Key Takeaways
1. Embrace Pythonic thinking for cleaner, more effective code
Python programmers prefer to be explicit, to choose simple over complex, and to maximize readability.
Clarity and simplicity: Python's design philosophy emphasizes readability and simplicity. This approach, often called "Pythonic," involves writing code that is clear, concise, and follows established conventions. Some key principles include:
- Using whitespace for indentation to define code blocks
- Favoring built-in functions and data types
- Employing list comprehensions for succinct data manipulation
- Following the PEP 8 style guide for consistent code formatting
Explicit is better than implicit: Python encourages writing code that clearly states its intentions. This means avoiding hidden side effects, using descriptive variable names, and preferring direct expressions over complex ones. For example, instead of using cryptic one-liners, break complex operations into multiple steps with meaningful variable names.
2. Master function design for flexibility and readability
Use keyword arguments with default values to make it easy to add new behaviors to a function, especially when the function has existing callers.
Flexible function signatures: Python offers several ways to create flexible and powerful functions:
- Use *args for variable positional arguments
- Employ **kwargs for variable keyword arguments
- Provide default values for optional parameters
- Utilize keyword-only arguments to enforce clarity
Generator functions: Instead of returning lists, consider using generator functions with the yield keyword. This approach allows for:
- Memory efficiency when dealing with large datasets
- Lazy evaluation of sequences
- Easy creation of iterable objects
By mastering these function design techniques, you can create more versatile and maintainable code that adapts to changing requirements with minimal refactoring.
3. Harness the power of classes and inheritance wisely
Use multiple inheritance only for mix-in utility classes.
Class design principles: When working with object-oriented programming in Python:
- Prefer composition over inheritance for most use cases
- Use abstract base classes to define interfaces
- Implement properties for controlled attribute access
- Utilize class and static methods for alternative constructors and utility functions
Effective inheritance: While Python supports multiple inheritance, use it judiciously:
- Employ mix-in classes for reusable functionality
- Use the super() function to properly initialize parent classes
- Be aware of the method resolution order (MRO) in complex inheritance hierarchies
By following these guidelines, you can create robust and flexible class hierarchies that promote code reuse without introducing unnecessary complexity.
4. Utilize metaclasses and attributes for advanced programming
Use metaclasses to ensure that subclasses are well formed at the time they are defined, before objects of their type are constructed.
Metaclass power: Metaclasses allow you to customize class creation:
- Validate class definitions at runtime
- Automatically register classes in a central registry
- Modify class attributes or methods during creation
Dynamic attributes: Python's flexible attribute system enables powerful techniques:
- Use getattr and setattr for lazy attribute loading
- Implement descriptors for reusable property-like behavior
- Leverage slots for memory optimization in performance-critical code
These advanced features provide fine-grained control over class behavior and attribute access, enabling sophisticated programming patterns and optimizations.
5. Leverage concurrency and parallelism for optimal performance
Python threads can't run bytecode in parallel on multiple CPU cores because of the global interpreter lock (GIL).
Concurrency models: Python offers several approaches to concurrent programming:
- Threads for I/O-bound tasks and maintaining responsiveness
- Multiprocessing for CPU-bound tasks and true parallelism
- Asynchronous programming with asyncio for high-concurrency I/O
Performance considerations: When optimizing Python code:
- Use the multiprocessing module for CPU-intensive tasks
- Leverage the concurrent.futures module for easy parallelism
- Consider Cython or numba for performance-critical sections
By understanding the strengths and limitations of each concurrency model, you can choose the most appropriate approach for your specific use case and achieve optimal performance.
6. Tap into Python's built-in modules for enhanced functionality
Use Python's built-in modules for algorithms and data structures.
Essential built-ins: Python's standard library offers a wealth of functionality:
- collections for specialized container datatypes
- itertools for efficient iteration and combination
- functools for higher-order functions and decorators
- datetime for date and time manipulation
- json for data serialization
Numeric and scientific computing: For advanced mathematical operations:
- Use decimal for precise decimal arithmetic
- Leverage math and statistics for mathematical functions
- Consider numpy and scipy for scientific computing (third-party packages)
By utilizing these built-in modules, you can solve common programming tasks efficiently without reinventing the wheel or relying on external dependencies.
7. Collaborate effectively and maintain production-ready code
The only way to have confidence in a Python program is to write tests.
Testing fundamentals: Implement a comprehensive testing strategy:
- Write unit tests for individual components
- Develop integration tests for system-wide behavior
- Use the unittest module for test case creation
- Consider pytest or nose for advanced testing features
Deployment best practices: Ensure your code is production-ready:
- Use virtual environments for dependency isolation
- Implement logging for effective debugging and monitoring
- Utilize configuration files for environment-specific settings
- Employ continuous integration and deployment (CI/CD) pipelines
By prioritizing testing and following deployment best practices, you can create reliable, maintainable Python applications that perform well in production environments and facilitate effective collaboration among team members.
Last updated:
FAQ
What's Effective Python: 59 Specific Ways to Write Better Python about?
- Pythonic Practices: The book by Brett Slatkin focuses on writing Pythonic code, which is clear, concise, and leverages Python's unique features.
- Intermediate Audience: It targets intermediate Python programmers looking to deepen their understanding and improve their coding practices.
- Practical Advice: Each of the 59 items offers practical guidance, often with code examples, to help readers apply concepts in their projects.
Why should I read Effective Python?
- Enhance Code Quality: The book teaches best practices and idiomatic usage, leading to more maintainable and efficient code.
- Learn from Experience: Brett Slatkin shares insights from his experience at Google, providing valuable lessons from real-world applications.
- Structured Learning: Organized into clear, digestible items, it allows readers to learn at their own pace and focus on specific areas of interest.
What are the key takeaways of Effective Python?
- Pythonic Thinking: Emphasizes writing code that adheres to Python's design philosophy, valuing readability and simplicity.
- Built-in Features: Encourages effective use of Python's built-in features and libraries, such as list comprehensions and understanding data types.
- Code Organization: Highlights organizing code with classes, functions, and modules for clean and maintainable codebases.
What are some specific methods or advice from Effective Python?
- List Comprehensions: Advises using list comprehensions over map and filter for clearer, more Pythonic code.
- Exceptions Over None: Suggests using exceptions for error handling instead of returning None to avoid ambiguity.
- @property Decorators: Discusses using @property decorators for managing attribute access and validation within classes.
How does Effective Python address Python 2 and Python 3 differences?
- Version Awareness: Provides guidance for both Python 2 and Python 3, crucial for developers in mixed environments.
- Specific Examples: Illustrates differences in syntax and behavior, such as string handling and print statements.
- Encouragement for Python 3: Strongly encourages adopting Python 3 for new projects due to its improvements and features.
What are some common pitfalls to avoid according to Effective Python?
- Complex Expressions: Warns against writing overly complex expressions in a single line, advocating for simpler logic.
- Private Attributes: Advises against heavy reliance on private attributes, preferring public attributes with clear documentation.
- Neglecting Documentation: Emphasizes the importance of clear documentation for functions, classes, and modules.
How does Effective Python suggest improving function design?
- Keyword Arguments: Recommends using keyword arguments for clarity and flexibility, avoiding positional argument confusion.
- Exceptions for Errors: Suggests raising exceptions for error conditions instead of returning None for clearer intent.
- Helper Functions: Encourages breaking down complex functions into smaller, more manageable helper functions.
What role do classes and inheritance play in Effective Python?
- Encapsulation: Discusses how classes encapsulate behavior and state, aiding in managing complex systems.
- Polymorphism and Inheritance: Emphasizes using these concepts for flexible and extensible code structures.
- Mix-ins: Introduces mix-ins for adding functionality without the complications of multiple inheritance.
How does Effective Python recommend handling attributes and properties?
- Plain Attributes: Advises using plain attributes for simplicity and clarity, aligning with Python's philosophy.
- @property for Validation: Recommends using @property for managing attribute access and enforcing rules.
- Avoid Side Effects: Cautions against side effects in property methods to maintain predictability.
How does Effective Python address performance optimization?
- Profiling First: Advises profiling code with tools like cProfile before optimizing to focus efforts effectively.
- Memory Management: Introduces tracemalloc for tracking memory usage and identifying leaks.
- Built-in Algorithms: Encourages using Python's built-in modules for efficient algorithms and data structures.
What are some advanced concepts covered in Effective Python?
- Metaclasses and Decorators: Delves into metaclasses for class manipulation and decorators for modifying function behavior.
- Concurrency with Asyncio: Discusses asyncio for writing concurrent code using async/await syntax.
- Custom Container Types: Explains creating custom container types by inheriting from collections.abc.
What are the best quotes from Effective Python and what do they mean?
- "Readability counts.": Emphasizes writing code that is easy to read and understand, crucial for collaboration and maintenance.
- "Explicit is better than implicit.": Reflects Python's design philosophy, encouraging clear and explicit code to reduce ambiguity.
- "There should be one—and preferably only one—obvious way to do it.": Advocates for simplicity and clarity, promoting standard practices and conventions.
Review Summary
Effective Python is highly praised for its concise, practical approach to improving Python skills. Readers appreciate its coverage of intermediate to advanced topics, clear explanations, and real-world examples. The book is recommended for experienced developers looking to write more Pythonic code. Some reviewers note that certain sections may be challenging for beginners or outdated due to Python's evolution. Overall, it's considered a valuable resource for Python programmers seeking to enhance their understanding and efficiency with the language.
Similar Books
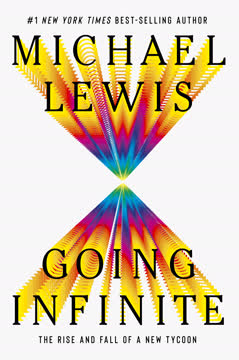
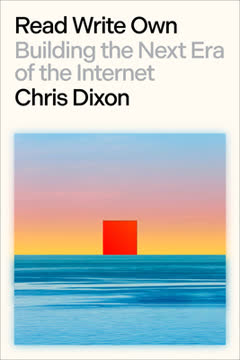
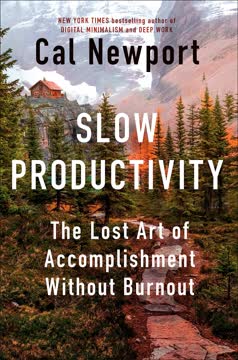
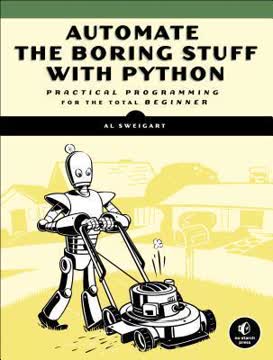

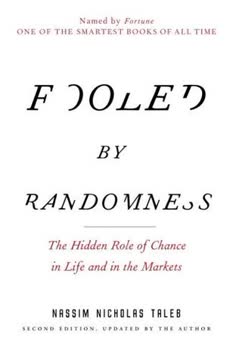
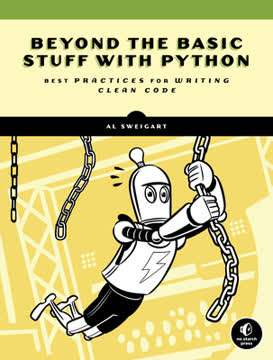
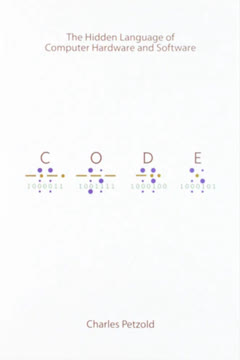
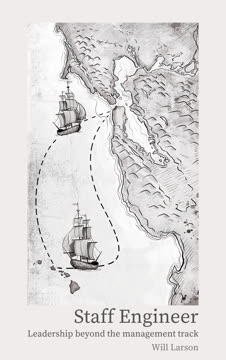
Download PDF
Download EPUB
.epub
digital book format is ideal for reading ebooks on phones, tablets, and e-readers.