Key Takeaways
1. Statistics: Measuring and Interpreting Data for Informed Decisions
Statistics is the measuring of data and interpreting that data to prove or disprove a point.
Data-driven insights. Statistics is a powerful tool for transforming raw data into actionable insights. It involves collecting, analyzing, and interpreting numerical information to draw conclusions, identify trends, and make informed decisions across various fields. From business and science to politics and sports, statistics provides a framework for understanding the world around us.
Core statistical concepts. The foundation of statistics lies in understanding key concepts such as:
- Frequency: How often an event occurs.
- Distribution: How data is spread across a range of values.
- Randomness: The unpredictable nature of events.
- Cause/effect relationships: How one variable influences another.
Applications of statistics. By mastering these concepts, individuals can leverage statistics to:
- Predict future outcomes.
- Identify patterns in data.
- Evaluate the effectiveness of interventions.
- Support decision-making with evidence-based analysis.
2. Data Collection: The Foundation of Sound Statistical Analysis
In fact, the collection of the data for any study can be the most critical factor in finding valid results.
Garbage in, garbage out. The quality of any statistical analysis is directly dependent on the quality of the data used. Accurate, reliable, and unbiased data collection is essential for drawing valid conclusions and making informed decisions.
Key considerations for data collection:
- Sampling: Selecting a representative subset of the population.
- Randomness: Ensuring that each member of the population has an equal chance of being selected.
- Sample size: Collecting enough data points to achieve statistical significance.
- Bias: Minimizing systematic errors that can skew the results.
Data sources. Data can be sourced from various places, including:
- Government databases (e.g., US Census Bureau).
- Financial databases (e.g., Yahoo! Finance).
- Online surveys (e.g., SurveyMonkey).
- Personal interviews.
- Mailed questionnaires.
3. Descriptive vs. Inferential Statistics: Understanding the Two Branches
If you are describing the data with measures of center, spread, or shape, then it’s descriptive statistics.
Two sides of the same coin. Statistics can be broadly categorized into two branches: descriptive and inferential. Descriptive statistics focuses on summarizing and presenting data in a meaningful way, while inferential statistics uses sample data to make generalizations about larger populations.
Descriptive statistics. This branch involves calculating measures such as:
- Mean: The average value.
- Median: The middle value.
- Mode: The most frequent value.
- Range: The difference between the highest and lowest values.
- Standard deviation: A measure of data spread around the mean.
Inferential statistics. This branch involves:
- Making educated guesses about population parameters.
- Testing theories and hypotheses.
- Modeling relationships between variables.
- Predicting future outcomes.
4. Visualizing Data: Unveiling Patterns Through Charts and Graphs
Sometimes it’s best to look at the data in a statistical problem with more than just numbers: sometimes you need to look at it with shapes, charts, and graphs.
Pictures speak louder than numbers. Visualizing data through charts and graphs can reveal patterns, trends, and relationships that might be missed when looking at raw numbers alone. Effective visualizations can communicate complex information in a clear and concise manner.
Common types of statistical charts and graphs:
- Dot plots: Displaying frequency of observations.
- Bar charts: Comparing quantities across categories.
- Histograms: Showing the distribution of continuous data.
- Frequency polygons: Estimating the shape of a distribution.
- Stem and leaf plots: Displaying data while preserving individual values.
- Time series graphs: Tracking data over time.
- Pie charts: Representing proportions of a whole.
Choosing the right visualization. The choice of chart or graph depends on the type of data and the message you want to convey. Consider the following:
- Use bar graphs for distinct entities (e.g., shoe sizes).
- Use histograms for continuous intervals (e.g., test scores).
- Use pie charts for data that adds up to 100%.
5. Measures of Central Tendency: Finding the Middle Ground
By determining the mean, you will be able to measure the middle of the data, or the average, of the frequency of both gaining and losing stock prices.
Defining the "average." Measures of central tendency provide a single value that represents the "center" of a dataset. The three most common measures are the mean, median, and mode, each with its own strengths and weaknesses.
Mean:
- Calculated by summing all values and dividing by the number of values.
- Sensitive to outliers (extreme values).
- Useful for data that is evenly distributed.
Median:
- The middle value when data is sorted.
- Less sensitive to outliers.
- Useful for skewed data.
Mode:
- The most frequent value.
- Can be used for both numerical and categorical data.
- May not exist or may be multiple modes.
Choosing the right measure. The best measure of central tendency depends on the nature of the data and the purpose of the analysis. The median is often the best stand-alone measure because it is not affected by the magnitude of the data.
6. Probability: Quantifying Uncertainty and Predicting Outcomes
Probability is the measure of whether and how frequently something will happen.
The language of chance. Probability provides a framework for quantifying uncertainty and predicting the likelihood of events. It is a fundamental concept in statistics and is used extensively in fields such as finance, marketing, and medicine.
Key concepts in probability:
- Empirical probability: Based on past experiences and observations.
- Subjective probability: Based on personal beliefs and judgments.
- Conditional probability: The probability of an event given that another event has already occurred.
- Independent events: Events whose outcomes do not affect each other.
- Mutually exclusive events: Events that cannot occur simultaneously.
Rules of probability:
- The probability of an event must be between 0 and 1.
- The sum of probabilities of all possible outcomes must equal 1.
Probability distributions. These mathematical functions describe the probability of different outcomes for a random variable. Common distributions include:
- Binomial distribution: For events with two possible outcomes (e.g., yes/no).
- Normal distribution: A bell-shaped curve that describes many natural phenomena.
7. Hypothesis Testing: Validating Claims with Statistical Evidence
With statistics, the goal is usually 95 percent accuracy before a researcher can assume that the guess is correct.
The scientific method in action. Hypothesis testing is a formal procedure for evaluating evidence and determining whether to support or reject a claim about a population. It involves formulating a null hypothesis (a statement to be tested) and an alternative hypothesis (the opposite of the null hypothesis).
Steps in hypothesis testing:
- State the null and alternative hypotheses.
- Choose a significance level (α), which represents the probability of rejecting the null hypothesis when it is true.
- Calculate a test statistic based on sample data.
- Determine the p-value, which is the probability of obtaining the observed results (or more extreme results) if the null hypothesis is true.
- Compare the p-value to the significance level. If the p-value is less than α, reject the null hypothesis.
Types of errors:
- Type I error: Rejecting the null hypothesis when it is true (false positive).
- Type II error: Failing to reject the null hypothesis when it is false (false negative).
8. Regression Analysis: Modeling Relationships and Predicting the Future
A predictive model uses a study’s results and then builds a tool that gives a good chance of predicting the future with similar data.
Uncovering hidden connections. Regression analysis is a statistical technique for modeling the relationship between a dependent variable (the outcome you want to predict) and one or more independent variables (the factors that might influence the outcome).
Types of regression:
- Simple linear regression: Modeling the relationship between two variables with a straight line.
- Multiple regression: Modeling the relationship between one dependent variable and multiple independent variables.
Key concepts in regression analysis:
- Regression equation: A mathematical formula that describes the relationship between the variables.
- Correlation coefficient (r): A measure of the strength and direction of the linear relationship between two variables.
- Coefficient of determination (R2): A measure of the proportion of variance in the dependent variable that is explained by the independent variables.
- Significance level (p-value): A measure of the statistical significance of the relationship between the variables.
Building predictive models. Regression analysis can be used to build predictive models that forecast future outcomes based on past data. These models are used in various fields, including:
- Marketing (e.g., predicting sales based on advertising spending).
- Finance (e.g., predicting stock prices based on economic indicators).
- Medicine (e.g., predicting patient outcomes based on medical history).
9. Data Quality: Ensuring Accuracy and Reliability in Statistical Studies
One of the best ways to determine the quality of your data is to first measure how many responses you’ve received.
The cornerstone of valid results. The quality of data is paramount in statistical analysis. Inaccurate, incomplete, or biased data can lead to misleading conclusions and flawed decision-making.
Factors affecting data quality:
- Accuracy: The extent to which data reflects the true values.
- Completeness: The extent to which all required data is present.
- Consistency: The extent to which data is free from contradictions.
- Validity: The extent to which data measures what it is supposed to measure.
- Reliability: The extent to which data is consistent over time and across different sources.
Strategies for ensuring data quality:
- Careful planning of data collection methods.
- Thorough training of data collectors.
- Implementation of data validation procedures.
- Regular monitoring of data quality metrics.
10. Ethics in Statistics: Maintaining Integrity and Avoiding Misrepresentation
When you use statistics, you are looking at groups of numbers from surveys and studies and then measuring how the numbers are related to each other.
Responsibility in data analysis. Statistics, while a powerful tool, can be misused to manipulate or misrepresent information. Ethical considerations are crucial in ensuring that statistical analyses are conducted responsibly and that results are presented fairly and accurately.
Ethical principles in statistics:
- Objectivity: Avoiding bias in data collection and analysis.
- Transparency: Clearly disclosing methods and assumptions.
- Accuracy: Presenting results truthfully and without distortion.
- Confidentiality: Protecting the privacy of individuals whose data is being used.
- Responsibility: Acknowledging the limitations of statistical analyses and avoiding overgeneralizations.
Avoiding misrepresentation. Be wary of:
- Cherry-picking data to support a particular viewpoint.
- Using misleading visualizations.
- Ignoring outliers that contradict the desired conclusion.
- Overstating the significance of results.
Last updated:
Review Summary
Statistics 101 receives mixed reviews, with an average rating of 2.88 out of 5. Many readers criticize the book for containing errors, typos, and confusing explanations. Some find it shallow and not suitable for beginners. However, a few reviewers appreciate its concise overview and accessible language. Critics note issues with mathematical calculations and disconnected paragraphs. While some readers found it helpful as a quick refresher, others suggest seeking better alternatives for learning statistics. Overall, the book's reception is largely negative due to its perceived lack of depth and accuracy.
Similar Books
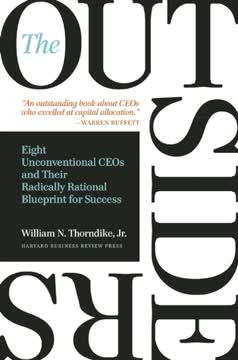
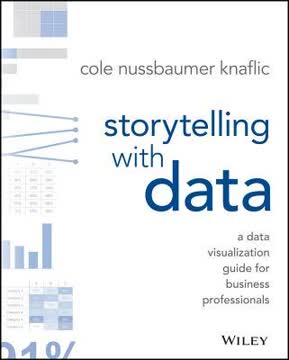
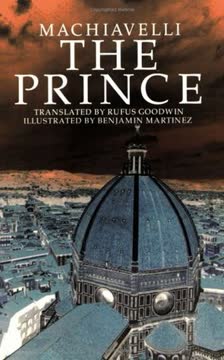
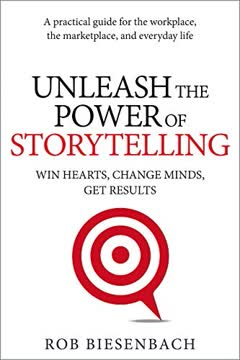
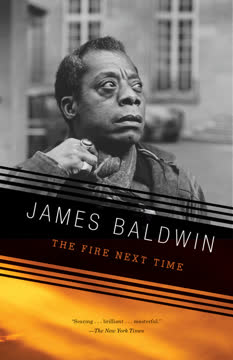
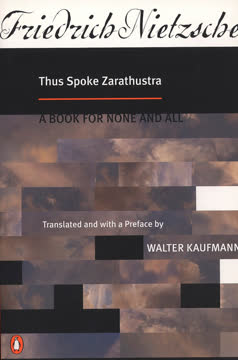
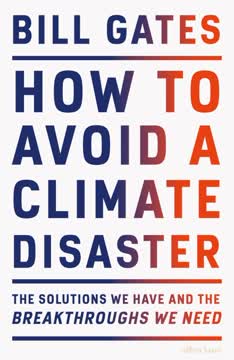
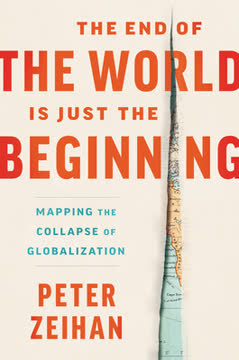
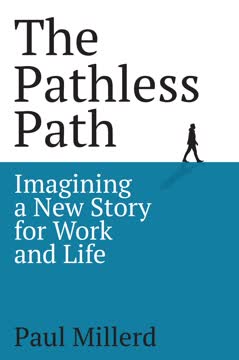
Download PDF
Download EPUB
.epub
digital book format is ideal for reading ebooks on phones, tablets, and e-readers.