重点摘要
1. 概率不仅仅是偶然:它是决策的强大工具
帕斯卡尔的法则将相同的推理应用于每一个单独的赌注。通过这样做,它给我们一个数字——期望值——使我们能够提前判断任何赌注是否值得下注。
期望值指导决策。 帕斯卡尔的期望值计算法则为在不确定性下做出理性决策提供了框架。通过将行动的潜在后果与其发生的概率相乘,我们可以判断某一特定行动是否值得。
这一概念远不止于赌博:
- 保险决策:权衡保费与潜在损失
- 商业投资:评估潜在回报与风险
- 个人选择:评估预防健康措施的价值
了解局限性。 虽然期望值是一个强大的工具,但重要的是要认识到它的局限性:
- 它假设我们能够准确估计概率和结果
- 它没有考虑个人的风险承受能力或心理因素
- 在某些情况下,例如帕斯卡尔关于信仰上帝的赌注,这一概念可能被推向极端
2. 平均法则常被误解,但对数据解读至关重要
平均法则告诉我们,当我们知道或怀疑我们正在处理涉及偶然因素的事件时,我们应该关注的不是事件本身,而是它们的相对频率——即每个事件出现的次数与总机会数的比例。
关注相对频率。 真正的平均法则并不保证小样本中的事物会“趋于平衡”。相反,它告诉我们要关注多次试验中的结果比例。
需要记住的关键点:
- 短期结果可能与长期平均值显著偏离
- 该法则适用于相对频率,而非原始数字
- 需要大量独立事件才能可靠
实际应用:
- 赌博:理解为什么赌场在长期内总是获胜
- 科学研究:解读临床试验的结果
- 市场分析:评估投资策略随时间的表现
3. 贝叶斯定理:用证据更新信念的革命性方法
对某事的新信念水平 = 旧信念水平 + 新证据的权重
理性更新信念。 贝叶斯定理提供了一个数学框架,用于将新证据纳入我们现有的信念中。这种方法在直接实验困难或不可能的领域尤其强大。
贝叶斯推理的关键组成部分:
- 先验信念:你对概率的初步估计
- 似然性:证据与不同假设的契合程度
- 后验信念:考虑证据后的更新概率
现实世界的应用:
- 医学诊断:根据疾病流行率解读测试结果
- 刑事调查:评估法医证据的强度
- 垃圾邮件过滤器:持续改进电子邮件分类
贝叶斯方法鼓励我们:
- 明确陈述我们的先验信念和假设
- 量化新证据的强度
- 随着信息的增加逐步完善我们的理解
4. 相关性并不意味着因果关系:误解数据的危险
为什么花了这么长时间才出现这一点是科学社会学中的一个有趣问题,因为从一开始就很明显,一些研究人员对线性回归的“条款和条件”进行了极端的扭曲。
警惕虚假的相关性。 在数据中发现模式的能力既是人类认知的优势,也是劣势。虽然相关性可以提供有价值的见解,但如果不小心,它们也可能误导我们。
解读相关性时的常见陷阱:
- 将相关性与因果关系混淆
- 忽视可能解释关系的隐藏变量
- 未考虑反向因果关系
- 忽略在大数据集中偶然相关的可能性
批判性思维至关重要。 在评估基于相关性的主张时:
- 寻找可能解释关系的合理机制
- 考虑替代解释和潜在的混杂因素
- 检查不同研究中相关性的强度和一致性
- 对确认先入为主偏见的相关性保持特别怀疑
记住:我们分析的数据越多,越可能仅凭偶然发现看似显著但毫无意义的相关性。
5. 正态分布:在现实应用中的力量与局限
在寻找“真实”相关性时,仅将十个变量配对,存在90%的风险会发现至少一个纯粹是偶然的统计显著性。
无处不在的钟形曲线。 正态分布是一个强大的数学工具,准确描述了许多自然现象。它在统计学和数据分析中的普遍性源于中心极限定理,该定理表明,许多独立随机变量的总和趋向于遵循正态分布。
正态分布的关键特性:
- 围绕均值对称
- 仅由两个参数描述:均值和标准差
- 大约68%的数据落在均值的一个标准差内
局限性与危险。 虽然正态分布极为有用,但在不适用的情况下假设正态性可能导致严重错误:
- 许多现实现象具有“厚尾”,而正态分布低估了这一点
- 财务回报、自然灾害和其他极端事件通常遵循不同的分布
- 假设正态性可能导致低估罕见但灾难性风险
在处理数据时:
- 在应用假设正态性的方法之前,先测试正态性
- 了解可能更好地适合您数据的替代分布
- 考虑使用不依赖于正态性假设的稳健统计方法
6. 极端事件遵循自己的规则:尾部风险的重要性
极值理论(EVT)。
理解非凡事件。 极值理论提供了一个框架,用于分析和预测超出正常经验范围的罕见事件。这在金融、工程和环境科学等领域至关重要,因为灾难性事件可能产生巨大的影响。
极值理论的关键概念:
- 广义极值(GEV)分布
- 重现期:估计极端事件发生的频率
- 阈值超越:关注超出某一严重程度水平的事件
极值理论的应用:
- 财务风险管理:估计市场崩溃中的潜在损失
- 防洪保护:设计能够抵御罕见但严重天气事件的结构
- 保险:为灾难性风险定价
应用极值理论的挑战:
- 对极端事件的历史数据有限
- 准确估计参数的困难
- 由于气候变化等因素,极端事件频率可能存在非平稳性
7. 财务模型并非万无一失:数学方法在金融中的局限性
金融危机有许多原因,包括政治、监管和心理因素。然而,它们都根植于同一种现象:人类试图应对不确定性。
模型是简化。 无论多么复杂,金融模型最终都是对复杂动态现实的简化。它们可以提供有价值的见解,但绝不能被视为对未来事件的万无一失的预测。
金融模型的局限性:
- 假设正态性,而现实世界的分布具有厚尾
- 难以考虑制度变化和结构性转变
- 无法捕捉非理性的人类行为和市场心理
- 低估罕见但极端事件的影响的倾向
对投资者和政策制定者的教训:
- 多样化仍然至关重要,但要意识到危机中相关性可能会改变
- 针对广泛的情景对投资组合和策略进行压力测试
- 认识到过去的表现并不能保证未来的结果
- 将定量模型与定性判断和常识相结合
记住:地图并不是领土。金融模型是辅助决策的工具,而不是可以确定预测未来的水晶球。
最后更新日期:
FAQ
What's Chancing It: The Laws of Chance and How They Can Work for You about?
- Exploring Probability: The book delves into the principles of probability and their impact on everyday life, decision-making, and scientific research.
- Real-Life Applications: It illustrates how understanding probability can aid in contexts like gambling, insurance, medical diagnoses, and investment strategies.
- Engaging Narrative: Robert Matthews combines personal anecdotes, historical examples, and scientific insights to create an engaging narrative for both specialists and non-specialists.
Why should I read Chancing It by Robert Matthews?
- Practical Decision-Making: The book equips readers with tools to make rational decisions in uncertain situations, relevant in today's complex world.
- Clear Explanations: Matthews explains complex mathematical concepts in an accessible manner, making it suitable for readers without a strong math background.
- Timely Insights: It provides a framework for evaluating claims and making informed decisions based on evidence and probability, crucial in an era of misinformation.
What are the key takeaways of Chancing It?
- Law of Averages: The book clarifies misconceptions about the Law of Averages, emphasizing relative frequencies over raw numbers.
- Bayesian Methods: Matthews highlights the importance of Bayesian probability for updating beliefs based on new evidence.
- Critical Thinking: It encourages questioning common assumptions about chance and risk, promoting a more analytical approach to decision-making.
How does Chancing It explain Bayesian methods?
- Bayesian Probability Defined: Matthews introduces Bayesian methods as a way to update beliefs based on new evidence, contrasting with traditional probability.
- Practical Applications: The book illustrates applications in fields like medicine and finance, showing how Bayesian methods improve decision-making.
- Likelihood Ratio: Emphasizes the importance of the Likelihood Ratio in Bayesian analysis for quantifying evidence strength.
What is the Law of Averages as explained in Chancing It?
- Misconceptions Addressed: Matthews clarifies that the Law of Averages refers to relative frequencies becoming predictable with more trials, not outcomes balancing out.
- Focus on Frequencies: The key takeaway is to concentrate on event frequencies relative to opportunities, essential for interpreting probabilistic scenarios.
- Real-World Examples: Uses experiments like coin tosses to demonstrate how the Law of Averages operates, showing randomness can lead to surprising short-term outcomes.
How does Chancing It critique traditional statistical methods?
- Limitations of Significance Testing: Matthews argues that significance testing often leads to false conclusions and lacks consideration of prior knowledge.
- Frequentist vs. Bayesian: Contrasts frequentist methods with Bayesian approaches, highlighting the latter's incorporation of prior beliefs for more reliable conclusions.
- Call for Change: Advocates for a shift towards Bayesian methods in research and decision-making for more accurate insights.
What are some real-world applications of Bayes's Theorem discussed in Chancing It?
- Medical Diagnoses: Illustrates how Bayes's Theorem improves diagnostic accuracy by updating disease probabilities based on test results.
- Search Operations: Uses the US Coast Guard's search for a missing fisherman to demonstrate decision-making guided by Bayesian updates.
- Cryptography: Discusses Alan Turing's use of Bayes's Theorem in code-breaking during WWII, showcasing its practical significance.
What is the "Problem of Priors" in Bayesian analysis?
- Definition: Refers to the challenge of establishing initial beliefs or probabilities before considering new evidence.
- Impact on Analysis: Without well-defined priors, Bayesian analysis results can be subjective and misleading.
- Addressing the Problem: Discusses strategies like using historical data or expert opinions to inform initial beliefs, enhancing analysis reliability.
What are some common misconceptions about probability discussed in Chancing It?
- Gambler’s Fallacy: The belief that past events influence future outcomes in independent trials is debunked; probabilities remain constant.
- Diagnostic Test Misinterpretation: Many overestimate condition likelihood based on accuracy rates without considering base rates; context is crucial.
- Randomness Equals Patternlessness: Clarifies that randomness can produce patterns over short sequences, misleading individuals into seeing trends.
What are the best quotes from Chancing It and what do they mean?
- Empowerment Through Understanding: "While we can never be free of chance, risk, and uncertainty, they all follow rules which can be turned to our advantage."
- Focus on Frequencies: "When trying to make sense of chance events, ignore the raw numbers. Focus instead on their relative frequency."
- Limitations of Certainty: "The dirty secret lurking in Bernoulli’s Golden Theorem is that when trying to gauge the effects of chance, God-like certainty is unattainable."
How does the author use historical examples to illustrate concepts in Chancing It?
- Alan Turing's Work: Turing's application of Bayes's Theorem in WWII code-breaking highlights its practical significance.
- US Coast Guard Search: Demonstrates the iterative process of updating beliefs with new evidence in real-world scenarios.
- Medical Case Studies: References medical studies to show Bayes's Theorem's impact on improving diagnostic accuracy.
How can I apply the concepts from Chancing It in my daily life?
- Evaluate Risks and Rewards: Use expected value and probability principles to assess decisions, from investments to health choices.
- Question Assumptions: Challenge common beliefs about chance and risk, seeking to understand underlying probabilities for better decision-making.
- Embrace Bayesian Thinking: Apply Bayesian methods to update beliefs with new information, allowing for continuous learning and adaptation.
评论
《机遇与概率法则:如何为你所用》获得了大多数积极的评价,因其对统计学和概率的易懂介绍而受到赞誉。读者们欣赏书中对复杂概念的清晰解释、历史背景以及实际应用。有些人觉得内容具有挑战性或过于专业,而另一些人则重视其对统计误区和贝叶斯方法的深刻见解。这本书与类似作品相比表现良好,推荐给那些希望理解概率及其在科学、金融等各个领域影响的人士。
Similar Books
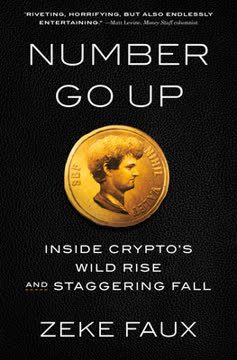
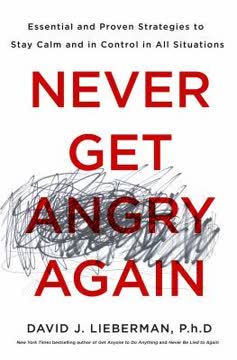
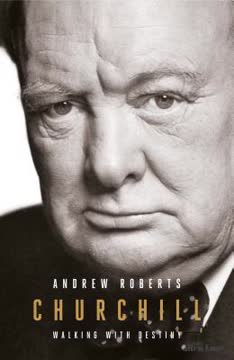
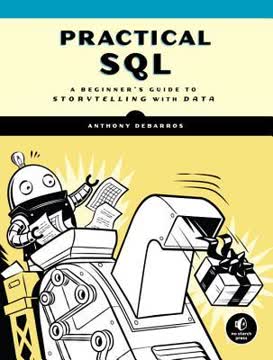
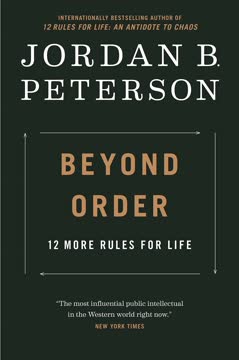
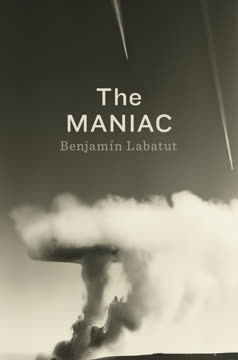
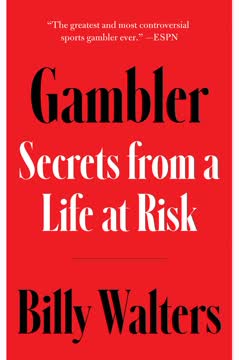