Key Takeaways
1. Statistics can be manipulated to mislead: Be aware of biased samples
"The secret language of statistics, so appealing in a fact-minded culture, is employed to sensationalize, inflate, confuse, and oversimplify."
Biased samples skew results. Surveys and studies often rely on samples that are not representative of the entire population. For example, a study on the income of Yale graduates may only include those who responded to a survey, potentially excluding less successful alumni who are unwilling to report their earnings. This can lead to inflated average income figures that do not accurately represent the entire graduating class.
Self-selection introduces bias. When participants choose whether to participate in a study, it can create a biased sample. For instance, people who are more satisfied with a product may be more likely to respond to a customer satisfaction survey, leading to overly positive results. To combat this, be wary of studies with low response rates or those that don't disclose their methodology.
Size matters in sampling. Small sample sizes can lead to unreliable conclusions. A study based on just a handful of cases may produce results that are not statistically significant or representative of the larger population. Always look for information on sample size and statistical significance when evaluating claims based on data.
2. Averages can deceive: Understand mean, median, and mode
"There are three kinds of averages: the mean, median, and mode. The same set of figures can be used to demonstrate at least three different averages."
Different averages tell different stories. The mean (arithmetic average), median (middle value), and mode (most frequent value) can each paint a different picture of the same data set. For example:
- Mean income in a community can be significantly skewed by a few high earners
- Median income often provides a more accurate representation of typical earnings
- Mode can highlight the most common income level
Choose the right average for context. When presented with an average, always ask which type is being used and why. For income data, the median is often more informative than the mean, as it's less affected by extreme values. In contrast, the mean might be more appropriate for data sets with less variation.
Beware of unspecified averages. When an "average" is presented without specifying the type, it's often chosen to support a particular narrative. Always be skeptical of unqualified averages and seek additional context to understand the full picture of the data.
3. Graphs and visual representations can distort reality
"The Gee-Whiz Graph...is employed to give the impression of important change where there is relatively little."
Truncated scales exaggerate changes. Graphs that don't start at zero can make small differences appear dramatic. For example, a graph showing stock prices over a short period might use a scale starting at 95 instead of 0, making a 5% change look like a 100% increase.
Manipulating proportions distorts comparisons. Two-dimensional or three-dimensional images used to represent one-dimensional data can create false impressions. For instance, doubling the height of a symbol might quadruple its area, exaggerating the difference it represents.
Visual tricks to watch for:
- Missing or inconsistent scale labels
- Changing the aspect ratio to steepen or flatten trend lines
- Using isometric projections to make later data points appear larger
- Inconsistent intervals on time-based axes
Always examine the scales and proportions carefully when interpreting visual data representations.
4. Correlation does not imply causation: Beware of false conclusions
"If B follows A, then A has caused B. An unwarranted assumption is being made that since smoking and low grades go together, smoking causes low grades."
Post hoc fallacy is pervasive. Many statistical claims fall into the trap of assuming that because one event followed another, the first event caused the second. This reasoning is flawed and can lead to incorrect conclusions.
Examples of false causation:
- Ice cream sales correlate with increased crime rates (both actually increase due to warmer weather)
- Countries with higher chocolate consumption have more Nobel laureates (likely due to other factors like wealth and education)
Consider alternative explanations. When presented with a correlation, always consider other factors that might explain the relationship. Look for:
- Common causes that affect both variables
- Reverse causation (B might cause A)
- Coincidental relationships, especially in small sample sizes
Demand evidence of causation. Proper scientific studies use controlled experiments and statistical techniques to establish causation. Be skeptical of causal claims based solely on observational data or correlations.
5. Percentages and ratios can be misleading when context is missing
"Percentages offer a fertile field for confusion."
Percent of what? Always ask what the base or denominator is when presented with a percentage. A 50% increase can mean very different things depending on the starting point. For example:
- A 50% increase in a small number (e.g., from 2 to 3) may be insignificant
- A 50% increase in a large number (e.g., from 1 million to 1.5 million) can be substantial
Percentage points vs. percentages. Be clear about the difference between percentage points and percentages. An increase from 2% to 4% is:
- A 2 percentage point increase
- A 100% increase
Ratios need context. When comparing ratios or rates, ensure you understand the scale and context. For instance, a doubling of a rare event might still result in a very small absolute number.
Always seek the absolute numbers behind percentages and ratios to gain a clearer understanding of their significance.
6. Beware of the "post hoc" fallacy in statistical interpretations
"To call some one of these the cause of some other is manifestly silly. But it is done every day."
Temporal sequence ≠ causation. The post hoc fallacy assumes that because one event followed another, the first event caused the second. This reasoning is flawed and can lead to incorrect conclusions in many fields, including medicine, economics, and social sciences.
Examples of post hoc fallacy:
- A politician takes office, and the economy improves (ignoring long-term economic cycles)
- A patient takes a new supplement and their symptoms improve (not accounting for natural recovery or placebo effect)
Consider multiple factors. Real-world phenomena are often the result of complex interactions between many variables. When evaluating claims of cause and effect:
- Look for controlled studies that account for confounding variables
- Consider alternative explanations and potential hidden factors
- Be skeptical of simple explanations for complex issues
Demand rigorous evidence. Proper scientific studies use techniques like randomized controlled trials and multivariate analysis to establish causation. Be wary of causal claims based solely on temporal sequence or correlation, especially in fields with many interacting variables.
7. Critical thinking is essential: Ask who, how, and what's missing
"Not all the statistical information that you may come upon can be tested with the sureness of chemical analysis or of what goes on in an assayer's laboratory. But you can prod the stuff with five simple questions, and by finding the answers avoid learning a remarkable lot that isn't so."
Question the source. Always ask "Who says so?" when presented with statistics. Consider potential biases:
- Is the source an interested party?
- Do they have a reputation to uphold or a product to sell?
- Are they using an "O.K. name" to lend credibility?
Examine the methodology. Ask "How do they know?" to understand the data collection and analysis process:
- Was the sample size adequate?
- Were appropriate statistical tests applied?
- Is the margin of error reported?
Look for missing information. Ask "What's missing?" to identify crucial context:
- Are comparisons provided?
- Is the time frame specified?
- Are raw numbers given alongside percentages?
By consistently applying these critical thinking questions, you can better evaluate the validity and relevance of statistical claims.
8. Numbers without context are meaningless: Always seek comparisons
"Many figures lose meaning because a comparison is missing."
Absolute numbers need context. A large number by itself doesn't tell you much. For example, "1000 deaths" could be tragic or insignificant depending on the population size and time frame.
Essential comparisons to seek:
- Historical data: How does this compare to previous years?
- Population-adjusted rates: Per capita figures often provide better insights
- Relevant benchmarks: How does this compare to similar situations or expectations?
Beware of isolated statistics. When presented with a single statistic, always ask:
- Compared to what?
- Is this a typical value or an outlier?
- What's the trend over time?
Demand relative measures. Whenever possible, look for statistics presented as rates, ratios, or percentages that provide built-in comparisons. These measures often give a clearer picture of the significance of a number in its proper context.
9. Watch for subject changes and irrelevant data in statistical arguments
"One thing is all too often reported as another."
Bait-and-switch tactics. Be alert for subtle changes in what's being measured or reported. Common switches include:
- Reported cases vs. actual incidence of a disease
- Survey responses vs. actual behavior
- Correlation vs. causation
Irrelevant data distracts. Watch for statistics that seem impressive but don't actually support the argument being made. Examples:
- Using total numbers instead of per capita rates when comparing populations of different sizes
- Citing laboratory results that don't translate to real-world effectiveness
Stay focused on the claim. When evaluating a statistical argument:
- Identify the main claim being made
- Assess whether each piece of data directly supports that claim
- Be wary of tangential information that may distract from weak central arguments
By maintaining focus on the core issue and scrutinizing the relevance of each data point, you can avoid being misled by cleverly presented but ultimately irrelevant statistics.
10. Develop statistical literacy to make informed decisions
"It is rather like the conviction among the people of the New Hebrides that body lice produce good health. Observation over the centuries had taught them that people in good health usually had lice and sick people very often did not."
Statistical literacy is crucial. In our data-driven world, the ability to critically evaluate statistical claims is essential for making informed decisions in personal, professional, and civic life.
Key skills to develop:
- Understanding basic statistical concepts (e.g., averages, sampling, correlation)
- Recognizing common statistical fallacies and manipulations
- Asking critical questions about data sources and methodologies
Apply skepticism consistently. Treat all statistical claims with a healthy dose of skepticism, regardless of whether they align with your preexisting beliefs. This balanced approach helps avoid confirmation bias and leads to more objective decision-making.
Seek multiple perspectives. When faced with important decisions based on statistical information:
- Consult diverse sources
- Look for expert interpretations and critiques
- Consider the limitations and uncertainties in the data
By developing these skills and habits, you can navigate the sea of statistics more confidently and make better-informed choices in all aspects of life.
Last updated:
FAQ
What's "How to Lie with Statistics" about?
- Overview: "How to Lie with Statistics" by Darrell Huff is a guide to understanding how statistics can be manipulated to mislead or deceive.
- Purpose: The book aims to educate readers on the common tricks used in presenting statistical data that can distort the truth.
- Content: It covers various statistical methods and how they can be used to create false impressions, from biased samples to misleading graphs.
- Illustrations: The book is illustrated by Irving Geis, which helps in visualizing the concepts discussed.
Why should I read "How to Lie with Statistics"?
- Critical Thinking: It enhances your ability to critically analyze statistical information presented in media, advertisements, and research.
- Awareness: The book raises awareness about the potential misuse of statistics in everyday life.
- Practical Examples: It provides practical examples and anecdotes that make the concepts relatable and easier to understand.
- Self-defense: Reading it equips you with the knowledge to defend against being misled by statistical manipulation.
What are the key takeaways of "How to Lie with Statistics"?
- Bias in Sampling: Be wary of biased samples that do not represent the whole population accurately.
- Misleading Averages: Understand the difference between mean, median, and mode, and how they can be used to mislead.
- Graphical Deception: Recognize how graphs can be manipulated to exaggerate or minimize trends.
- Correlation vs. Causation: Learn to differentiate between correlation and causation to avoid false conclusions.
What are the best quotes from "How to Lie with Statistics" and what do they mean?
- "There are three kinds of lies: lies, damned lies, and statistics." This quote, attributed to Disraeli, highlights how statistics can be used to deceive.
- "Statistical thinking will one day be as necessary for efficient citizenship as the ability to read and write." H.G. Wells emphasizes the importance of understanding statistics in modern society.
- "Round numbers are always false." Samuel Johnson suggests skepticism towards overly neat figures, as they often oversimplify complex data.
- "It ain’t so much the things we don’t know that get us in trouble. It’s the things we know that ain’t so." Artemus Ward warns against false beliefs, which can be perpetuated by misleading statistics.
How does Darrell Huff explain the concept of "The Sample with the Built-in Bias"?
- Definition: A biased sample is one that does not accurately represent the population it is supposed to reflect.
- Example: Huff uses the example of Yale graduates' income, where only those with known addresses and who responded were included, skewing the results.
- Impact: Such samples can lead to conclusions that are not reflective of the true situation, often exaggerating or minimizing the reality.
- Solution: Ensure samples are random and representative to avoid built-in biases.
What does "The Well-Chosen Average" mean in the context of the book?
- Different Averages: Huff explains the difference between mean, median, and mode, and how each can tell a different story.
- Manipulation: By choosing a specific type of average, one can present data in a way that supports a particular narrative.
- Example: A neighborhood's average income can appear high or low depending on whether the mean or median is used.
- Critical Evaluation: Always question which type of average is being used and why.
How are "The Little Figures That Are Not There" used to mislead?
- Missing Data: Important figures like sample size or error margins are often omitted, leading to misleading conclusions.
- Range and Deviation: Without understanding the range or deviation, an average can be meaningless.
- Example: Housing statistics based on average family size can misrepresent the needs of the population.
- Awareness: Look for missing figures that could change the interpretation of the data.
What is the "Gee-Whiz Graph" and how does it deceive?
- Graph Manipulation: Graphs can be manipulated by truncating axes or changing scales to exaggerate trends.
- Visual Impact: A small change can appear significant if the graph is designed to mislead visually.
- Example: A ten percent increase can look like a dramatic rise if the graph is truncated.
- Critical Viewing: Always check the scales and axes of graphs to understand the true data.
How does "The One-Dimensional Picture" mislead readers?
- Pictorial Graphs: These use images to represent data, which can exaggerate differences through size manipulation.
- Misleading Impressions: A larger image can suggest a much greater difference than the data supports.
- Example: A moneybag representing income can be drawn larger to imply a bigger disparity.
- Skepticism: Be cautious of pictorial representations that may distort the actual data.
What is the "Semiattached Figure" and how is it used?
- Definition: This involves using a figure that is related but not directly relevant to the claim being made.
- Example: Claiming a product kills germs in a test tube to imply it cures colds in humans.
- Misleading Correlation: It creates a false sense of causation or relevance.
- Critical Questioning: Always ask how the figure is related to the claim being made.
How does the book address the fallacy of "Post Hoc"?
- Post Hoc Fallacy: Assuming that because one event follows another, the first caused the second.
- Example: Correlating smoking with low grades without considering other factors.
- Multiple Explanations: There may be other factors causing both events, or the relationship may be coincidental.
- Skeptical Analysis: Always consider alternative explanations for correlations.
How can readers "Talk Back to a Statistic" according to Darrell Huff?
- Question the Source: Consider who is presenting the statistic and their potential biases.
- Check the Methodology: Look into how the data was collected and whether the sample was representative.
- Look for Missing Information: Identify any missing data that could alter the interpretation.
- Evaluate the Logic: Ensure the conclusions drawn from the data make logical sense and are not based on faulty assumptions.
Review Summary
How to Lie with Statistics is widely praised as a timeless, accessible guide to understanding and critically evaluating statistical information. Readers appreciate its clear explanations, humor, and practical examples that expose common tricks used to mislead with data. Many consider it essential reading for developing statistical literacy and critical thinking skills. While some find the dated examples charming, others wish for more modern content. Overall, reviewers highly recommend the book for its valuable lessons on interpreting statistics in everyday life.
Similar Books
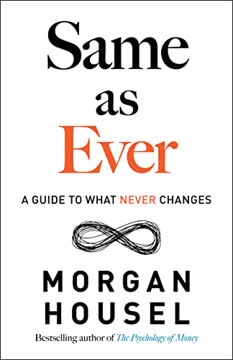
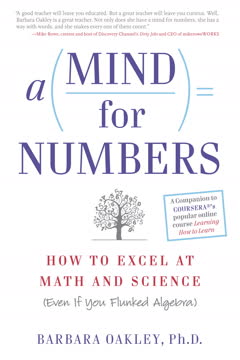
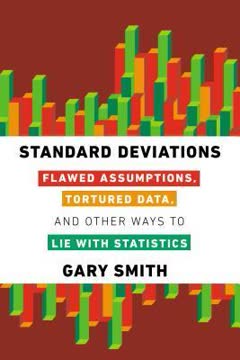
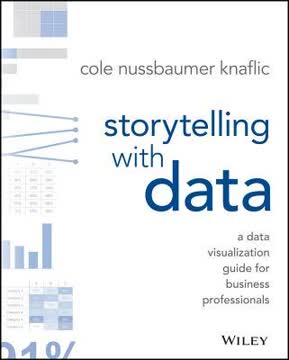
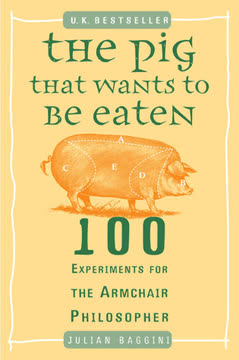
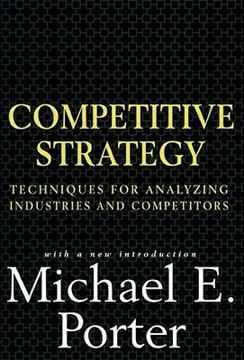
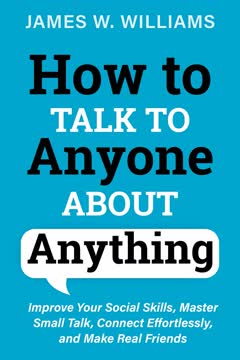
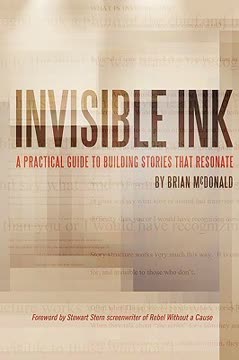
Download PDF
Download EPUB
.epub
digital book format is ideal for reading ebooks on phones, tablets, and e-readers.