Key Takeaways
1. Bayes' Rule: A Simple Idea for Updating Belief
On its face Bayes’ rule is a simple, one-line theorem: by updating our initial belief about something with objective new information, we get a new and improved belief.
An elegant statement. At its core, Bayes' rule is a straightforward mathematical formula describing how to revise an existing belief in light of new evidence. It posits that a revised belief (posterior probability) is proportional to the initial belief (prior probability) multiplied by the likelihood of observing the new data given the initial belief. This process allows for continuous learning and refinement of understanding as more information becomes available.
Learning from experience. The rule provides a quantitative framework for learning from experience, moving from an initial state of uncertainty or belief to a more informed state. It formalizes the intuitive process of adjusting one's opinion when presented with new facts. This simple concept, discovered by the Reverend Thomas Bayes in the 1740s, laid the groundwork for a mathematical approach to decision-making under uncertainty.
A powerful logic. While simple in concept, the rule's power lies in its ability to combine different types of information – subjective initial beliefs and objective new data – into a coherent framework. It offers a logical way to reason about the world, particularly in situations where absolute certainty is impossible and decisions must be made based on incomplete or ambiguous information.
2. Born in Controversy: The Enduring Debate Over Subjective Priors
Opponents, meanwhile, regarded Bayes’ rule as subjectivity run amok.
The problem of priors. The most contentious aspect of Bayes' rule has always been the "prior probability" – the initial belief assigned before any new data is considered. Critics argued that introducing subjective hunches or opinions into rigorous mathematical analysis was unscientific and undermined objectivity. This philosophical divide sparked centuries of debate.
Quantifying ignorance. Early applications, like assuming equal probabilities when nothing was known (the "law of insufficient reason"), were particularly attacked. Critics saw this as attempting to quantify ignorance, arguing that a lack of knowledge should not be represented by a specific probability distribution. This fueled the perception that the rule was arbitrary and unreliable.
A fundamental disagreement. The controversy reflected a deeper conflict about the nature of probability itself. Frequentists believed probability should only measure the long-run frequency of repeatable events, demanding objective data. Bayesians, however, argued that probability could also measure a subjective degree of belief, applicable even to unique, unrepeatable events. This fundamental disagreement led to a bitter, long-lasting schism in the field of statistics.
3. Declared Dead by Theorists, Kept Alive by Practitioners
Theoretically, Bayes’ rule was verboten. But it could deal with all kinds of data, whether copious or sparse.
Academic disdain. Despite its logical appeal, Bayes' rule was largely rejected by leading statistical theorists for over a century, particularly after Laplace. Figures like Karl Pearson and Ronald Fisher vehemently opposed its use, viewing it as unscientific due to its reliance on subjective priors. They actively campaigned against it, declaring it "dead and buried."
Real-world utility. Yet, while academic statisticians shunned the rule, practitioners facing real-world problems found it indispensable. It was used in diverse fields where decisions had to be made with limited or uncertain information:
- French artillery aiming
- US insurance premium setting (Credibility theory)
- Bell Telephone system management
- Assessing judicial evidence (Dreyfus affair)
A tool for uncertainty. These practitioners needed a method that could incorporate all available information, including expert judgment and historical data, and provide updated probabilities for unique situations. Frequentist methods, designed for repeatable experiments and large datasets, were often inadequate. Bayes' rule, despite its theoretical controversies, proved to be a powerful tool for navigating uncertainty in practice.
4. Secret Wartime Triumphs in Codebreaking and Search
One spectacular success occurred during the Second World War, when Alan Turing developed Bayes to break Enigma, the German navy’s secret code, and in the process helped to both save Britain and invent modern electronic computers and software.
Classified applications. The Second World War provided critical, albeit secret, opportunities for Bayes' rule to prove its power. Facing life-or-death decisions with incomplete information, military and intelligence agencies turned to methods that could update beliefs rapidly.
- Alan Turing's Bayesian system (Banburismus, using "bans") was crucial for breaking German naval Enigma codes.
- Claude Shannon used Bayesian concepts for information theory and cryptography at Bell Labs.
- Andrei Kolmogorov applied Bayesian ideas to artillery aiming in Russia.
- Bernard Koopman developed Bayesian search theory for the US Navy to hunt submarines.
A vital tool for decision-making. These wartime applications demonstrated Bayes' effectiveness in sequential analysis and decision-making under extreme uncertainty, often with limited data. The ability to quantify and update beliefs based on incoming intelligence was invaluable.
Hidden impact. Despite these vital contributions to the Allied victory, the successes of these Bayesian methods remained highly classified after the war. This secrecy prevented the wider statistical community from learning about the rule's practical power, contributing to its continued marginalization in academic circles for decades.
5. The Post-War Revival: Building a Logical Foundation
To its adherents, it is an elegant statement about learning from experience.
A new generation of theorists. After the war, a small but dedicated group of statisticians began to build a rigorous theoretical foundation for Bayesian methods. Figures like Leonard Jimmie Savage and Dennis Lindley developed the philosophical and mathematical underpinnings, arguing that probability as a measure of subjective belief was the only logical basis for statistical inference and decision-making.
Subjectivity embraced. Savage, in particular, championed the idea of "personal probability," asserting that subjective beliefs could be quantified and updated rationally using Bayes' rule. He argued that this approach provided a coherent framework for decision-making under uncertainty, unlike the fragmented methods of frequentism.
Intense debate continues. This theoretical revival sparked renewed and often acrimonious debates with the frequentist establishment. While Bayesians built elegant logical systems, they still faced the challenge of demonstrating the practical superiority of their methods, particularly given the computational difficulties involved in applying Bayes to complex problems.
6. Proving Its Value on Complex Real-World Problems
Drawing on primary source material and interviews with statisticians and other scientists, The Theory That Would Not Die is the riveting account of how a seemingly simple theorem ignited one of the greatest controversies of all time.
Tackling the intractable. Despite computational limitations and academic resistance, a few determined researchers began applying Bayes' rule to complex, real-world problems that were difficult for frequentist methods to handle. These applications showcased the rule's unique strengths:
- Jerome Cornfield used Bayes to analyze epidemiological data, linking smoking to lung cancer and identifying heart disease risk factors.
- Fred Iklé and Albert Madansky used Bayes at RAND to assess the probability of accidental nuclear detonations.
- Frederick Mosteller and David Wallace used Bayes and early computers to resolve the authorship of the disputed Federalist Papers.
- Robert Schlaifer and Howard Raiffa developed Bayesian decision theory for business, introducing practical tools like decision trees.
Beyond simple averages. These studies often involved combining diverse sources of information, dealing with small or biased datasets, and making inferences about unique events – situations where frequentist assumptions were strained. They demonstrated that Bayes could provide valuable insights and support decision-making in complex, uncertain environments.
Laying the groundwork. While these applications were often laborious and sometimes faced skepticism, they provided crucial public demonstrations of Bayes' potential. They challenged the prevailing view that the rule was merely a theoretical curiosity and highlighted the need for computational tools that could unlock its power for more widespread use.
7. The Computational Revolution Makes Bayes Practical
Today, Bayes’ rule is used everywhere from DNA de-coding to Homeland Security.
The curse of dimensionality. As computers generated vast, complex datasets with numerous variables (the "curse of high dimensionality"), traditional statistical methods struggled. Bayesian calculations, which often involve complex integration, were particularly challenging. For decades, the lack of computational power was a major barrier to applying Bayes to realistic problems.
A breakthrough algorithm. The situation changed dramatically with the development and popularization of Markov Chain Monte Carlo (MCMC) methods in the late 1980s and early 1990s. This technique, which uses random sampling (Monte Carlo) guided by dependencies (Markov chains), provided an efficient way to perform the complex calculations required by Bayes' rule, replacing difficult integration.
- Pioneered by physicists (Metropolis, Hastings) and later generalized by statisticians (Geman, Smith, Gelfand, Wong, Tanner).
- Made complex Bayesian models computationally feasible for the first time.
Unlocking the power. MCMC, along with user-friendly software like BUGS, transformed Bayes from a theoretically elegant but practically difficult method into a powerful, accessible tool. This computational revolution was the key factor in the rule's eventual widespread adoption across numerous scientific and commercial fields.
8. From Obscurity to Ubiquity: Bayes Permeates Modern Life
It has penetrated computer science, artificial intelligence, machine learning, Wall Street, astronomy and physics, Homeland Security, Microsoft, and Google.
Widespread adoption. With computational barriers removed, Bayes' rule experienced a dramatic surge in popularity and application. Its ability to handle uncertainty, combine diverse information, and make inferences from complex data made it invaluable in the age of big data.
- Internet: Spam filtering, search engines, recommender systems (Netflix).
- Technology: Speech recognition, machine translation, image processing, robotics (driverless cars).
- Science: Genetics, astronomy, physics, ecology, medical testing.
- Finance: Hedge funds, risk assessment.
- Security: Antiterrorism, military tracking.
A versatile tool. Bayes' rule, often implemented through Bayesian networks and MCMC, became a go-to method for problems involving pattern recognition, prediction, and decision-making under uncertainty. Its flexibility allowed it to be adapted to a vast array of applications, far beyond the dreams of its early proponents.
Pragmatism prevails. The widespread adoption was driven less by philosophical conversion and more by practical results. Bayes simply worked better than alternative methods for many complex, data-rich problems, leading to its integration into the core technologies and research methodologies of the twenty-first century.
9. A Metaphor for the Mind: The Bayesian Brain
In short, growing evidence suggests that we have Bayesian brains.
Understanding learning. Beyond its practical applications, Bayes' rule has emerged as a powerful theoretical framework for understanding fundamental processes, including how humans learn and perceive the world. The "Bayesian Brain" hypothesis proposes that the brain operates by performing Bayesian-like computations.
Combining prior and sensory data. This theory suggests that the brain constantly combines prior knowledge and expectations about the environment with noisy, incomplete sensory information to form perceptions and make decisions. This process allows us to navigate an uncertain world effectively, making inferences about reality based on limited cues.
A fundamental principle. From simple motor control (like estimating where to reach) to complex perception (like recognizing objects in blurry images), the brain appears to use Bayesian principles to minimize uncertainty and optimize responses. This perspective elevates Bayes' rule from a statistical tool to a potential model for the very mechanisms of human cognition and learning.
Last updated:
Review Summary
The Theory That Would Not Die explores the history of Bayes' theorem, from its origins to modern applications. Readers appreciate the engaging storytelling and historical context but some find it lacks technical depth. The book highlights the theorem's controversial nature and its eventual acceptance in various fields. While praised for its accessibility, some criticize the oversimplification of complex concepts and perceived bias against frequentist statistics. Overall, it offers an intriguing look at the development and impact of Bayesian statistics.
Similar Books
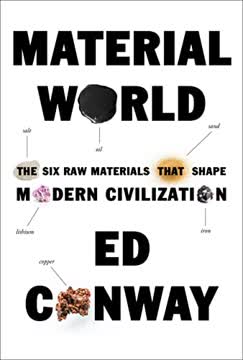
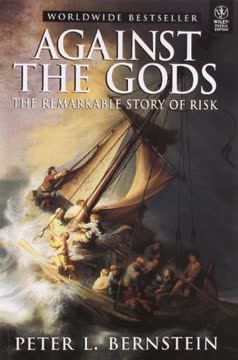
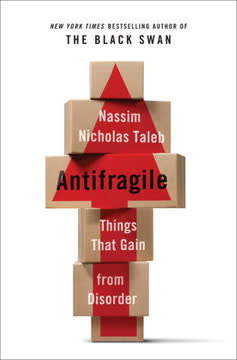
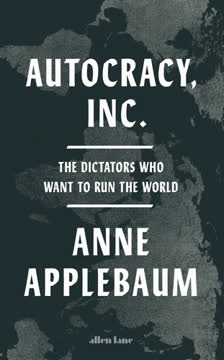


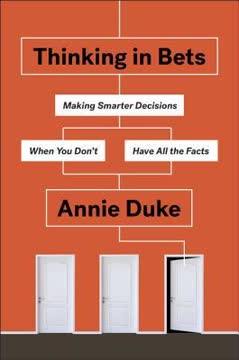
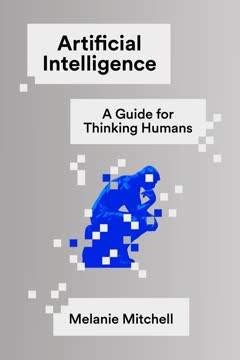
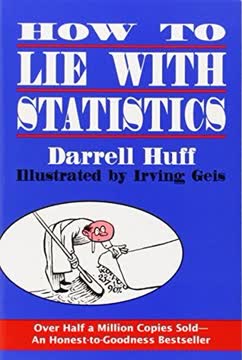
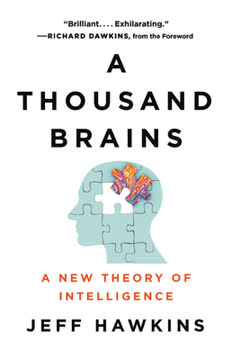
Download PDF
Download EPUB
.epub
digital book format is ideal for reading ebooks on phones, tablets, and e-readers.