نکات کلیدی
1. مبانی یادگیری ماشین: یادگیری تحت نظارت و یادگیری بدون نظارت
یادگیری ماشین جایی است که مهارتهای محاسباتی و الگوریتمی علم داده با تفکر آماری علم داده تلاقی میکند و نتیجه آن مجموعهای از رویکردها برای استنتاج و کاوش دادهها است که بیشتر به محاسبات مؤثر مربوط میشود تا نظریه مؤثر.
یادگیری تحت نظارت شامل مدلسازی روابط بین ویژگیهای ورودی و خروجیهای برچسبگذاری شده است. این نوع یادگیری شامل وظایف طبقهبندی است که هدف آن پیشبینی دستههای گسسته و وظایف رگرسیون است که به پیشبینی مقادیر پیوسته میپردازد. به عنوان مثال، پیشبینی قیمت مسکن یا طبقهبندی ایمیلها به عنوان هرزنامه.
یادگیری بدون نظارت بر کشف الگوها در دادههای بدون برچسب تمرکز دارد. تکنیکهای کلیدی شامل:
- خوشهبندی: گروهبندی نقاط داده مشابه
- کاهش ابعاد: سادهسازی دادههای پیچیده در حالی که اطلاعات اساسی حفظ میشود
این مفاهیم بنیادی، پایه و اساس یادگیری ماشین را تشکیل میدهند و چارچوبی برای مقابله با چالشهای مختلف تحلیل دادهها فراهم میکنند.
2. Scikit-Learn: کتابخانهای قدرتمند برای یادگیری ماشین در پایتون
Scikit-Learn مجموعهای از ابزارهای کارآمد برای یادگیری ماشین و مدلسازی آماری از جمله طبقهبندی، رگرسیون، خوشهبندی و کاهش ابعاد را از طریق یک رابط کاربری یکسان در پایتون ارائه میدهد.
طراحی API یکسان باعث میشود که Scikit-Learn کاربرپسند و کارآمد باشد. این کتابخانه الگوی یکنواختی را برای تمام مدلهای خود دنبال میکند:
- انتخاب یک کلاس و وارد کردن آن
- ایجاد نمونهای از کلاس با هایپرپارامترهای مورد نظر
- تطبیق مدل با دادههای شما
- اعمال مدل بر روی دادههای جدید
این روند استاندارد به کاربران این امکان را میدهد که به راحتی بین الگوریتمهای مختلف بدون تغییرات قابل توجه در کد جابجا شوند. Scikit-Learn همچنین به طور یکپارچه با سایر کتابخانههای علمی پایتون مانند NumPy و Pandas ادغام میشود و آن را به ابزاری چندمنظوره برای پروژههای علم داده تبدیل میکند.
3. نمایش داده و پیشپردازش در Scikit-Learn
بهترین راه برای فکر کردن به دادهها در Scikit-Learn، در قالب جداول داده است.
فرمتبندی صحیح دادهها برای یادگیری ماشین مؤثر بسیار حیاتی است. Scikit-Learn انتظار دارد که دادهها در یک فرمت خاص باشند:
- ماتریس ویژگیها (X): ساختار شبیه به آرایه 2 بعدی با شکل [n_samples, n_features]
- آرایه هدف (y): آرایه 1 بعدی با طول n_samples
مراحل پیشپردازش معمولاً شامل:
- مدیریت دادههای گمشده از طریق تخمین
- مقیاسبندی ویژگیها به یک دامنه مشترک
- کدگذاری متغیرهای دستهای
- انتخاب ویژگی یا کاهش ابعاد
Scikit-Learn ابزارهای مختلفی برای این وظایف پیشپردازش ارائه میدهد، مانند SimpleImputer برای دادههای گمشده و StandardScaler برای مقیاسبندی ویژگیها. پیشپردازش صحیح اطمینان میدهد که الگوریتمها به طور بهینه عمل کنند و نتایج قابل اعتمادی تولید کنند.
4. انتخاب مدل و تکنیکهای اعتبارسنجی
یک مدل تنها به اندازه پیشبینیهایش خوب است.
اعتبارسنجی متقابل یک تکنیک حیاتی برای ارزیابی عملکرد مدل و جلوگیری از بیشبرازش است. این فرآیند شامل:
- تقسیم دادهها به مجموعههای آموزشی و آزمایشی
- آموزش مدل بر روی دادههای آموزشی
- ارزیابی عملکرد بر روی دادههای آزمایشی
Scikit-Learn ابزارهایی مانند train_test_split برای تقسیمات ساده و cross_val_score برای اعتبارسنجی متقابل k-fold پیشرفته ارائه میدهد. این روشها به:
- برآورد عملکرد مدل بر روی دادههای دیدهنشده
- مقایسه مدلها یا هایپرپارامترهای مختلف
- شناسایی بیشبرازش یا کمبرازش کمک میکنند
علاوه بر این، تکنیکهایی مانند منحنیهای یادگیری و منحنیهای اعتبارسنجی به تجسم عملکرد مدل در اندازههای مختلف مجموعههای آموزشی و مقادیر هایپرپارامترها کمک میکنند و فرآیند انتخاب مدل را راهنمایی میکنند.
5. مهندسی ویژگی: تبدیل دادههای خام به ورودیهای مفید
یکی از مراحل مهم در استفاده از یادگیری ماشین در عمل، مهندسی ویژگی است — یعنی تبدیل هر اطلاعاتی که درباره مشکل خود دارید به اعدادی که میتوانید برای ساخت ماتریس ویژگی خود استفاده کنید.
مهندسی ویژگی مؤثر میتواند به طور قابل توجهی عملکرد مدل را بهبود بخشد. تکنیکهای رایج شامل:
- ایجاد ویژگیهای چندجملهای برای ضبط روابط غیرخطی
- تقسیم متغیرهای پیوسته به دستههای گسسته
- کدگذاری متغیرهای دستهای با استفاده از کدگذاری یکداغ یا کدگذاری هدف
- استخراج ویژگیهای متنی با استفاده از تکنیکهایی مانند TF-IDF
- ترکیب ویژگیهای موجود برای ایجاد ویژگیهای جدید و معنادار
Scikit-Learn ابزارهای مختلفی برای مهندسی ویژگی ارائه میدهد، مانند PolynomialFeatures برای ایجاد ویژگیهای چندجملهای و تعامل و CountVectorizer یا TfidfVectorizer برای دادههای متنی. هنر مهندسی ویژگی اغلب به دانش دامنه و خلاقیت نیاز دارد تا مرتبطترین اطلاعات را از دادههای خام استخراج کند.
6. بیز ساده: الگوریتمهای طبقهبندی سریع و ساده
مدلهای بیز ساده گروهی از الگوریتمهای طبقهبندی بسیار سریع و ساده هستند که اغلب برای مجموعههای داده با ابعاد بسیار بالا مناسب هستند.
رویکرد احتمالی اساس طبقهبندهای بیز ساده را تشکیل میدهد که بر اساس نظریه بیز است. ویژگیهای کلیدی شامل:
- زمانهای آموزش و پیشبینی سریع
- عملکرد خوب با دادههای با ابعاد بالا
- توانایی مدیریت دادههای پیوسته و گسسته
انواع طبقهبندهای بیز ساده:
- بیز ساده گاوسی: فرض میکند که ویژگیها توزیع نرمال دارند
- بیز ساده چندجملهای: مناسب برای دادههای گسسته، اغلب در طبقهبندی متنی استفاده میشود
- بیز ساده برنولی: برای وکتورهای ویژگی دوتایی استفاده میشود
با وجود سادگیشان، طبقهبندهای بیز ساده اغلب عملکرد شگفتانگیزی دارند، به ویژه در وظایف طبقهبندی متنی. آنها به عنوان مبنای عالی عمل میکنند و به ویژه زمانی که منابع محاسباتی محدود هستند، بسیار مفیدند.
7. رگرسیون خطی: پایهای برای مدلسازی پیشبینی
مدلهای رگرسیون خطی نقطه شروع خوبی برای وظایف رگرسیون هستند.
قابلیت تفسیر و سادگی رگرسیون خطی را به انتخابی محبوب برای بسیاری از وظایف مدلسازی پیشبینی تبدیل میکند. مفاهیم کلیدی شامل:
- حداقل مربعات معمولی (OLS) برای یافتن خط بهترین برازش
- رگرسیون خطی چندگانه برای مدیریت چندین ویژگی ورودی
- تکنیکهای منظمسازی مانند رگرسیون لاسو و ریج برای جلوگیری از بیشبرازش
رگرسیون خطی به عنوان یک بلوک سازنده برای مدلهای پیچیدهتر عمل میکند و مزایایی از جمله:
- تفسیر آسان اهمیت ویژگیها
- زمانهای آموزش و پیشبینی سریع
- پایهای برای درک تکنیکهای رگرسیون پیشرفتهتر را ارائه میدهد
در حالی که در ضبط روابط غیرخطی محدود است، رگرسیون خطی میتواند از طریق ویژگیهای چندجملهای یا رگرسیون تابع پایه برای مدلسازی الگوهای پیچیدهتر در دادهها گسترش یابد.
آخرین بهروزرسانی::
نقد و بررسی
کتاب راهنمای علم داده با پایتون عمدتاً نظرات مثبتی دریافت کرده و به خاطر رویکرد عملی و توضیحات واضحش در مورد ابزارهای اساسی مانند NumPy، Pandas و Matplotlib ستایش شده است. خوانندگان از عمق مطالب مربوط به دستکاری و تجسم دادهها قدردانی میکنند. فصل یادگیری ماشین به عنوان یک مقدمه خوب در نظر گرفته میشود، هرچند برخی آن را از نظر عمق ناکافی میدانند. این کتاب برای مبتدیان و به عنوان مرجعی برای کاربران با تجربه توصیه میشود. برخی از منتقدان اشاره میکنند که برخی بخشها ممکن است قدیمی شده باشند و چند نفر نیز به کمبود تمرینها و مثالهای واقعی انتقاد کردهاند.
Similar Books
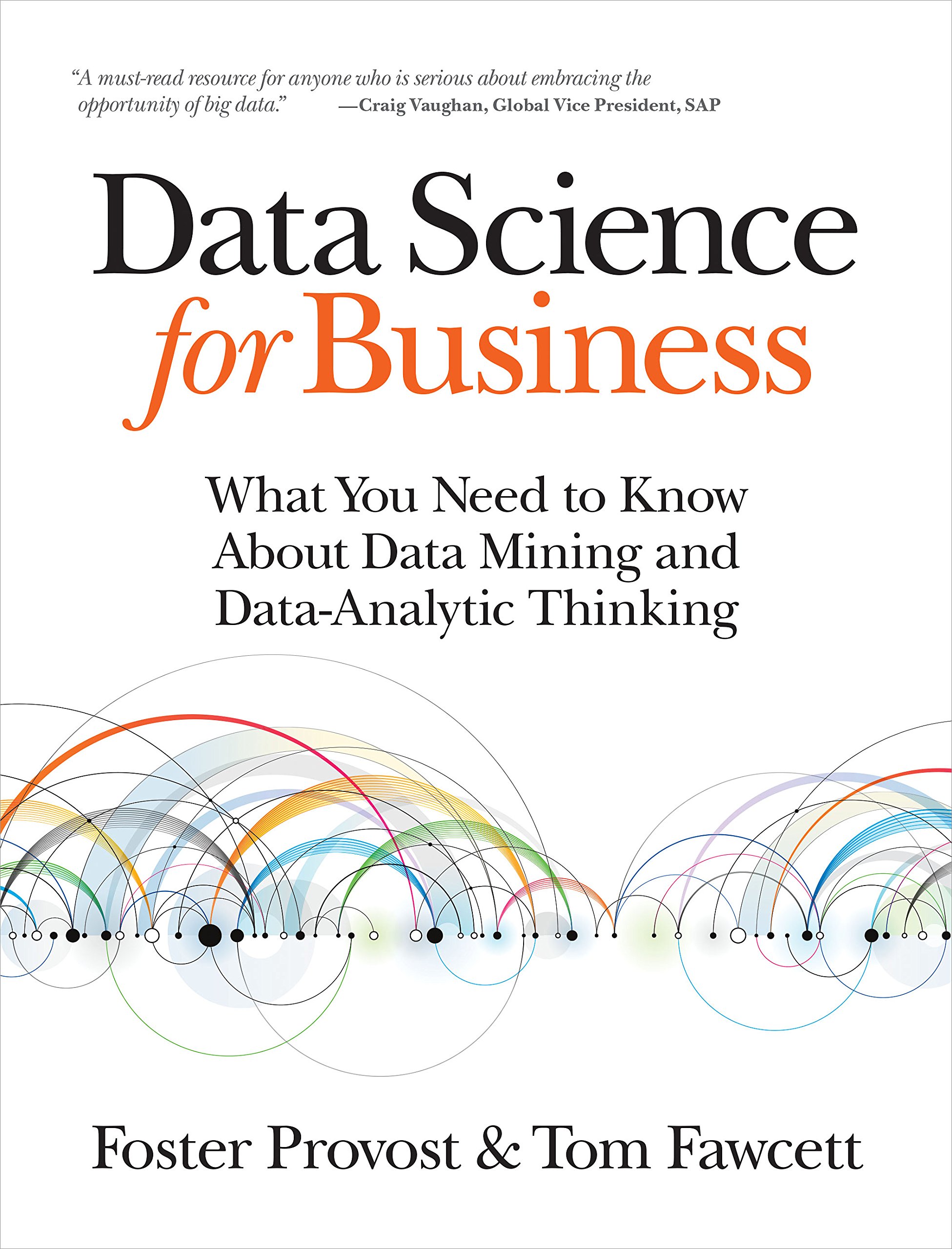
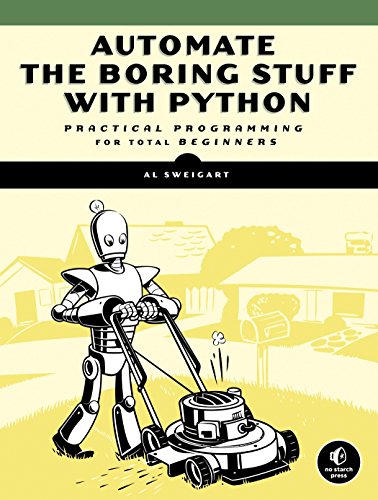
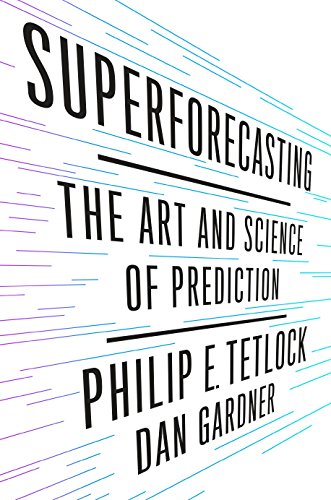
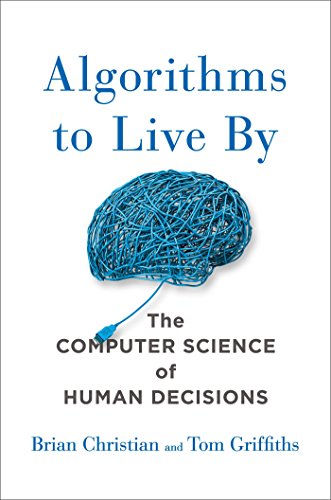
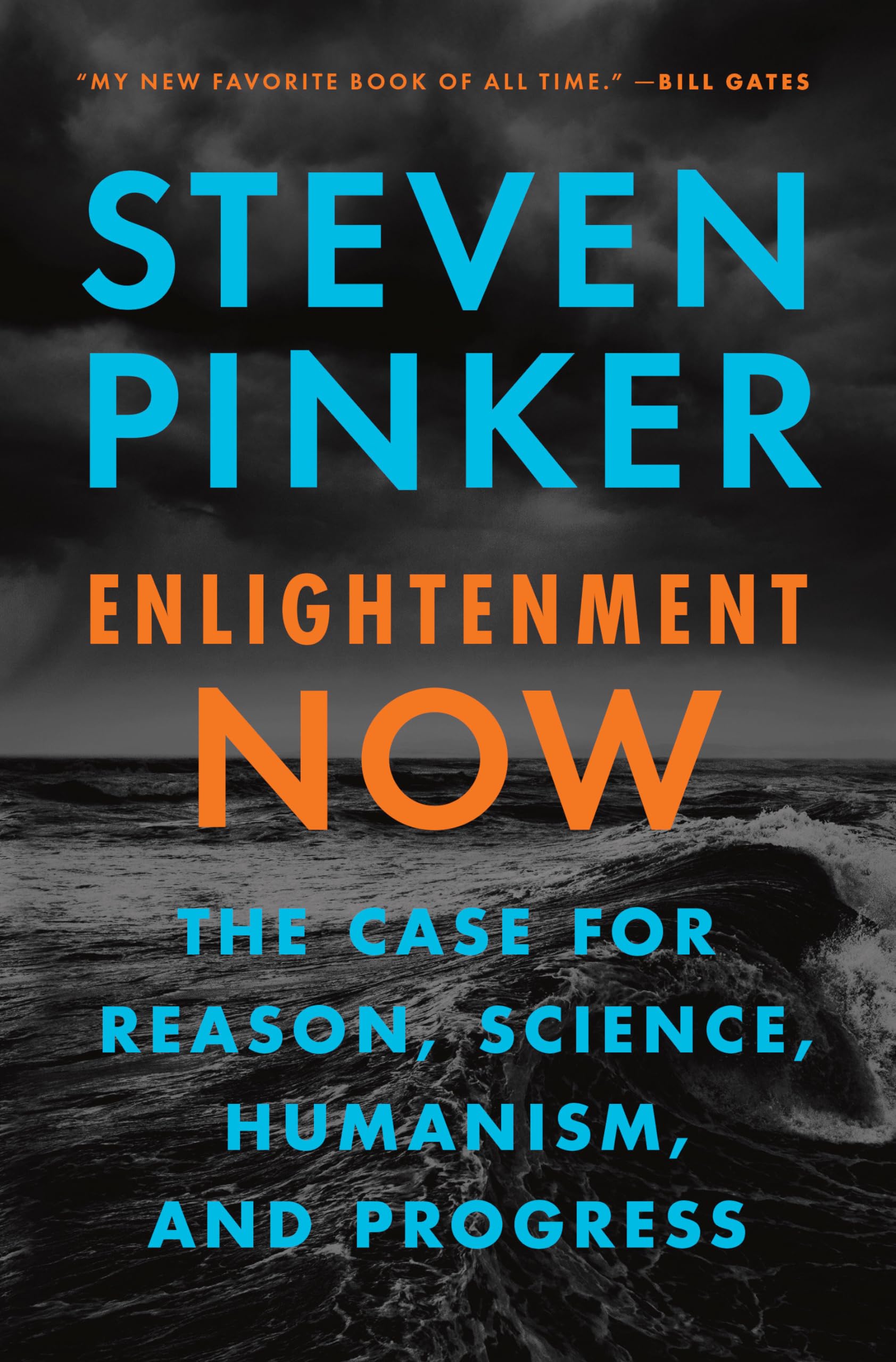
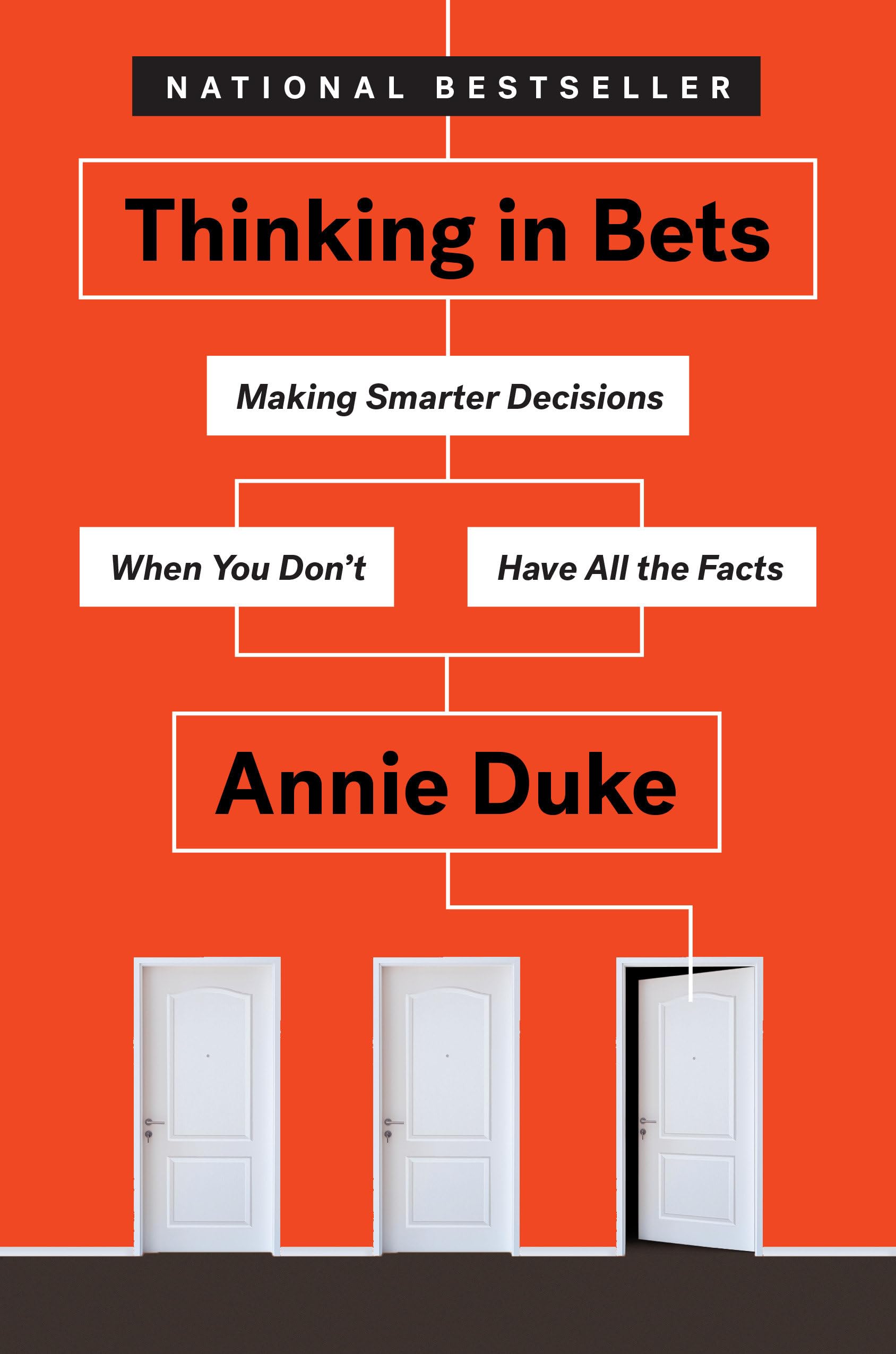
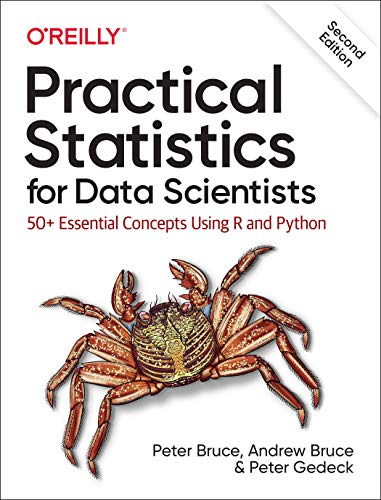
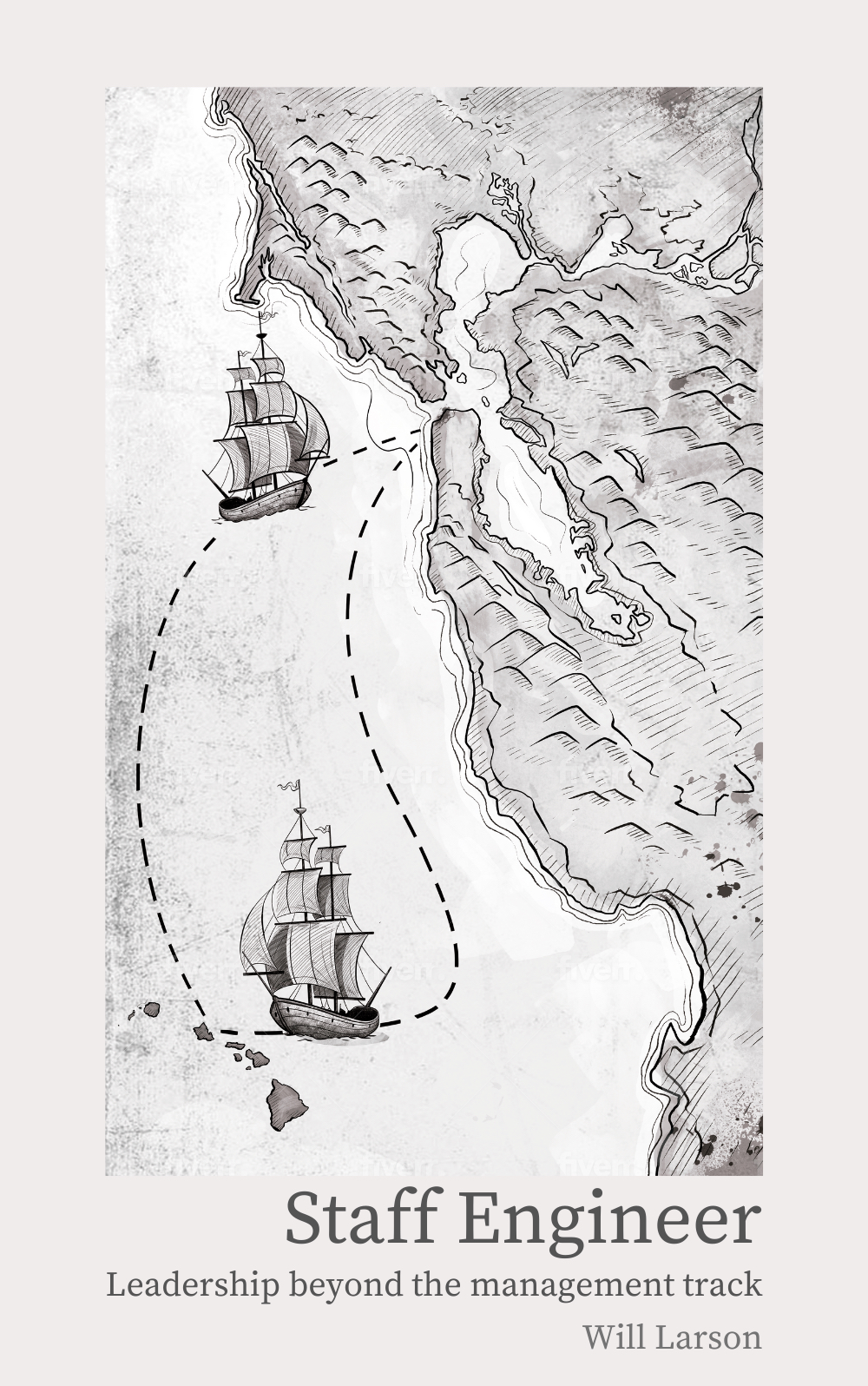