محیط
موفقیتهای قابل توجه شامل:
- شکست دادن قهرمانان جهانی در بازی گو توسط AlphaGo از DeepMind
- رقابت ربات Dota 2 از OpenAI در سطح حرفهای
- یادگیری مهارتهای حرکتی پیچیده توسط سیستمهای رباتیک خودران
توانایی یادگیری تقویتی در یادگیری در محیطهای پیچیده و پویا، آن را به رویکردی امیدوارکننده برای توسعه سیستمهای هوش مصنوعی سازگارتر و عمومیتر تبدیل میکند.
5. دادههای کلان و محاسبات ابری پیشرفتهای یادگیری ماشین را تغذیه میکنند
ما دادههای کلان داریم، اما دادههای فردا بزرگتر خواهند بود.
مقیاس پیشرفت را به جلو میبرد. انفجار دادههای دیجیتال و در دسترس بودن منابع محاسبات ابری قدرتمند، عوامل کلیدی در پیشرفتهای اخیر یادگیری ماشین بودهاند. مجموعههای داده بزرگتر به مدلها این امکان را میدهند که الگوهای پیچیدهتری را یاد بگیرند، در حالی که محاسبات توزیعشده امکان آموزش و پیادهسازی الگوریتمهای پیچیدهتر را فراهم میکند.
روندهای کلیدی:
- رشد در تولید داده از دستگاههای IoT، رسانههای اجتماعی و تراکنشهای دیجیتال
- پیشرفت در فناوریهای ذخیرهسازی و پردازش داده
- دموکراتیزه کردن هوش مصنوعی از طریق پلتفرمهای یادگیری ماشین مبتنی بر ابر
همافزایی بین دادههای کلان و یادگیری ماشین یک چرخه مثبت ایجاد میکند: مدلهای بهتر منجر به کاربردهای مفیدتر میشوند که به نوبه خود دادههای بیشتری برای بهبودهای بیشتر تولید میکنند. این دینامیک احتمالاً به پیشرفت سریع در قابلیتهای هوش مصنوعی در حوزههای مختلف ادامه خواهد داد.
6. یادگیری ماشین نوآوریها را در بینایی کامپیوتری، شناسایی گفتار و پردازش زبان طبیعی به حرکت در میآورد
یادگیری ماشین نقش مهمی در خودروهای خودران ایفا میکند که منجر به رانندگی نرمتر، کنترل سریعتر و کارایی سوخت بیشتر میشود، اما همچنین در حسگرهای هوشمند، بهعنوان مثال، از طریق شناسایی خودکار عابران پیاده، دوچرخهسواران، علائم ترافیکی و غیره.
تحول در تعامل انسان و کامپیوتر. یادگیری ماشین به بهبودهای چشمگیری در نحوه درک و تعامل کامپیوترها با جهان منجر شده است. در بینایی کامپیوتری، مدلهای یادگیری عمیق اکنون میتوانند اشیاء، چهرهها و صحنهها را با دقت نزدیک به انسان شناسایی کنند. سیستمهای شناسایی گفتار قدرت دستیارهای مجازی و خدمات ترجمه در زمان واقعی را فراهم میکنند. الگوریتمهای پردازش زبان طبیعی در حال بهبود ترجمه ماشینی، خلاصهسازی متن و سیستمهای پرسش و پاسخ هستند.
حوزههای کاربرد کلیدی:
- وسایل نقلیه خودران
- تصویربرداری و تشخیص پزشکی
- دستگاههای هوشمند خانگی
- خدمات ترجمه زبان
- سیستمهای توصیه محتوا
این پیشرفتها تعامل انسان و کامپیوتر را طبیعیتر و شهودیتر میکنند و امکانات جدیدی برای ابزارها و خدمات هوش مصنوعی در صنایع مختلف ایجاد میکنند.
7. ملاحظات اخلاقی و حریم خصوصی دادهها در حالی که هوش مصنوعی بیشتر در حال گسترش است، حیاتی هستند
با جمعآوری و تحلیل دادههای بیشتر و بیشتر، تصمیمات ما در حوزههای مختلف بهطور فزایندهای خودکار و مبتنی بر داده خواهد شد و ما باید نگران پیامدهای چنین فرآیندهای خودمختار و تصمیماتی که اتخاذ میکنند، باشیم.
توسعه هوش مصنوعی مسئولانه. با قدرتمندتر و گستردهتر شدن سیستمهای یادگیری ماشین، ضروری است که به نگرانیهای اخلاقی و تأثیرات منفی بالقوه پرداخته شود. مسائل کلیدی شامل حریم خصوصی داده، تعصب الگوریتمی، شفافیت و قابلیت توضیح تصمیمات هوش مصنوعی و پیامدهای اجتماعی اتوماسیون است.
ملاحظات مهم:
- اطمینان از انصاف و عدم تبعیض در سیستمهای هوش مصنوعی
- حفاظت از حریم خصوصی فردی در جمعآوری و تحلیل دادهها
- توسعه مدلهای هوش مصنوعی قابل توضیح برای کاربردهای حیاتی
- رسیدگی به احتمال جابجایی شغلی بهدلیل اتوماسیون هوش مصنوعی
- ایجاد چارچوبهای قانونی برای توسعه و پیادهسازی هوش مصنوعی
محققان، سیاستگذاران و رهبران صنعت باید با هم همکاری کنند تا دستورالعملهای اخلاقی و بهترین شیوهها را برای توسعه مسئولانه هوش مصنوعی تدوین کنند. این شامل ترویج تنوع در تیمهای تحقیقاتی هوش مصنوعی، انجام ارزیابیهای تأثیر جامع و ایجاد گفتوگوهای عمومی درباره نقش هوش مصنوعی در جامعه است.
8. آینده هوش مصنوعی در سیستمهای هوشمندتر و سازگارتر نهفته است
چه زمانی به آن سطح از هوش خواهیم رسید و چه مقدار پردازش و آموزش لازم خواهد بود هنوز مشخص نیست. در حال حاضر، یادگیری ماشین بهنظر میرسد که امیدوارکنندهترین راه برای دستیابی به آن است، بنابراین با ما همراه باشید.
به سوی هوش عمومی مصنوعی. در حالی که سیستمهای هوش مصنوعی کنونی در انجام وظایف خاص برتری دارند، هدف بلندمدت توسعه هوش عمومی و انعطافپذیرتر است. این شامل ایجاد سیستمهایی است که بتوانند دانش را بین حوزهها منتقل کنند، با دادههای کمتری یاد بگیرند و بهراحتی به موقعیتهای جدید سازگار شوند.
جهتگیریهای تحقیقاتی امیدوارکننده:
- یادگیری متا: یادگیری نحوه یادگیری بهطور مؤثرتر
- یادگیری با چند نمونه: تعمیم از تعداد بسیار کمی مثال
- یادگیری چندمدلی: ادغام اطلاعات از انواع دادههای مختلف
- استدلال علّی: درک روابط علت و معلولی
مسیر به سمت سیستمهای هوش مصنوعی پیشرفتهتر احتمالاً شامل ترکیب بینشهای حاصل از یادگیری ماشین، علوم اعصاب، علوم شناختی و سایر رشتهها خواهد بود. با توانمندی بیشتر این سیستمها، ضروری است که اطمینان حاصل شود که آنها بهگونهای توسعه مییابند که با ارزشهای انسانی همراستا بوده و به نفع جامعه بهطور کلی باشد.
آخرین بهروزرسانی::
FAQ
What's "Machine Learning: The New AI" by Ethem Alpaydın about?
- Overview of Machine Learning: The book provides a comprehensive introduction to machine learning, explaining how it enables computers to learn from data and improve their performance over time.
- Historical Context: It traces the evolution of machine learning from its early days to its current status as a key component of artificial intelligence.
- Applications and Implications: The book discusses various applications of machine learning, from facial recognition to autonomous vehicles, and explores its implications for the future of technology and society.
- Educational Resource: It serves as an educational resource for those new to the field, offering insights into the fundamental concepts and methodologies of machine learning.
Why should I read "Machine Learning: The New AI" by Ethem Alpaydın?
- Comprehensive Introduction: The book is an excellent starting point for anyone interested in understanding the basics of machine learning and its role in artificial intelligence.
- Expert Insights: Written by a leading expert in the field, it provides authoritative insights into the principles and practices of machine learning.
- Real-World Applications: It offers numerous examples of how machine learning is applied in various industries, making the concepts more relatable and easier to understand.
- Future Implications: The book discusses the potential future developments in machine learning, helping readers understand its long-term impact on technology and society.
What are the key takeaways of "Machine Learning: The New AI"?
- Learning from Data: Machine learning involves creating algorithms that allow computers to learn from and make predictions based on data.
- Supervised vs. Unsupervised Learning: The book explains the difference between supervised learning, where models are trained on labeled data, and unsupervised learning, which involves finding patterns in unlabeled data.
- Neural Networks and Deep Learning: It covers the basics of neural networks and the concept of deep learning, which involves training models with multiple layers of abstraction.
- Ethical Considerations: The book highlights the ethical and privacy concerns associated with machine learning, emphasizing the need for responsible use of technology.
What are the best quotes from "Machine Learning: The New AI" and what do they mean?
- "A quiet revolution has been taking place in computer science for the last two decades." This quote highlights the transformative impact of machine learning on the field of computer science.
- "Machine learning is not just the commercial application of methods to extract information from data; learning is also a requisite of intelligence." It underscores the importance of machine learning in developing intelligent systems.
- "The aim of this book is to give the reader an overall idea about what machine learning is, the basics of some important learning algorithms, and a set of example applications." This quote encapsulates the book's purpose as an educational resource for understanding machine learning.
How does Ethem Alpaydın explain the concept of supervised learning in "Machine Learning: The New AI"?
- Definition and Process: Supervised learning is described as a process where models are trained on labeled data, meaning the input data is paired with the correct output.
- Examples Provided: The book provides examples such as credit scoring and image recognition to illustrate how supervised learning is applied in real-world scenarios.
- Model Training: It explains how models learn to make predictions by minimizing the difference between their predictions and the actual outcomes in the training data.
- Importance of Labeled Data: The book emphasizes the necessity of having a large and diverse set of labeled data to train effective supervised learning models.
What is unsupervised learning according to "Machine Learning: The New AI"?
- Definition and Purpose: Unsupervised learning involves finding patterns or structures in data without pre-existing labels, aiming to discover hidden relationships.
- Clustering and Association: The book discusses methods like clustering, which groups similar data points, and association, which finds rules that describe large portions of the data.
- Applications: Examples include customer segmentation and market basket analysis, where unsupervised learning helps identify natural groupings or associations in data.
- Exploratory Nature: It highlights the exploratory nature of unsupervised learning, which can reveal insights that were not previously considered.
How does "Machine Learning: The New AI" describe neural networks and deep learning?
- Neural Networks Basics: The book explains neural networks as models composed of interconnected nodes (neurons) that process information in layers.
- Deep Learning Concept: Deep learning is described as using neural networks with many layers to learn complex patterns in data, allowing for high-level abstractions.
- Applications and Successes: It discusses the success of deep learning in fields like image and speech recognition, where it has achieved state-of-the-art results.
- Training Challenges: The book also addresses the challenges of training deep networks, such as the need for large datasets and significant computational resources.
What role does probability play in machine learning according to Ethem Alpaydın?
- Modeling Uncertainty: Probability is used to model uncertainty in data and predictions, allowing for more robust decision-making.
- Bayesian Methods: The book covers Bayesian methods, which incorporate prior knowledge into the learning process to improve model predictions.
- Randomness in Data: It explains how probability helps account for randomness and noise in data, which can affect the accuracy of machine learning models.
- Decision Making: Probability is crucial in decision-making processes, such as calculating expected values to choose the best course of action.
What ethical considerations are discussed in "Machine Learning: The New AI"?
- Data Privacy: The book emphasizes the importance of protecting individuals' privacy when collecting and analyzing data for machine learning.
- Bias and Fairness: It discusses the potential for bias in machine learning models and the need for fairness in their development and deployment.
- Transparency and Accountability: The book advocates for transparency in how machine learning models are trained and used, ensuring accountability for their outcomes.
- Impact on Society: It highlights the broader societal implications of machine learning, including its potential to disrupt industries and affect employment.
How does "Machine Learning: The New AI" address the future of machine learning?
- Continued Growth: The book predicts continued growth and integration of machine learning into various aspects of technology and daily life.
- Advancements in Algorithms: It anticipates advancements in algorithms that will enable more efficient and accurate learning from data.
- Interdisciplinary Impact: The book foresees machine learning impacting a wide range of fields, from healthcare to finance, by providing new insights and capabilities.
- Ethical and Regulatory Challenges: It acknowledges the ethical and regulatory challenges that will need to be addressed as machine learning becomes more pervasive.
What is the significance of reinforcement learning in "Machine Learning: The New AI"?
- Learning from Interaction: Reinforcement learning is described as a method where agents learn by interacting with their environment and receiving feedback in the form of rewards.
- Applications in Gaming: The book highlights its use in gaming, where reinforcement learning has been used to develop agents that can play complex games like Go and chess.
- Delayed Rewards: It explains the concept of delayed rewards, where the agent must learn to associate actions with outcomes that occur much later.
- Exploration vs. Exploitation: The book discusses the balance between exploration (trying new actions) and exploitation (using known actions) to maximize rewards.
How does Ethem Alpaydın explain the concept of clustering in "Machine Learning: The New AI"?
- Grouping Similar Data: Clustering is described as the process of grouping similar data points together based on their attributes.
- Unsupervised Learning Method: It is an unsupervised learning method, meaning it does not rely on labeled data to find patterns.
- Applications in Segmentation: The book provides examples such as customer segmentation, where clustering helps identify distinct groups within a dataset.
- Exploratory Analysis: Clustering is used for exploratory data analysis, revealing natural groupings that may not have been previously considered.
نقد و بررسی
کتاب یادگیری ماشین با نظرات متنوعی مواجه شده و میانگین امتیاز آن ۳.۶۲ از ۵ است. خوانندگان از معرفی قابل فهم مفاهیم یادگیری ماشین در این کتاب قدردانی میکنند، هرچند برخی آن را بیش از حد ابتدایی یا خشک میدانند. این کتاب به خاطر تعادل بین جزئیات فنی و توضیحات کلی مورد تحسین قرار گرفته و برای غیرمتخصصانی که به دنبال یک مرور کلی هستند، مناسب است. با این حال، برخی به کمبود مثالهای عملی و اطلاعات قدیمی آن انتقاد کردهاند. در حالی که این کتاب برای درک مفاهیم بنیادی ارزشمند است، ممکن است نتواند نیاز کسانی را که به دنبال دانش فنی عمیق یا کاربرد عملی هستند، برآورده کند.
Similar Books
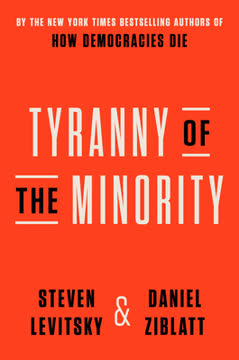
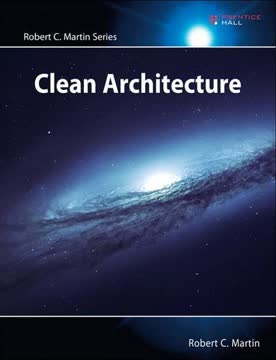
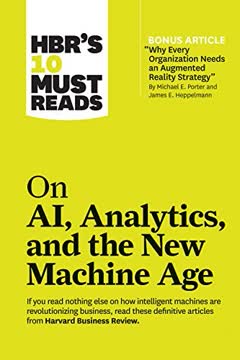
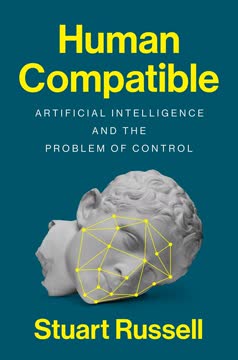
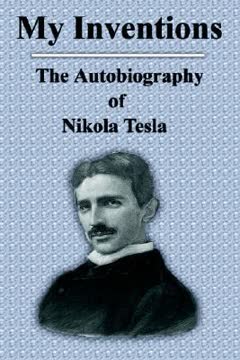
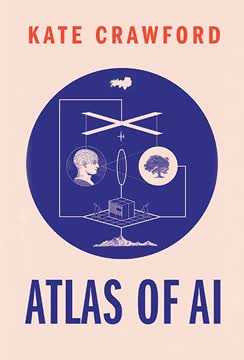