重点摘要
1. 开发一个成功的算法交易系统需要严格的测试和情绪纪律
“如果看起来好得令人难以置信,那它可能真的不可信。”
严格测试至关重要。 开发一个盈利的算法交易系统需要广泛的回测、样本外测试和实时评估。避免常见的陷阱,如曲线拟合、过度优化和仅依赖历史数据。确保在多种市场条件和时间框架下测试你的策略,以确保其稳健性。
情绪纪律是必不可少的。 即使在算法交易中,交易心理也起着重要作用。要为回撤和亏损期做好准备,并坚持预先定义的规则和风险管理指南。避免根据短期结果或情绪调整系统的诱惑。
- 稳健测试过程的关键组成部分:
- 历史回测
- 样本外测试
- 前向分析
- 蒙特卡洛模拟
- 实时纸上交易(孵化)
2. 设置SMART目标并为交易策略创建结构化开发过程
“如果你想完成某件事,你必须有目标。”
定义SMART目标。 为你的交易系统设定具体的、可衡量的、可实现的、相关的和有时间限制的目标。这为开发和评估提供了明确的框架。例如,目标是特定年度回报率,并在定义的时间范围内限制最大回撤。
遵循结构化过程。 创建一个逐步的开发过程,以确保一致性和彻底性。这应包括创意生成、初步测试、深入分析和实时评估。记录每一步,以保持清晰并允许未来的改进。
- 策略开发过程的关键阶段:
- 确立目标和宗旨
- 生成交易想法
- 进行有限测试
- 执行前向分析
- 运行蒙特卡洛模拟
- 实时孵化策略
- 评估多样化潜力
- 实施头寸规模规则
3. 使用前向分析和蒙特卡洛模拟验证交易系统
“前向分析往往会产生更稳定的权益曲线。”
前向分析减轻过拟合。 这种技术涉及在一部分历史数据上优化参数,然后在随后的样本外期间进行测试。多次重复此过程,以更现实地表示策略在实际交易中的表现。
蒙特卡洛模拟提供概率洞察。 通过随机化历史交易的顺序,蒙特卡洛分析有助于估计交易系统的潜在结果范围。这包括最大回撤、年度回报和破产风险等指标,提供更全面的策略风险回报概况。
- 前向分析和蒙特卡洛模拟的关键好处:
- 减少曲线拟合和过拟合
- 更现实的性能预期
- 更好地理解潜在回撤和风险
- 提高对策略稳健性的信心
4. 多样化多个不相关策略对于长期成功至关重要
“适当的多样化,可能是我见过的最接近所谓交易‘圣杯’的东西。”
分散风险于策略之间。 开发和交易多个不相关的策略,以减少整体投资组合风险。这有助于平滑权益曲线,并随着时间的推移提供更一致的回报。目标是具有不同市场、时间框架和交易风格的策略。
衡量多样化效果。 使用相关性分析、权益曲线线性度和组合蒙特卡洛模拟来评估策略组合的多样化收益。持续监控和调整策略组合,以保持最佳多样化。
- 实现策略多样化的方法:
- 交易不同市场(如货币、商品、指数)
- 变换时间框架(如日内、波段、长期)
- 采用不同的交易风格(如趋势跟踪、均值回归、突破)
- 使用不相关的进出场规则
5. 头寸规模和风险管理与交易策略本身同样重要
“如果你交易更多合约,你的回报会上升,但风险也会增加。”
实施稳健的头寸规模。 开发一种头寸规模方法,平衡潜在回报与可接受的风险水平。常见的方法包括固定比例、固定比率和最优f。根据账户权益和市场条件定期审查和调整头寸规模规则。
在多个层面管理风险。 在交易、策略和投资组合层面实施风险管理。设置止损、定义最大回撤限额,并建立停止策略的标准。考虑使用期权或其他对冲技术,以在极端市场条件下限制下行风险。
- 关键风险管理考虑因素:
- 每笔交易的风险限额
- 策略级别的回撤阈值
- 投资组合范围的风险分配
- 策略之间的相关性
- 整体账户破产风险
6. 密切监控实时策略表现,并准备放弃表现不佳的系统
“当你的实际表现低于预期表现时,这不就像有人在偷你的钱吗?”
跟踪绩效指标。 定期监控关键绩效指标,如利润因子、夏普比率、最大回撤和胜率。将实际结果与基于历史测试和蒙特卡洛模拟的预期表现进行比较。使用权益曲线和回撤图进行可视化分析。
建立明确的退出标准。 定义停止交易策略的具体条件。这可以基于最大回撤、连续亏损交易或与预期表现的显著偏差。坚持这些预定义的规则,以避免在回撤期间的情绪决策。
- 绩效监控工具:
- 每日/每周绩效图表
- 带标准差带的权益曲线
- 回撤分析
- 基于蒙特卡洛的绩效范围
- 策略相关性矩阵
7. 自动化交易需要持续警惕和处理意外问题的计划
“自动化交易并不意味着无人值守交易。”
保持警惕。 定期监控你的自动化交易系统,以发现潜在问题,如数据馈送问题、执行错误或意外的市场条件。实施诸如每日头寸检查和异常活动的自动警报等保护措施。
为意外情况做好准备。 为各种场景制定应急计划,如停电、互联网中断或软件故障。准备备用系统,并明确手动干预的程序。定期测试和更新你的灾难恢复计划。
- 自动化交易的关键考虑因素:
- 交易算法中的稳健错误处理
- 冗余的互联网连接和电源供应
- 明确的手动覆盖程序
- 定期系统健康检查和维护
- 持续监控交易和头寸
最后更新日期:
FAQ
What's Building Winning Algorithmic Trading Systems about?
- Focus on Algorithmic Trading: The book details Kevin J. Davey's journey from a novice to a successful algorithmic trader, emphasizing the development of mechanical trading systems using data analysis and statistical methods.
- Comprehensive Guide: It covers system design, testing, and live trading, providing a practical guide for traders at all levels to create and implement their own trading systems.
- Real-Life Experiences: Davey shares personal anecdotes and lessons from his trading career, offering relatable insights into the trading world.
Why should I read Building Winning Algorithmic Trading Systems?
- Learn from Experience: Kevin J. Davey is a proven trader with significant success in trading competitions, offering practical and applicable insights.
- Structured Approach: The book provides a systematic methodology for developing trading systems, including testing methods like Monte Carlo analysis and walk-forward testing.
- Diverse Audience: It offers valuable information for both beginners and experienced traders to enhance their trading strategies and decision-making processes.
What are the key takeaways of Building Winning Algorithmic Trading Systems?
- Importance of Testing: Rigorous testing, including historical back-testing and Monte Carlo analysis, is crucial to ensure trading systems are robust.
- Psychological Aspects: Understanding and managing psychological challenges is essential for maintaining discipline and confidence in trading.
- Continuous Improvement: Traders should regularly evaluate and adapt their strategies based on performance data for long-term success.
What is Monte Carlo analysis in Building Winning Algorithmic Trading Systems?
- Simulation of Trade Outcomes: Monte Carlo analysis simulates potential outcomes by varying the order of trades, assessing risk and potential drawdowns.
- Understanding Risk: It helps traders understand the likelihood of different outcomes, crucial for effective risk management.
- Input Requirements: Requires inputs like starting equity and expected trades to generate a realistic picture of strategy performance.
How does Kevin J. Davey suggest developing a trading system in Building Winning Algorithmic Trading Systems?
- Set SMART Goals: Emphasizes setting Specific, Measurable, Attainable, Relevant, and Time-bound goals for clarity and direction.
- Iterative Testing Process: Recommends evaluating strategies in stages to identify viable ones without overfitting to historical data.
- Focus on Entries and Exits: Encourages developing clear rules for both entry and exit strategies to enhance profitability.
What is walk-forward analysis as described in Building Winning Algorithmic Trading Systems?
- Testing Methodology: Involves optimizing a strategy over a period and testing it on subsequent out-of-sample data to assess adaptability.
- In-Sample and Out-of-Sample: Divides analysis into periods for optimization and testing, helping avoid overfitting.
- Realistic Performance Expectations: Provides a realistic expectation of strategy performance in live trading by identifying potential weaknesses.
What are the common pitfalls in algorithmic trading mentioned in Building Winning Algorithmic Trading Systems?
- Over-Optimization: Tweaking strategies excessively to fit historical data can lead to poor live performance.
- Ignoring Market Changes: Failing to adapt strategies to changing conditions can render them ineffective.
- Emotional Decision-Making: Emotions can influence decisions, making discipline crucial for sticking to strategies.
How can I ensure my trading strategy is robust as per Building Winning Algorithmic Trading Systems?
- Rigorous Testing: Use historical back-testing, walk-forward analysis, and Monte Carlo simulations to validate performance.
- Diversification: Incorporate multiple uncorrelated strategies to reduce risk and improve performance.
- Continuous Monitoring: Regularly evaluate real-time performance against historical expectations for timely adjustments.
How does Building Winning Algorithmic Trading Systems address trading psychology?
- Emotional Management: Emphasizes managing emotions to prevent impulsive decisions and maintain discipline.
- Building Confidence: Shares strategies for building confidence, crucial during drawdowns to stick to strategies.
- Learning from Mistakes: Encourages viewing mistakes as learning opportunities to foster resilience and improve performance.
What are the best quotes from Building Winning Algorithmic Trading Systems and what do they mean?
- "If it seems too good to be true, it probably is.": Cautions against over-optimizing systems and stresses realistic expectations.
- "You must have goals.": Highlights the necessity of setting clear objectives for direction and focus.
- "Treat your data with utmost care!": Stresses the importance of accurate data for reliable strategy testing and success.
How does Monte Carlo simulation work in trading strategies according to Building Winning Algorithmic Trading Systems?
- Risk Assessment Tool: Simulates thousands of outcomes to assess risk and potential performance based on historical data.
- Statistical Analysis: Evaluates metrics like probability of ruin and expected drawdown, providing insights into strategy performance.
- Informed Decision-Making: Helps traders make informed decisions about position sizing and risk management.
How does the author suggest handling losing trades in Building Winning Algorithmic Trading Systems?
- Accepting Losses: Emphasizes accepting losses as a natural part of trading to avoid emotional decision-making.
- Reviewing Performance: Advises reviewing performance to determine if losses are due to strategy flaws or market fluctuations.
- Sticking to the Strategy: Encourages consistency in following the trading plan despite short-term losses for long-term success.
评论
《构建算法交易系统,+网站》获得了大多数正面评价,平均评分为4.05/5。读者们赞赏其开发交易系统的实用、逐步指导方法,包括回测、前向分析和蒙特卡罗模拟。许多人认为这本书对初学者和有经验的交易者都很有帮助。一些人批评其过于关注期货交易和某些部分的重复性。总体而言,读者们重视这本书对算法交易的现实视角及其潜在的陷阱,一些人认为这是有志于成为算法交易者的必读书籍。
Similar Books
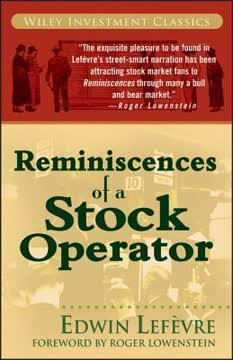
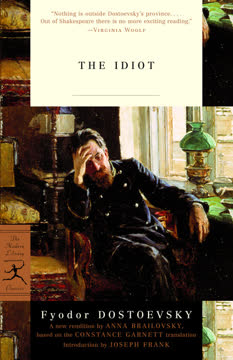
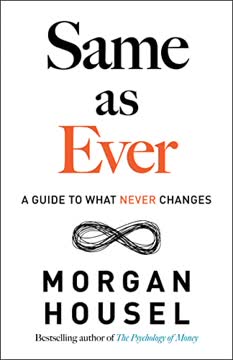
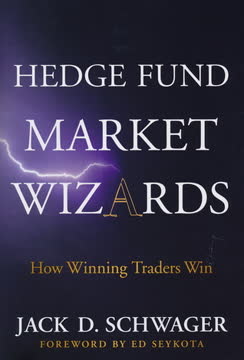
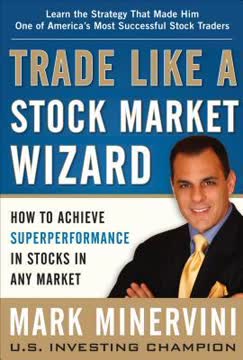
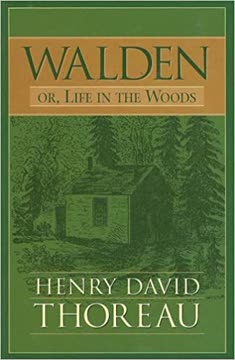
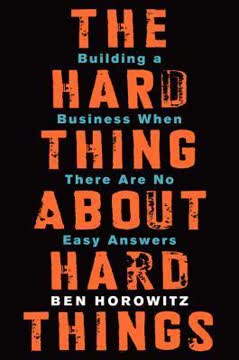
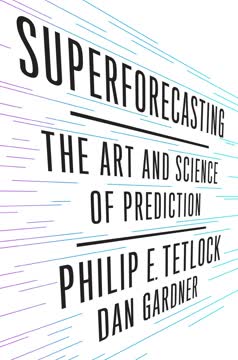