Key Takeaways
1. Statistics: The Power to Turn Data into Insight
It's easy to lie with statistics, but it's hard to tell the truth without them.
Data-driven decision making. Statistics provides tools to process and analyze vast amounts of information, transforming raw data into meaningful insights. This power allows us to answer important questions across various fields, from economics to public health.
Overcoming intuition. Statistical analysis often reveals counterintuitive truths, challenging our assumptions and biases. By relying on data and rigorous analysis, we can make more informed decisions and avoid common pitfalls of human judgment.
Ethical considerations. While statistics is a powerful tool, it can be misused or misinterpreted. Understanding statistical concepts helps us critically evaluate claims, spot potential manipulation, and use data responsibly to improve our world.
2. Descriptive Statistics: Summarizing Complex Information
A batting average is a descriptive statistic.
Measures of central tendency. The mean, median, and mode provide different ways to describe the "middle" of a dataset:
- Mean: The average, sensitive to outliers
- Median: The middle value, resistant to outliers
- Mode: The most frequent value
Measures of dispersion. These statistics describe how spread out the data are:
- Range: The difference between the highest and lowest values
- Standard deviation: The average distance from the mean
- Variance: The square of the standard deviation
Visual representations. Graphs and charts can effectively summarize large datasets:
- Histograms: Show the distribution of data
- Box plots: Display median, quartiles, and outliers
- Scatter plots: Illustrate relationships between variables
3. Correlation: Understanding Relationships Between Variables
Correlation does not imply causation.
Strength and direction. The correlation coefficient measures the strength and direction of a linear relationship between two variables, ranging from -1 to 1:
- Positive correlation: As one variable increases, the other tends to increase
- Negative correlation: As one variable increases, the other tends to decrease
- No correlation: No consistent linear relationship between variables
Limitations. While correlation can reveal interesting patterns, it's crucial to remember that correlation does not prove causation. Other factors, such as confounding variables or reverse causality, may explain the observed relationship.
Real-world applications. Correlation analysis is used in various fields:
- Finance: Analyzing relationships between different investment assets
- Marketing: Identifying factors that influence consumer behavior
- Health: Studying connections between lifestyle factors and disease risk
4. Probability: Navigating Uncertainty and Risk
Probability doesn't make mistakes; people using probability make mistakes.
Basic concepts. Probability measures the likelihood of an event occurring, ranging from 0 (impossible) to 1 (certain):
- Independent events: The outcome of one does not affect the other
- Conditional probability: The likelihood of an event given another has occurred
- Expected value: The average outcome over many trials
Risk assessment. Probability helps quantify and manage risks in various domains:
- Insurance: Setting premiums based on the likelihood of claims
- Finance: Evaluating investment opportunities and potential losses
- Public health: Estimating the impact of interventions or disease outbreaks
Common misconceptions. Understanding probability can help avoid fallacies:
- Gambler's fallacy: Believing past outcomes influence future independent events
- Base rate neglect: Ignoring the underlying probability of an event
- Conjunction fallacy: Assuming specific conditions are more probable than general ones
5. The Central Limit Theorem: The Foundation of Statistical Inference
The central limit theorem is the Lebron James of statistics.
Key principle. The central limit theorem states that the distribution of sample means approximates a normal distribution, regardless of the underlying population distribution, given a large enough sample size.
Implications for inference. This theorem allows us to:
- Make inferences about populations based on sample data
- Calculate confidence intervals for population parameters
- Perform hypothesis tests to evaluate claims about populations
Practical applications. The central limit theorem is crucial in:
- Polling and survey research
- Quality control in manufacturing
- Financial modeling and risk assessment
6. Regression Analysis: Isolating Causal Relationships
Regression analysis is the miracle elixir of social science research.
Multiple variables. Regression analysis allows us to examine the relationship between a dependent variable and multiple independent variables, controlling for confounding factors.
Types of regression:
- Linear regression: For continuous dependent variables
- Logistic regression: For binary dependent variables
- Multiple regression: Incorporating several independent variables
Interpretation. Key aspects of regression results:
- Coefficients: Indicate the strength and direction of relationships
- R-squared: Measures how much variation is explained by the model
- Statistical significance: Assesses the reliability of the results
7. Program Evaluation: Measuring the Impact of Interventions
We care about what works.
Experimental design. Randomized controlled trials are the gold standard:
- Treatment group: Receives the intervention
- Control group: Does not receive the intervention
- Random assignment: Ensures groups are comparable
Quasi-experimental methods. When randomization is not possible:
- Difference-in-differences: Compares changes over time between groups
- Regression discontinuity: Exploits arbitrary cutoffs in program eligibility
- Instrumental variables: Uses external factors to simulate randomization
Challenges. Program evaluation must address:
- Selection bias: Participants may differ from non-participants
- Spillover effects: The intervention may indirectly affect the control group
- External validity: Results may not generalize to other contexts
8. Data Quality: The Cornerstone of Reliable Analysis
Garbage in, garbage out.
Data collection. Ensuring data quality starts with proper collection methods:
- Representative sampling: Avoiding selection bias
- Appropriate sample size: Balancing precision and cost
- Standardized procedures: Minimizing measurement error
Data cleaning. Preparing data for analysis involves:
- Handling missing values: Imputation or exclusion
- Identifying and addressing outliers
- Checking for consistency and logical errors
Data management. Maintaining data integrity requires:
- Clear documentation of data sources and variables
- Secure storage and backup procedures
- Version control for datasets and analysis scripts
9. Common Statistical Pitfalls: Avoiding Misinterpretation
Statistical malfeasance has very little to do with bad math.
Correlation vs. causation. Always consider alternative explanations for observed relationships:
- Reverse causality: The effect might cause the supposed cause
- Confounding variables: Other factors might explain the relationship
- Spurious correlations: Random chance can produce misleading associations
Selection bias. Be wary of non-representative samples:
- Survivorship bias: Only considering successful cases
- Self-selection bias: Participants choose to be in a study
- Publication bias: Only positive results get published
Misuse of p-values. Avoid overreliance on statistical significance:
- P-hacking: Manipulating data or analysis to achieve significance
- Multiple comparisons: Increased risk of false positives
- Practical significance: Statistical significance doesn't imply importance
10. Real-World Applications: Statistics in Action
Statistics can help answer these questions.
Public health. Statistics informs critical decisions:
- Evaluating the effectiveness of vaccines and treatments
- Identifying risk factors for diseases
- Modeling the spread of infectious diseases
Economics and finance. Statistical tools guide policy and investment:
- Forecasting economic growth and inflation
- Assessing the impact of policy changes
- Managing investment portfolios and risk
Social sciences. Statistics helps us understand human behavior:
- Analyzing voting patterns and political trends
- Studying educational outcomes and interventions
- Evaluating the effectiveness of social programs
Technology. Modern applications of statistics include:
- Machine learning and artificial intelligence
- Recommendation systems (e.g., Netflix, Amazon)
- Natural language processing and sentiment analysis
Last updated:
Review Summary
Naked Statistics receives mostly positive reviews for making statistics accessible and engaging through real-world examples and humor. Readers appreciate its clear explanations of complex concepts without heavy mathematics. Some find it too basic for those with prior statistics knowledge. The book is praised for cultivating critical thinking about statistical claims. Criticisms include occasional dry sections and overuse of American sports examples. Overall, it's recommended as an introductory text for students and general readers interested in understanding statistics.
Similar Books
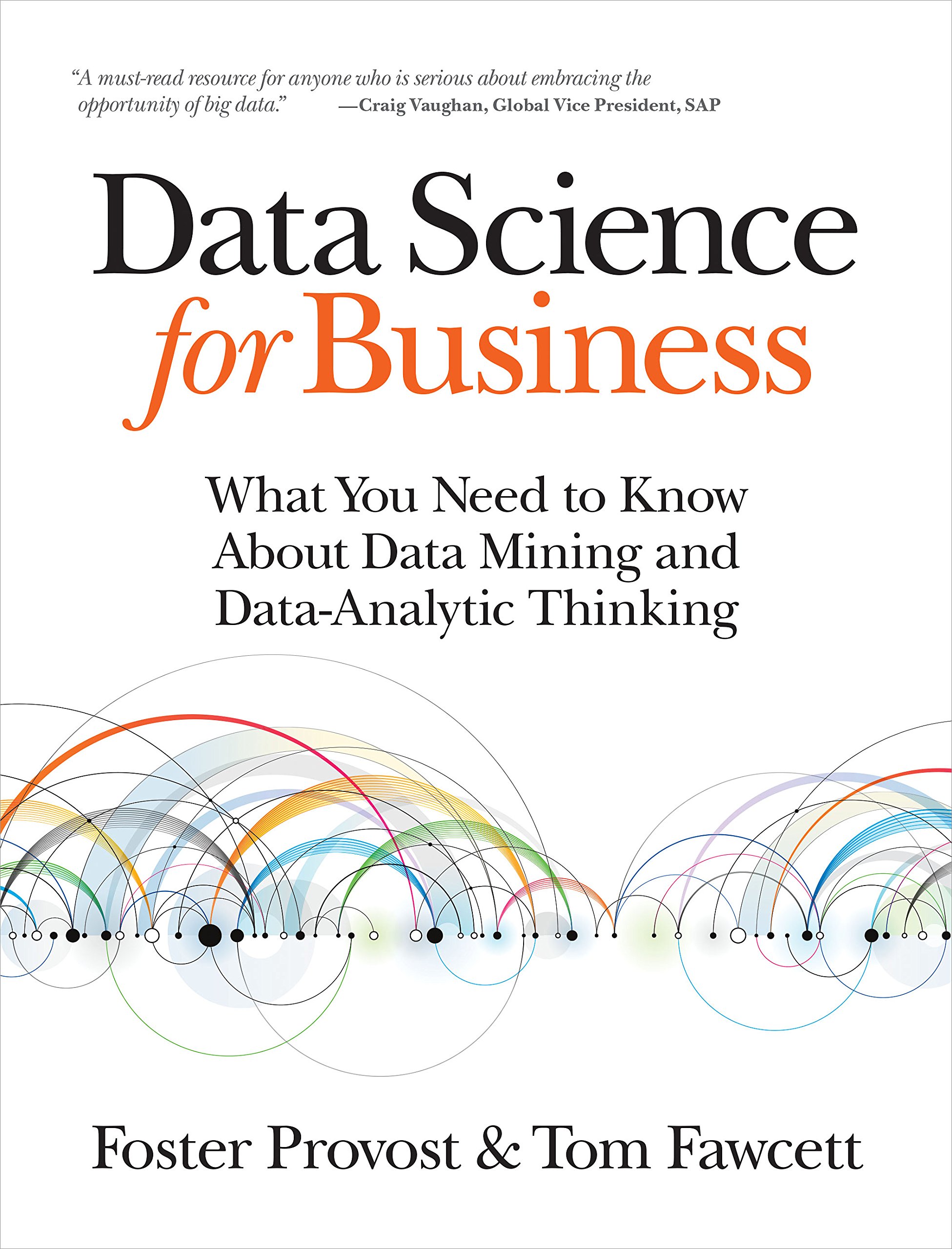
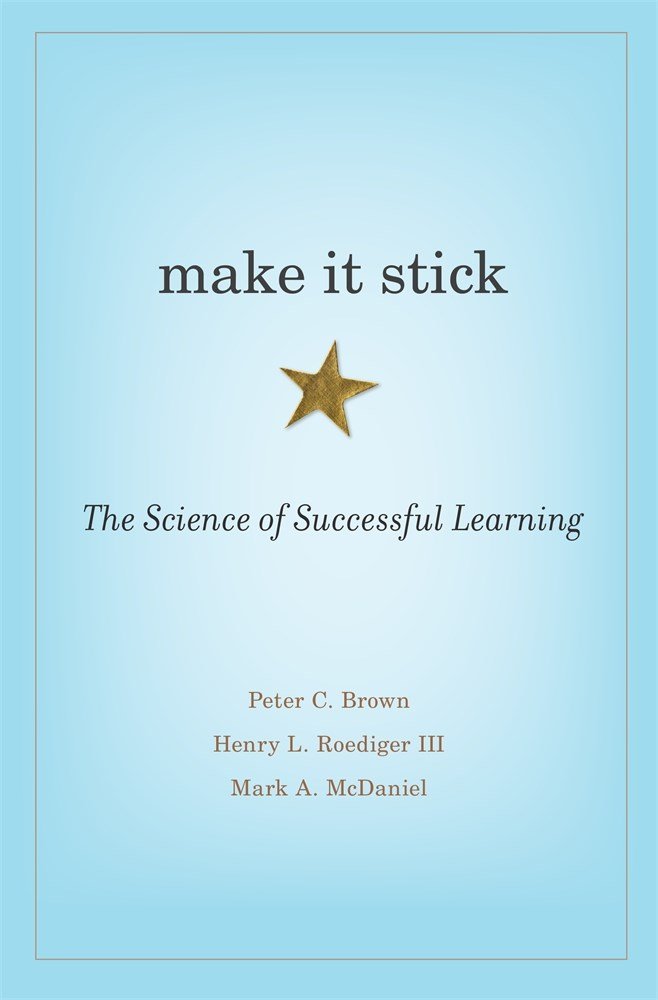
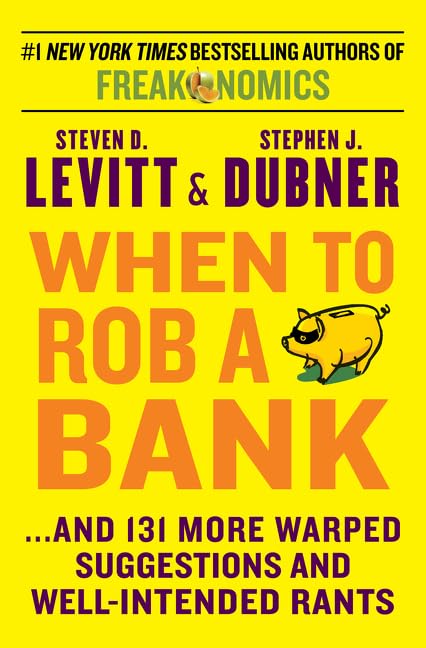
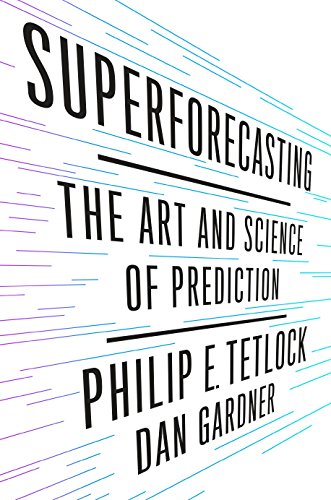
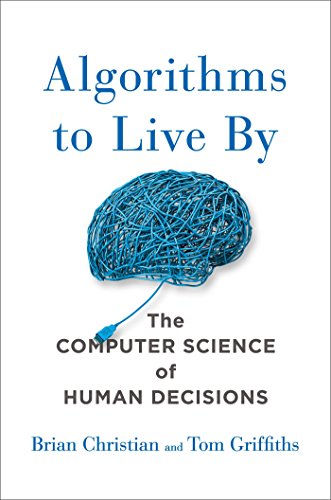
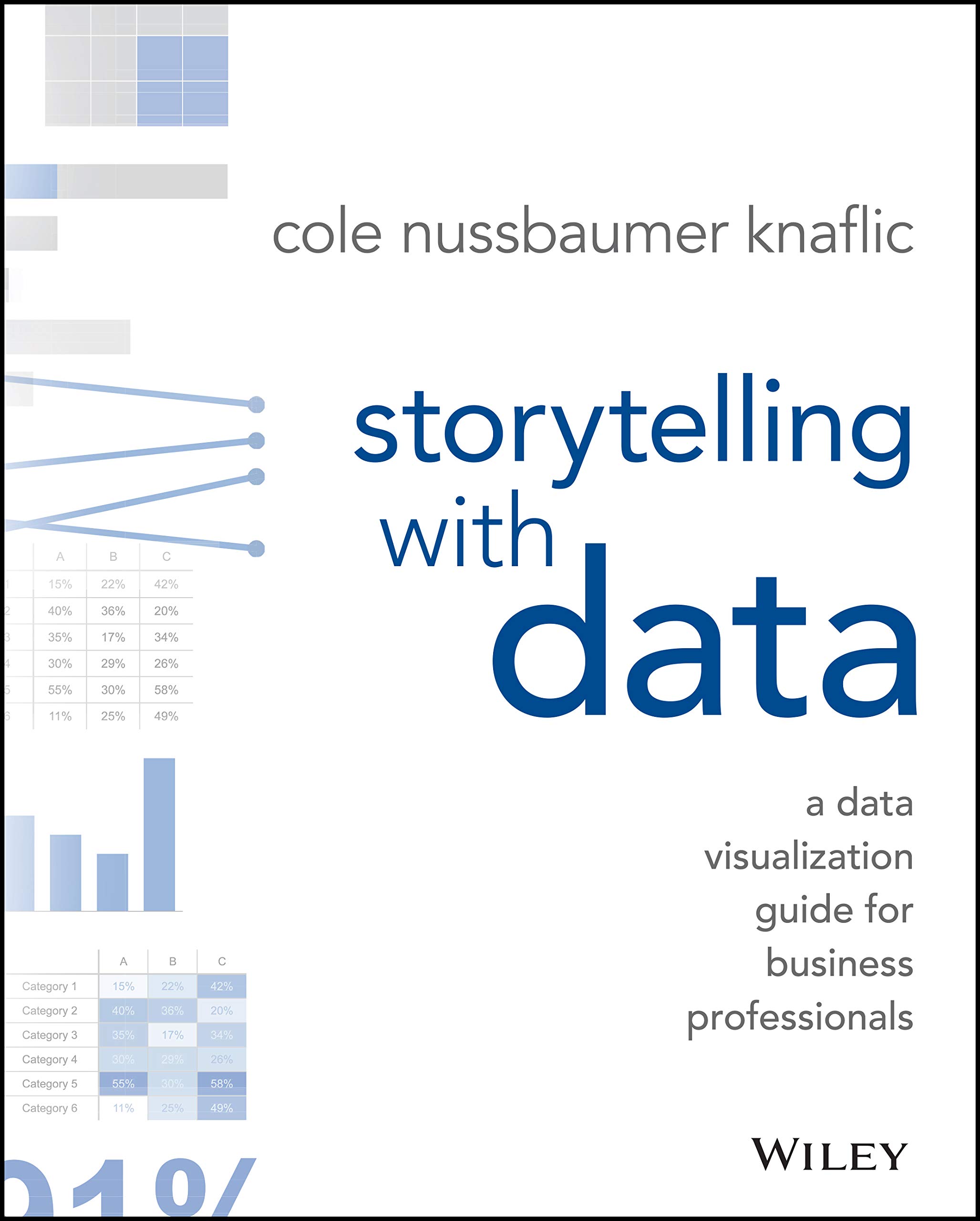
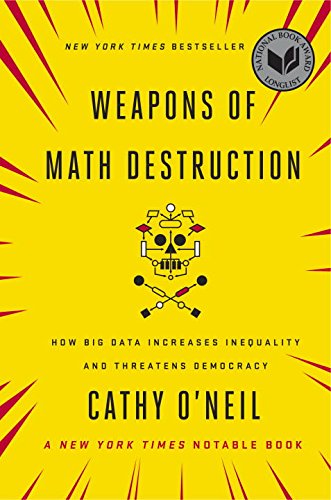

Download PDF
Download EPUB
.epub
digital book format is ideal for reading ebooks on phones, tablets, and e-readers.