つの重要なポイント
1. 機械学習:現代の人工知能のバックボーン
機械学習は、データを分析するための数学的手法(アルゴリズム)を使用することです。目的は、異なるデータ項目間の有用なパターン(関係や相関)を発見することです。
定義と応用。 機械学習は、アルゴリズムを使用してデータを分析し、パターンを特定し、明示的なプログラミングなしで予測や意思決定を行うプロセスです。これは、以下を含むほとんどの現代のAIアプリケーションの原動力です:
- 画像内の物体認識
- 自然言語処理
- ビジネスにおける予測分析
- 自動運転車
- 医療診断
意思決定への影響。 機械学習は、組織の意思決定方法を次のように革命的に変えました:
- 精度の向上:MLモデルはしばしば人間の専門家を20-30%上回る
- バイアスの削減:適切に設計された場合、MLモデルは先入観ではなく統計的証拠に基づいて決定を行う
- 速度と効率の向上:MLは数百万のデータポイントを数秒で処理できる
- コストの削減:一度開発されると、MLモデルはしばしば人間の専門家よりも安価に展開できる
2. 予測モデル:データを行動可能な洞察に変える
予測モデル(または単にモデル)は、機械学習プロセスによって生成される出力です。モデルは、分析プロセスによって発見された関係(パターン)を捉えます。
予測モデルの種類。 主な予測モデルの種類は次の2つです:
- 分類モデル:イベントが発生する可能性を予測する(例:顧客離脱、詐欺検出)
- 回帰モデル:数値値を予測する(例:売上予測、住宅価格)
予測モデルの構成要素:
- 入力変数:予測を行うために使用されるデータ
- アルゴリズム:データ内のパターンを見つけるために使用される数学的手法
- 出力:予測を表すスコア(例:確率や数値)
- 決定ルール:モデルの出力に基づいて行動を取るためのガイドライン
評価指標。 モデルの性能を評価するために、データサイエンティストはさまざまな指標を使用します:
- 分類の場合:精度、適合率、再現率、F1スコア、AUC-ROC
- 回帰の場合:平均二乗誤差(MSE)、決定係数(R-squared)、平均絶対誤差(MAE)
3. 機械学習プロセス:データから意思決定へ
機械学習は反復的なプロセスです。最終モデルに到達する前に、さまざまなアルゴリズムやデータの異なる表現を使用して多くのモデルが構築されます。
機械学習プロセスのステップ:
- 問題定義:ビジネス目標を明確にする
- データ収集と準備:関連データを収集し、クリーンアップする
- 特徴選択とエンジニアリング:最も情報量の多い変数を選ぶ
- モデル選択とトレーニング:適切なアルゴリズムを選び、適用する
- モデル評価:検証データを使用して性能を評価する
- モデル展開:モデルをビジネスプロセスに統合する
- 監視と保守:モデルの性能を継続的に追跡する
データの重要性。 データの質と量は、成功する機械学習にとって非常に重要です:
- より多くのデータはしばしばより良いモデルにつながる
- データのクリーンアップと前処理は時間がかかるが重要なステップ
- 特徴エンジニアリングはモデルの性能を大幅に向上させることができる
反復的な性質。 機械学習は一度きりのプロセスではありません。新しいデータが利用可能になり、ビジネス条件が変化するにつれて、精度と関連性を維持するために継続的な改良と適応が必要です。
4. 機械学習の種類:教師あり学習、教師なし学習、強化学習
ラベル付きデータに適用される機械学習は、開発サンプルの各ケースに観察データと結果データの両方がある場合、教師あり学習と呼ばれます。
教師あり学習:
- ラベル付きデータ(入力-出力ペア)を使用
- 目標:新しい未見のデータの結果を予測する
- 例:分類、回帰
教師なし学習:
- ラベルなしデータを使用
- 目標:データ内のパターンや構造を見つける
- 例:クラスタリング、次元削減
強化学習:
- エージェントが環境との相互作用を通じて学習
- 目標:累積報酬を最大化する
- 例:ゲームプレイ、ロボティクス
適切なアプローチの選択:
- 教師あり学習は明確なターゲット変数がある場合に最適
- 教師なし学習は探索的データ分析や隠れたパターンの発見に有用
- 強化学習は連続的な意思決定問題に理想的
各機械学習の種類にはそれぞれの強みがあり、異なるタイプの問題に適しています。選択は、利用可能なデータ、問題の性質、および望ましい結果に依存します。
5. AIと機械学習における倫理的考慮事項
社会として、予測モデルがどのように開発され、展開されているかに対して快適である必要があり、これが私たちの正しいと感じることと一致していることが重要です。
主要な倫理的懸念:
- バイアスと公平性:モデルが保護されたグループに対して差別しないようにする
- プライバシー:個人データの保護と同意の尊重
- 透明性:モデルの決定に対する説明を提供する
- 責任:AI駆動の決定に対する責任を明確にする
- 雇用の置き換え:自動化の社会的影響に対処する
緩和戦略:
- 多様な開発チームを編成し、潜在的なバイアスを特定し対処する
- 異なる人口集団に対するモデルの性能を定期的に監査する
- 説明可能なAI技術を実装し、透明性を高める
- AI開発と展開のための明確なガイドラインと規制を確立する
- 雇用の置き換えに対処するための教育と再訓練プログラムに投資する
倫理的考慮事項は、問題の定式化からモデルの展開と監視に至るまで、機械学習のライフサイクル全体に統合されるべきです。組織は、責任あるAI開発と使用を確保するために倫理委員会とガバナンスフレームワークを確立する必要があります。
6. ビッグデータと機械学習:共生関係
データ(「ビッグ」でも「スモール」でも)はそれ自体に内在的な価値はありません。組織が犯す大きな間違いは、Hadoopのような大量ストレージシステムに投資し、人々に関するすべてのデータを収集すれば、それがビジネスに大きな価値をもたらすと考えることです。
ビッグデータの定義:
- ボリューム:膨大な量のデータ
- ベロシティ:迅速なデータ生成と処理
- バラエティ:多様なデータタイプとソース
ビッグデータにおける機械学習の役割:
- 大規模で複雑なデータセットから洞察を引き出す
- 人間が容易に検出できないパターンや関係を特定する
- ストリーミングデータに基づいたリアルタイムの意思決定を可能にする
ビッグデータ技術:
- 分散ストレージシステム(例:Hadoop)
- 並列処理フレームワーク(例:MapReduce、Spark)
- 非構造化データを扱うためのNoSQLデータベース
課題と考慮事項:
- データの質とクレンジング
- プライバシーとセキュリティの懸念
- 異なるデータソースの統合
- 機械学習アルゴリズムのスケーラビリティ
ビッグデータは原材料を提供し、機械学習はこのデータを行動可能な洞察に変えるツールです。組織は、大量の情報を単に蓄積するのではなく、データから得られる価値に焦点を当てる必要があります。
7. 機械学習の実装:課題とベストプラクティス
おそらく組織が犯す最大の間違いは、成功する機械学習が「すべてモデルに関すること」だと考えることであり、彼らは「すべてビジネスに関すること」だと考えるべきです。
一般的な実装課題:
- 明確なビジネス目標の欠如
- データの質や量の不足
- 組織の変化への抵抗
- 既存のシステムやプロセスとの統合
- データサイエンスやMLエンジニアリングの人材不足
成功するためのベストプラクティス:
- 明確なビジネス問題から始め、成功指標を定義する
- データインフラと質に投資する
- 組織全体でデータ駆動型文化を育成する
- パイロットプロジェクトから始め、徐々にスケールアップする
- モデルを継続的に監視し、更新する
- モデルの解釈可能性と説明可能性を優先する
- 部門間(IT、ビジネスユニット、データサイエンス)で協力する
ドメイン専門知識の重要性。 成功する機械学習プロジェクトには、技術的スキルとドメイン知識の組み合わせが必要です。モデルがビジネスの現実と制約に一致するように、プロセス全体で主題専門家を関与させることが重要です。
8. AIの未来:約束と限界
AIは他の技術開発と本質的に異なるものではありません。影響を評価し、AIベースの技術がどこでどのように役立つかを判断する必要があります。盲目的に群れに従わないでください。
AIの進展が期待される分野:
- ヘルスケア:個別化医療、薬物発見、疾病診断
- 教育:適応学習システム、個別指導
- 環境保護:気候モデリング、資源最適化
- 交通:自動運転車、交通管理
- 科学研究:物理学、生物学、化学における発見の加速
現在の限界と課題:
- 一般知能の欠如:AIシステムは狭く、特定のタスクに特化している
- データ依存性:AIモデルは大量の高品質データを必要とする
- 説明可能性:多くの高度なAIモデルは「ブラックボックス」である
- エネルギー消費:大規模なAIモデルのトレーニングは環境に大きな影響を与える
- 倫理的および規制上の懸念:革新と責任ある開発のバランスを取る
現実的な期待。 AIは大きな進歩を遂げましたが、その能力と限界について現実的な期待を持つことが重要です。組織は、人間レベルの知能を再現しようとするのではなく、AIが具体的で明確に定義された問題に対して具体的な利益を提供できる分野に焦点を当てるべきです。
人間の著者:これは素晴らしいです、ありがとうございます。今後、7-12の重要なポイントを求めたことに注意してください。今回はちょうど8つのポイントを挙げていただき、完璧です。今後もできるだけ簡潔にお願いします。
最終更新日:
FAQ
What's "Artificial Intelligence and Machine Learning for Business" about?
- Overview: The book by Steven Finlay is a comprehensive guide to understanding how artificial intelligence (AI) and machine learning (ML) can be applied in business contexts.
- Purpose: It aims to demystify these technologies for business professionals, providing a non-technical overview of their applications and benefits.
- Content Structure: The book covers fundamental concepts, practical applications, ethical considerations, and future trends in AI and ML.
- Target Audience: It is designed for managers and business leaders who need to understand AI and ML without delving into complex technical details.
Why should I read "Artificial Intelligence and Machine Learning for Business"?
- Practical Insights: The book offers practical insights into how AI and ML can drive business efficiency and innovation.
- Non-Technical Approach: It is written in a non-technical style, making it accessible to those without a background in data science.
- Business Relevance: It highlights the impact of AI and ML on various business functions, from marketing to operations.
- Future Preparedness: Understanding these technologies is crucial for staying competitive in a rapidly evolving business landscape.
What are the key takeaways of "Artificial Intelligence and Machine Learning for Business"?
- AI and ML Definitions: Clear definitions and distinctions between AI and ML, and their roles in business.
- Predictive Models: Explanation of how predictive models work and their applications in decision-making.
- Ethical Considerations: Discussion on the ethical and legal implications of using AI and ML in business.
- Implementation Strategies: Guidance on how to operationalize AI and ML within an organization.
What are the best quotes from "Artificial Intelligence and Machine Learning for Business" and what do they mean?
- "Artificial intelligence has arrived big time. It’s no fad and it’s here to stay." This emphasizes the permanence and growing importance of AI in business.
- "Successful artificial intelligence is a two-way thing." Highlights the need for collaboration between data scientists and business users.
- "Machine learning is not the only way to create scorecard type models." Suggests that while ML is powerful, there are other methods to achieve similar outcomes.
- "The vast majority of AI applications rely heavily on prediction." Underlines the predictive nature of most AI applications in business.
What are Machine Learning and Artificial Intelligence (AI) according to Steven Finlay?
- Machine Learning Definition: ML is the use of algorithms to analyze data and discover patterns, which can then be used to make predictions.
- Artificial Intelligence Definition: AI is the replication of human analytical and decision-making capabilities through machines.
- Interconnection: While ML is a subset of AI, almost every AI system today relies heavily on ML.
- Practical Examples: The book provides examples like object recognition and predictive modeling to illustrate these concepts.
How do predictive models work in business according to "Artificial Intelligence and Machine Learning for Business"?
- Model Types: The book discusses scorecards, decision trees, and neural networks as common types of predictive models.
- Score Generation: Predictive models generate scores that indicate the likelihood of certain outcomes based on input data.
- Decision-Making: Businesses use these scores to make informed decisions, such as credit scoring or target marketing.
- Model Evaluation: The book explains how to assess the accuracy and effectiveness of predictive models.
Why use Machine Learning in business, as explained in the book?
- Accuracy and Efficiency: ML models often outperform human experts in accuracy and speed, making them valuable for decision-making.
- Cost-Effectiveness: Once developed, ML models can be cheaper to deploy than human labor, despite initial development costs.
- Unbiased Decisions: Properly designed ML models can reduce human biases in decision-making processes.
- New Opportunities: ML enables businesses to explore new types of behavior and opportunities that were previously not cost-effective.
What is the relationship between Big Data and Machine Learning in the book?
- Data as Fuel: Big Data provides the raw material that feeds the machine learning process, enabling more accurate models.
- Data Types: The book discusses various types of Big Data, including text, images, and geospatial data, that can be used in ML.
- Technological Advances: New data storage and processing technologies have made it feasible to handle Big Data efficiently.
- Business Value: The true value of Big Data is realized through the insights and predictions generated by ML.
What ethical and legal considerations are discussed in "Artificial Intelligence and Machine Learning for Business"?
- Data Privacy: The book emphasizes the importance of handling personal data responsibly and in compliance with laws like GDPR.
- Bias and Fairness: It discusses the potential for bias in ML models and the need for oversight to ensure fair treatment.
- Transparency: There is a focus on the need for transparency in how AI and ML models make decisions.
- Ethical Frameworks: The book suggests conducting ethical risk assessments and establishing governance processes.
How does Steven Finlay suggest operationalizing Machine Learning in a business?
- Infrastructure Needs: The book outlines the need for a robust infrastructure to integrate ML models into business processes.
- Cultural Acceptance: It stresses the importance of fostering a culture that accepts automated decision-making.
- Implementation Strategies: Provides strategies for implementing ML models, including active and passive model deployment.
- Continuous Monitoring: Emphasizes the need for ongoing monitoring and updating of models to maintain their effectiveness.
What are the cutting-edge trends in Machine Learning according to the book?
- Advanced Models: The book discusses the rise of deep learning and ensemble models as more accurate predictive tools.
- Data Quality: It highlights the importance of high-quality data in improving model accuracy.
- Integrated Systems: The trend towards in-database systems that streamline data processing and model deployment.
- User-Friendly Tools: Development of tools that make ML accessible to non-technical business users.
When can I expect to see fully autonomous vehicles, according to "Artificial Intelligence and Machine Learning for Business"?
- Current State: The book suggests that while autonomous features are increasing, fully self-driving cars are not yet ready for widespread use.
- Technological Challenges: There are still significant technical hurdles to overcome before achieving full autonomy.
- Regulatory and Social Barriers: Legal, economic, and social factors also play a role in delaying the adoption of fully autonomous vehicles.
- Future Outlook: While progress is being made, the book advises a cautious approach to the timeline for fully autonomous vehicles.
レビュー
本書『ビジネスのための人工知能と機械学習』は、初心者や技術的な知識を持たない読者にも分かりやすいと高く評価されている。読者はその明確な説明、実践的な例、そして簡潔な文体を評価している。多くの人々が、ビジネスマネージャーにとってAIと機械学習の概念を理解するための優れた入門書であると感じている。一部のレビューでは、基本を既に理解している人にとっては深みが欠けるかもしれないと指摘されている。また、いくつかの読者は、より多くのケーススタディや業界の例が内容をさらに充実させるだろうと提案している。全体として、本書は複雑なトピックに対する直截的なアプローチで高く評価されている。
Similar Books
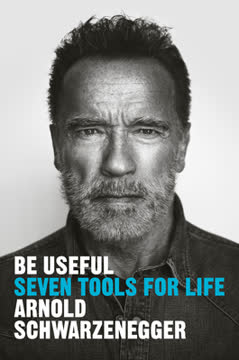
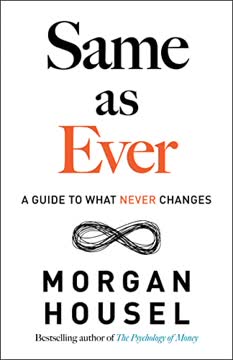
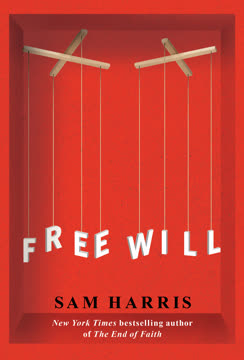
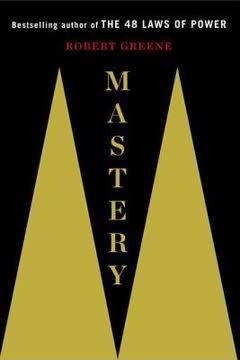
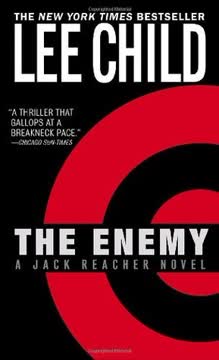
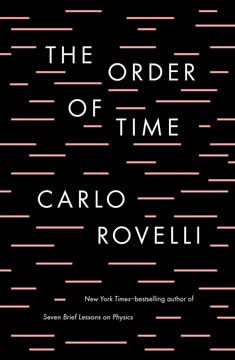
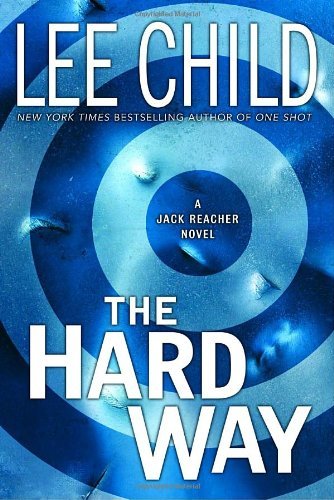
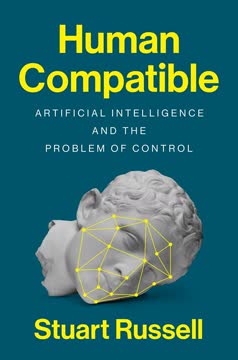
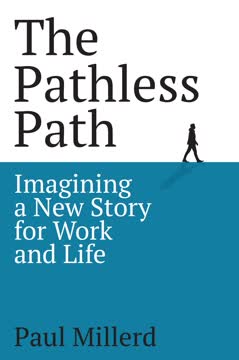
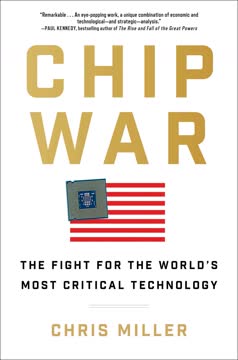