मुख्य निष्कर्ष
1. जोखिम प्रबंधन में खामियां हैं और इसे सुधारने की आवश्यकता है
सबसे बड़े जोखिम अक्सर वे होते हैं जो अधिक दुर्लभ होते हैं लेकिन संभावित रूप से विनाशकारी हो सकते हैं—शायद ऐसे घटनाएं जो इस संगठन में अभी तक नहीं हुई हैं।
वर्तमान स्थिति का जोखिम प्रबंधन। कई संगठन जोखिमों का आकलन और प्रबंधन करने के लिए अप्रभावी तरीकों पर निर्भर करते हैं। ये तरीके अक्सर सबसे महत्वपूर्ण खतरों की पहचान करने या उन्हें सही तरीके से मूल्यांकित करने में विफल रहते हैं। जोखिम प्रबंधन की प्रथाएं अक्सर नियमित, आसानी से मापने योग्य जोखिमों पर ध्यान केंद्रित करती हैं जबकि दुर्लभ लेकिन संभावित रूप से विनाशकारी घटनाओं को नजरअंदाज कर देती हैं।
सुधार की आवश्यकता। प्रभावी जोखिम प्रबंधन के लिए आवश्यक है:
- एक व्यापक दृष्टिकोण जो सभी संभावित जोखिमों पर विचार करता है
- संभावनाओं और प्रभावों का सटीक आकलन करने के लिए मात्रात्मक तरीके
- जोखिम आकलनों का नियमित मूल्यांकन और अद्यतन
- समग्र निर्णय-निर्माण प्रक्रियाओं में जोखिम प्रबंधन का एकीकरण
2. लोकप्रिय जोखिम आकलन विधियां अक्सर बेकार होती हैं
जोखिम मैट्रिक्स गलती से मात्रात्मक रूप से छोटे जोखिमों को उच्च गुणात्मक रेटिंग दे सकते हैं। नकारात्मक रूप से सहसंबंधित आवृत्तियों और गंभीरताओं वाले जोखिमों के लिए, ये "बेकार से भी बदतर" हो सकते हैं, जिससे खराब निर्णय लेने की स्थिति उत्पन्न होती है।
गुणात्मक विधियों की समस्याएं। कई संगठन जोखिमों का आकलन करने के लिए जोखिम मैट्रिक्स या अन्य गुणात्मक स्कोरिंग सिस्टम का उपयोग करते हैं। इन विधियों में कई महत्वपूर्ण खामियां हैं:
- संभावना और प्रभाव श्रेणियों की परिभाषाओं में अस्पष्टता
- जोखिमों की सटीक तुलना या प्राथमिकता देने में असमर्थता
- जटिल जोखिम परिदृश्यों को सरल बनाने की प्रवृत्ति
- जोखिमों के बीच सहसंबंधों की अनदेखी
खराब विधियों के परिणाम। दोषपूर्ण जोखिम आकलन तकनीकों का उपयोग करने से निम्नलिखित समस्याएं उत्पन्न हो सकती हैं:
- जोखिम न्यूनीकरण के लिए संसाधनों का गलत आवंटन
- प्रमुख जोखिमों के बारे में झूठी सुरक्षा की भावना
- संभावित विनाशकारी घटनाओं की अनदेखी
- गलत जोखिम जानकारी के आधार पर खराब निर्णय लेना
3. प्रभावी जोखिम प्रबंधन के लिए मात्रात्मक विधियां आवश्यक हैं
जीवन के सबसे महत्वपूर्ण प्रश्न, अधिकांशतः, वास्तव में केवल संभाव्यता की समस्याएं होती हैं।
मात्रात्मक दृष्टिकोण के लाभ। मात्रात्मक जोखिम आकलन विधियां कई लाभ प्रदान करती हैं:
- संभावनाओं और संभावित प्रभावों का सटीक मापन
- विविध जोखिमों की तुलना और प्राथमिकता देने की क्षमता
- ऐतिहासिक डेटा और विशेषज्ञ निर्णय का एकीकरण
- डेटा-आधारित निर्णय लेने का समर्थन
मुख्य मात्रात्मक उपकरण:
- अनिश्चितता को मॉडल करने के लिए संभाव्यता वितरण
- जटिल परिदृश्यों के लिए मोंटे कार्लो सिमुलेशन
- नई जानकारी के साथ संभावनाओं को अद्यतन करने के लिए बेयesian विधियां
- वैल्यू एट रिस्क (VaR) और अन्य वित्तीय जोखिम मैट्रिक्स
4. विशेषज्ञ निर्णयों की कैलिब्रेशन और निरंतर मूल्यांकन की आवश्यकता है
कहा जाता है कि सच्चे विशेषज्ञ जानते हैं जब वे नहीं जानते। हालाँकि, गैर-विशेषज्ञ (चाहे वे सोचते हैं कि वे हैं या नहीं) निश्चित रूप से नहीं जानते जब वे नहीं जानते।
विशेषज्ञ निर्णयों की चुनौतियां। जोखिम आकलन के लिए विशेषज्ञ राय पर निर्भर रहना समस्याग्रस्त हो सकता है क्योंकि:
- अनुमानों में अधिक आत्मविश्वास
- निर्णयों में असंगति
- जोखिम धारणा को प्रभावित करने वाले संज्ञानात्मक पूर्वाग्रह
- कई विशेषज्ञों की राय को संयोजित करने में कठिनाई
विशेषज्ञ इनपुट में सुधार:
- संभाव्यता आकलनों में सुधार के लिए कैलिब्रेशन प्रशिक्षण
- पूर्वाग्रह को कम करने के लिए संरचित निष्कर्षण तकनीकें
- कई विशेषज्ञों की राय का प्रदर्शन-भारित समेकन
- वास्तविक परिणामों के खिलाफ विशेषज्ञ निर्णयों की नियमित फीडबैक और मूल्यांकन
5. बेयesian विधियां और अनुभवजन्य परीक्षण जोखिम मॉडलों में सुधार करते हैं
बेयस का प्रमेय एक सरल लेकिन शक्तिशाली गणितीय उपकरण है और इसे जोखिम विश्लेषकों के लिए ऐसे स्थितियों का मूल्यांकन करने के लिए एक बुनियादी उपकरण होना चाहिए।
बेयesian विश्लेषण की शक्ति। बेयesian विधियां नए डेटा उपलब्ध होने पर जोखिम आकलनों को अद्यतन करने के लिए एक मजबूत ढांचा प्रदान करती हैं। इसके प्रमुख लाभों में शामिल हैं:
- पूर्व ज्ञान और नए डेटा का समावेश
- छोटे नमूना आकार और दुर्लभ घटनाओं को संभालने की क्षमता
- समय के साथ जोखिम अनुमानों में निरंतर सुधार
अनुभवजन्य परीक्षण का महत्व। जोखिम मॉडलों को नियमित रूप से वास्तविक दुनिया के परिणामों के खिलाफ मान्य किया जाना चाहिए:
- ऐतिहासिक डेटा के खिलाफ मॉडल का बैकटेस्टिंग
- मॉडल भविष्यवाणियों का ट्रैकिंग और वास्तविक परिणामों की तुलना
- देखी गई प्रदर्शन के आधार पर मॉडलों को समायोजित करना
- समग्र जोखिम आकलन प्रथाओं में सुधार के लिए मेटा-विश्लेषण का उपयोग करना
6. मोंटे कार्लो सिमुलेशन जोखिम विश्लेषण के लिए शक्तिशाली उपकरण हैं
मोंटे कार्लो निश्चित रूप से मूल्य पर जोखिम की गणना करने के लिए सबसे शक्तिशाली विधि है।
मोंटे कार्लो सिमुलेशन के लाभ:
- कई अनिश्चितताओं के साथ जटिल प्रणालियों को मॉडल करने की क्षमता
- संभावित परिणामों के लिए संभाव्यता वितरण का निर्माण
- विभिन्न प्रकार के जोखिम कारकों को शामिल करने की लचीलापन
- परिदृश्य विश्लेषण और तनाव परीक्षण का समर्थन
मोंटे कार्लो विधियों को लागू करना:
- प्रमुख जोखिम चर और उनके संभाव्यता वितरण की पहचान करना
- चर के बीच संबंधों और सहसंबंधों को परिभाषित करना
- परिणाम उत्पन्न करने के लिए हजारों यादृच्छिक सिमुलेशन चलाना
- जोखिम प्रोफाइल और संभावित प्रभावों को समझने के लिए परिणामों का विश्लेषण करना
7. संगठनात्मक संस्कृति और प्रोत्साहन जोखिम प्रबंधन की सफलता के लिए महत्वपूर्ण हैं
मैंने अपने तीस वर्षों में जो सबसे बड़ा सुधार देखा है, वह सहकर्मी समीक्षा है। सब कुछ इस कठोर फ़िल्टर से गुजरता है इससे पहले कि यह प्रबंधन के निर्णय लेने के लिए जाए।
जोखिम-जानकारी संस्कृति का निर्माण। प्रभावी जोखिम प्रबंधन के लिए संगठनात्मक प्रतिबद्धता की आवश्यकता होती है:
- कठोर जोखिम आकलन के लिए नेतृत्व का समर्थन
- सभी निर्णय-निर्माण प्रक्रियाओं में जोखिम विचारों का एकीकरण
- संभावित जोखिमों के बारे में खुली संचार को प्रोत्साहित करना
- निरंतर सीखने और सुधार की संस्कृति को बढ़ावा देना
अच्छे जोखिम प्रबंधन के साथ प्रोत्साहनों को संरेखित करना:
- आशावादी पूर्वानुमानों के बजाय सटीक जोखिम आकलनों को पुरस्कृत करना
- कर्मचारी मूल्यांकन में जोखिम प्रबंधन प्रदर्शन को शामिल करना
- बेहतर जोखिम विश्लेषण के लिए संसाधन और प्रशिक्षण प्रदान करना
- जोखिम से संबंधित निर्णयों और परिणामों के लिए स्पष्ट जिम्मेदारी स्थापित करना
अंतिम अपडेट:
FAQ
What's The Failure of Risk Management about?
- Critique of Current Practices: The book critiques traditional risk management methods, particularly qualitative ones, arguing they often lead to poor decision-making.
- Call for Quantitative Methods: Douglas W. Hubbard advocates for a more scientific and quantitative approach to risk management, emphasizing statistical methods like Monte Carlo simulations.
- Structured Analysis: It is divided into three parts: identifying the crisis in risk management, analyzing why current methods are flawed, and offering practical solutions.
Why should I read The Failure of Risk Management?
- Critical Insights: Hubbard provides a thorough examination of the flaws in popular risk management methods, essential for professionals in the field.
- Practical Solutions: The book offers actionable advice and methodologies to improve risk assessment and management practices.
- Broad Applicability: It is relevant for various industries, including finance, engineering, and project management, making it a valuable resource for a wide audience.
What are the key takeaways of The Failure of Risk Management?
- Ineffectiveness of Qualitative Methods: Hubbard argues that qualitative scoring methods, such as risk matrices, often add error and do not improve decision-making.
- Need for Quantitative Analysis: The book advocates for using quantitative methods to better assess risks and make informed decisions.
- Calibration of Expert Judgment: It emphasizes the importance of calibrating expert estimates to improve accuracy in risk assessments.
What are the best quotes from The Failure of Risk Management and what do they mean?
- “First, do no harm.”: This principle should guide risk management practices, ensuring that methods do not introduce additional errors or worsen decision-making.
- “Never attribute to malice that which can be adequately explained by stupidity.”: This highlights the importance of understanding systemic issues rather than blaming individuals for failures.
- “Experience is inevitable. Learning is not.”: This underscores the need for organizations to actively learn from past experiences rather than relying solely on historical knowledge.
What are the “Four Horsemen” of risk management mentioned in The Failure of Risk Management?
- Actuaries: They use scientific and mathematical methods primarily in insurance and pensions, but their influence is limited outside these areas.
- War Quants: Engineers and scientists who apply probabilistic risk analysis and decision analysis, often using simulations to model risks.
- Economists: Financial analysts who focus on statistical analysis of historical data, but may overlook systemic risks and interactions.
- Management Consultants: They often promote qualitative methods that lack empirical support, leading to widespread adoption of ineffective practices.
How does Douglas W. Hubbard define risk in The Failure of Risk Management?
- Long Definition: Risk is described as “a potential loss, disaster, or other undesirable event measured with probabilities assigned to losses of various magnitudes.”
- Short Definition: It is simply “the possibility that something bad could happen.”
- Emphasis on Measurement: Hubbard stresses that risk should be quantifiable, allowing for better decision-making and management.
What is the significance of calibration in risk management according to The Failure of Risk Management?
- Improving Accuracy: Calibration helps experts provide more accurate probability estimates, reducing overconfidence and inconsistency in risk assessments.
- Training Methods: Hubbard discusses techniques for training experts to improve their judgment, which can lead to better decision-making outcomes.
- Empirical Evidence: The book presents research showing that calibrated experts perform significantly better than uncalibrated ones, making calibration a critical component of effective risk management.
What are the problems with popular risk assessment methods discussed in The Failure of Risk Management?
- Qualitative Scoring Issues: Methods like risk matrices often rely on arbitrary scales that compress a wide range of values into limited categories, leading to imprecision.
- Subjective Errors: These methods do not account for known biases and errors in human judgment, which can result in systematic underestimation of risks.
- Lack of Empirical Support: Many popular methods lack scientific validation, making them unreliable for making significant decisions.
How does Douglas W. Hubbard propose to fix the issues in risk management?
- Adopt Quantitative Methods: He advocates for the use of quantitative analysis, such as Monte Carlo simulations, to better assess risks and inform decisions.
- Improve Expert Input: The book suggests methods for calibrating expert judgments and using empirical data to enhance risk assessments.
- Establish Clear Risk Tolerance: Hubbard emphasizes the need for organizations to define their risk tolerance quantitatively, allowing for better resource allocation in risk mitigation.
What is the “one-for-one substitution model” introduced in The Failure of Risk Management?
- Simple Quantitative Model: This model replaces qualitative risk matrices with a straightforward quantitative approach that uses explicit probabilities for likelihood and impact.
- Expert Input: Experts provide subjective estimates of probabilities and potential losses, which are then used in a Monte Carlo simulation to generate a loss exceedance curve.
- Decision Support: The model aims to support decision-making by allowing organizations to visualize risks and compare them against their risk tolerance.
What are the limitations of expert knowledge in risk management according to The Failure of Risk Management?
- Overconfidence: Experts often overestimate their accuracy in predicting outcomes, leading to systematic errors in risk assessments.
- Inconsistency: Experts may provide different estimates for the same risk at different times, indicating a lack of reliability in their judgments.
- Need for Calibration: The book stresses the importance of calibrating expert estimates to improve their accuracy and reduce the impact of biases on decision-making.
How does The Failure of Risk Management address the concept of "calibration"?
- Calibration Importance: Calibration is presented as a critical component of effective risk analysis. Hubbard explains that calibrated estimates lead to more accurate assessments of uncertainty and risk.
- Methods for Calibration: The book outlines various methods for calibrating expert estimates, including the use of equivalent bets and structured reflection on potential errors.
- Impact on Decision-Making: Improved calibration can significantly enhance the quality of risk assessments, leading to better-informed decisions. Hubbard emphasizes that organizations should prioritize calibration training for their experts.
समीक्षाएं
जोखिम प्रबंधन की विफलता को मिश्रित समीक्षाएँ मिली हैं, जिसमें कई लोग इसके पारंपरिक जोखिम प्रबंधन विधियों की आलोचना और मात्रात्मक दृष्टिकोणों के समर्थन की सराहना कर रहे हैं। पाठक हबर्ड की उन अंतर्दृष्टियों की प्रशंसा करते हैं जो गुणात्मक जोखिम आकलनों की सीमाओं को उजागर करती हैं और अधिक कठोर, डेटा-आधारित विधियों की आवश्यकता पर जोर देती हैं। हालांकि, कुछ पाठकों को यह पुस्तक दोहरावदार और अन्य दृष्टिकोणों की अत्यधिक आलोचना करने वाली लगती है। आलोचकों का कहना है कि जबकि हबर्ड प्रभावी रूप से जोखिम प्रबंधन में समस्याओं को उजागर करते हैं, वे व्यावहारिक समाधानों की सीमित पेशकश करते हैं। कुल मिलाकर, यह पुस्तक जोखिम प्रबंधन और निर्णय लेने की भूमिकाओं में पेशेवरों के लिए विचारोत्तेजक मानी जाती है।
Similar Books
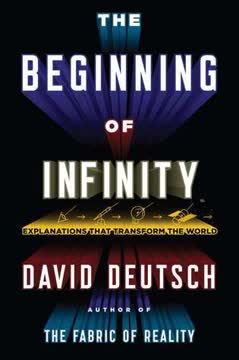
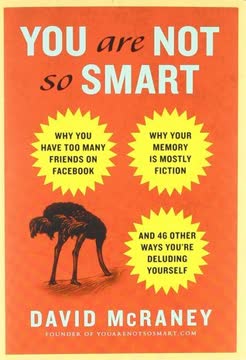
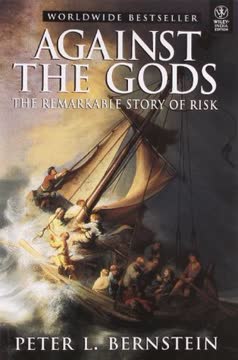
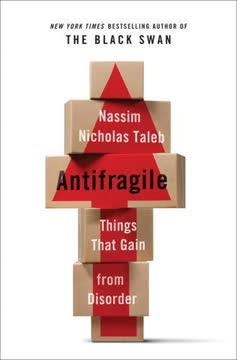
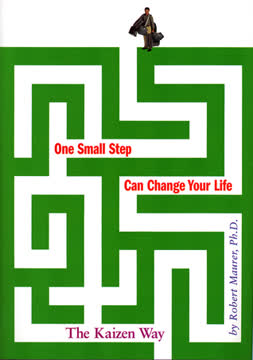
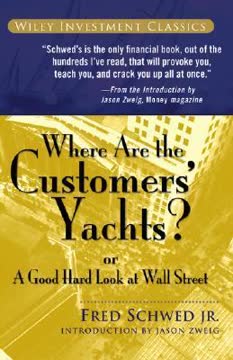
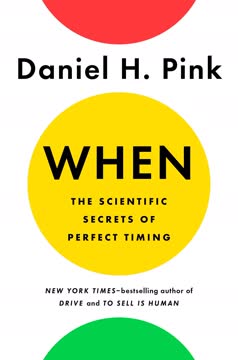
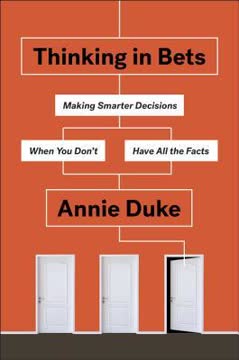
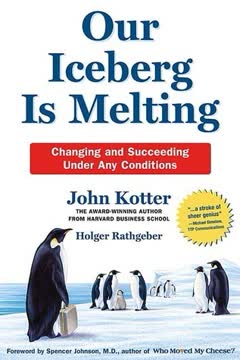
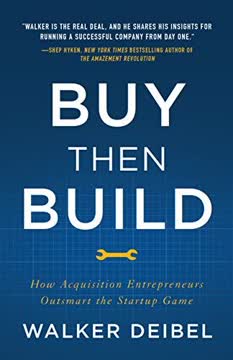