Key Takeaways
1. AI's Material Basis: Earth as an Extractive Industry
Computational media now participate in geological (and climatological) processes, from the transformation of the earth’s materials into infrastructures and devices to the powering of these new systems with oil and gas reserves.
AI's reliance on resources. Artificial intelligence is not an ethereal concept but a deeply material industry dependent on the extraction of Earth's resources. From lithium mines in Nevada to rare earth mineral deposits in Inner Mongolia, the creation of AI systems requires a vast supply chain of minerals, energy, and materials. This demand fuels environmentally destructive mining practices, often overlooked in discussions of technological progress.
Environmental impact. The tech sector's demand for resources contributes significantly to environmental degradation. The extraction of minerals contaminates waterways, destroys forests, and displaces communities. Furthermore, the energy-intensive nature of AI, particularly in training large models, contributes to a growing carbon footprint, rivaling that of the aviation industry.
Need for a shift in perspective. To understand the true cost of AI, we must move beyond the abstract promises of technological advancement and consider its material consequences. This requires acknowledging the environmental and human costs associated with resource extraction, energy consumption, and the global supply chains that support AI systems.
2. The Human Cost: Labor Exploitation in AI Systems
Coordinating the actions of humans with the repetitive motions of robots and line machinery has always involved a controlling of bodies in space and time.
AI's dependence on human labor. Despite the narrative of automation, AI systems rely heavily on human labor, often hidden and poorly compensated. This includes digital pieceworkers labeling data, Amazon warehouse employees fulfilling orders, and content moderators filtering harmful content. These workers are essential to making AI systems function, yet their contributions are often undervalued and their working conditions exploitative.
Time and control. The management of time is central to the exploitation of labor in AI systems. Workers are subjected to constant surveillance and algorithmic assessment, with their every action tracked and measured to maximize efficiency. This creates a stressful and dehumanizing work environment, where workers are treated as mere appendages to the machine.
Need for worker solidarity. To address the exploitation of labor in AI systems, workers must organize and demand better working conditions, fair wages, and greater control over their time and labor. This requires building solidarity across different sectors of the AI industry, from miners to engineers, and challenging the power structures that perpetuate exploitation.
3. Data as Infrastructure: The Erasure of Context and Consent
All publicly accessible digital material—including data that is personal or potentially damaging—is open to being harvested for training datasets that are used to produce AI models.
Data extraction. The AI industry relies on the mass harvesting of data, often without consent or regard for privacy. This includes personal information, images, and text scraped from the internet and used to train AI models. This practice treats data as a free resource, ignoring the ethical and social implications of collecting and using people's information without their knowledge or permission.
From image to infrastructure. The transformation of images into data strips them of their context and meaning. Mugshots, selfies, and personal photos are reduced to data points, used to train facial recognition systems and other AI models. This erasure of context can lead to biased and discriminatory outcomes, as AI systems learn to associate certain features with negative stereotypes.
Ethical concerns. The current practices of data collection and use in AI raise profound ethical concerns. We must move beyond the idea that data is a neutral resource and recognize the power dynamics inherent in its collection, labeling, and use. This requires developing ethical guidelines and regulations that protect people's privacy and prevent the misuse of their data.
4. Classification as Power: Encoding Bias in AI Systems
By looking at how classifications are made, we see how technical schemas enforce hierarchies and magnify inequity.
Classification as a political act. AI systems rely on classification to make sense of the world. However, the categories used to classify data are not neutral or objective but reflect the biases and assumptions of their creators. These biases can be encoded into AI systems, leading to discriminatory outcomes.
The problem of bias. AI systems have been shown to exhibit bias in a variety of domains, from facial recognition to criminal justice. These biases often reflect historical patterns of discrimination, perpetuating and amplifying existing inequalities. For example, facial recognition systems may be less accurate for people with darker skin, leading to misidentification and wrongful arrests.
Beyond bias debates. To address the problem of bias in AI, we must move beyond technical fixes and address the underlying social and political structures that shape the data and algorithms used to train AI systems. This requires challenging the power dynamics that perpetuate inequality and promoting more equitable and inclusive approaches to AI development.
5. Affect Recognition: The Troubled Science of Reading Emotions
The solution to the Clever Hans riddle, Pfungst wrote, was the unconscious direction from the horse’s questioners.
The claim of universal emotions. Affect recognition systems are based on the idea that emotions are universal and can be reliably detected from facial expressions. However, this claim is highly contested, with many researchers arguing that emotions are culturally variable and context-dependent.
The influence of Paul Ekman. The work of psychologist Paul Ekman has been influential in shaping the field of affect recognition. Ekman's research, which began in the 1960s, claimed to identify a set of basic emotions that are universally expressed and recognized. However, his methods and findings have been widely criticized for their lack of scientific rigor.
Ethical concerns. Despite the scientific doubts surrounding affect recognition, these tools are being rapidly deployed in a variety of high-stakes contexts, from hiring to policing. This raises serious ethical concerns, as people may be judged and discriminated against based on inaccurate and unreliable assessments of their emotional state.
6. AI as a Tool of State Power: Surveillance and Control
The military past and present of artificial intelligence have shaped the practices of surveillance, data extraction, and risk assessment we see today.
Military origins of AI. The development of AI has been heavily influenced by military funding and priorities. This has shaped the field's focus on surveillance, data extraction, and risk assessment, with little regard for the ethical and social implications.
The Snowden archive. The Snowden archive reveals the extent to which intelligence agencies have used AI to collect and analyze data on a massive scale. These tools, once reserved for national security purposes, are now being deployed domestically, blurring the lines between military and civilian surveillance.
The Third Offset strategy. The U.S. military's Third Offset strategy seeks to maintain its dominance in AI by partnering with the tech sector. This has led to a close relationship between the military and Silicon Valley, with tech companies providing AI tools and expertise to the Defense Department.
7. The Great Houses of AI: Centralizing Power and Widening Asymmetries
These politics are driven by the Great Houses of AI, which consist of the half-dozen or so companies that dominate large-scale planetary computation.
Concentration of power. The AI industry is dominated by a small number of powerful technology corporations. These companies control vast amounts of data, resources, and expertise, giving them a significant advantage in shaping the development and deployment of AI systems.
Widening inequalities. The concentration of power in the hands of a few tech giants exacerbates existing inequalities. AI systems are often designed to serve the interests of these companies, further widening the gap between the rich and the poor, the powerful and the marginalized.
Need for regulation. To address the concentration of power in the AI industry, we need stronger regulations that promote competition, protect privacy, and ensure that AI systems are used in a way that benefits society as a whole. This requires challenging the dominance of the tech giants and promoting more democratic and accountable forms of AI governance.
8. Challenging the Logics: Towards Interconnected Movements for Justice
As conditions on Earth change, calls for data protection, labor rights, climate justice, and racial equity should be heard together.
Interconnected movements. Addressing the foundational problems of AI requires connecting issues of power and justice. This includes data protection, labor rights, climate justice, and racial equity. By working together, these movements can challenge the structures of power that AI currently reinforces.
Politics of refusal. We must reject the idea that AI is inevitable and that we have no choice but to accept its consequences. This requires challenging the narratives of technological determinism and demanding more democratic and accountable forms of AI governance.
A different vision. By connecting issues of power and justice, we can create a different vision for AI, one that prioritizes human well-being, environmental sustainability, and social equity. This requires challenging the extractive logics of AI and building a more just and sustainable future for all.
Last updated:
FAQ
What's Atlas of AI about?
- Exploration of AI's Impact: Atlas of AI by Kate Crawford delves into the profound implications of artificial intelligence on society, politics, and the environment. It critiques the portrayal of AI as a neutral technology, highlighting its entanglement with power structures and extractive industries.
- Critique of AI Myths: The book challenges the notion that AI can replicate human intelligence, emphasizing that intelligence is shaped by cultural and historical contexts. Crawford uses examples like Clever Hans to illustrate biases in systems claiming objectivity.
- Interconnected Systems: Crawford presents AI as a "megamachine" reliant on a vast network of resources, including human labor and natural materials, urging readers to consider the broader implications of AI technologies on human rights and social justice.
Why should I read Atlas of AI?
- Critical Perspective: The book offers a critical lens on AI, challenging readers to think about the ethical and social ramifications of technology. It encourages a deeper understanding of how AI systems are designed and the biases they may perpetuate.
- Broader Context: Crawford situates AI within a larger framework of extraction and exploitation, linking it to historical and contemporary issues of power and inequality. This context is crucial for understanding the societal impacts of AI.
- Engaging Narratives: Combining rigorous research with compelling storytelling, the book makes complex topics accessible and engaging. Readers will find a blend of academic analysis and real-world examples that illustrate the stakes involved in AI development.
What are the key takeaways of Atlas of AI?
- AI as a Power Structure: The book posits that AI reflects existing power structures, serving the interests of those in control. AI systems are not neutral but are designed to optimize for specific outcomes that often reinforce inequality.
- Environmental and Labor Costs: Crawford highlights the significant environmental impact of AI, particularly in terms of resource extraction and energy consumption, and reveals how AI systems rely on cheap labor from marginalized communities.
- Call for Justice: Crawford advocates for a movement towards justice that addresses the interconnectedness of technology, capitalism, and social equity. She urges readers to consider how AI can be reoriented to serve the public good rather than perpetuate harm.
What are the best quotes from Atlas of AI and what do they mean?
- "Artificial intelligence is a registry of power.": This quote encapsulates Crawford's argument that AI systems reflect and reinforce existing power dynamics rather than being impartial technologies. It suggests that understanding AI requires examining who benefits from its deployment.
- "The story of Clever Hans is a cautionary reminder that you can’t always be sure of what a model has learned from the data it has been given.": This highlights the potential for bias in AI systems, emphasizing that the data used to train models can lead to misleading conclusions.
- “AI systems are expressions of power that emerge from wider economic and political forces.”: This quote challenges the reader to critically assess who benefits from AI technologies, emphasizing that AI is not neutral but reflects and amplifies existing power dynamics.
How does Kate Crawford define artificial intelligence in Atlas of AI?
- Not Artificial or Intelligent: Crawford argues that AI is neither truly artificial nor genuinely intelligent. Instead, it is a complex interplay of human labor, natural resources, and socio-political structures.
- Embodied and Material: She emphasizes that AI systems are built from physical materials and depend on extensive infrastructures, which include energy and labor. This challenges the common perception of AI as a purely digital phenomenon.
- Political and Social Practices: Crawford asserts that AI is shaped by political and social practices, meaning it cannot be understood in isolation from the contexts in which it operates.
What is the Clever Hans Effect mentioned in Atlas of AI?
- Observer-Expectancy Effect: The Clever Hans Effect refers to the phenomenon where a subject performs based on unintentional cues from the observer rather than genuine understanding. This highlights the influence of biases in interpreting intelligence.
- Implications for AI: Crawford uses this effect to illustrate how AI systems can also be misinterpreted based on the data they are trained on and the expectations of their creators.
- Broader Context of Intelligence: The effect raises questions about how intelligence is defined and measured, suggesting that our understanding of intelligence is often shaped by cultural and contextual factors.
How does Atlas of AI address the environmental impact of AI?
- Resource Extraction: Crawford discusses the extensive mining and resource extraction required to power AI technologies, particularly the demand for rare earth minerals, leading to significant ecological damage.
- Energy Consumption: The book highlights the enormous energy demands of AI systems, particularly in data centers, which contribute to carbon emissions and climate change.
- Interconnectedness with Climate Justice: Crawford connects the environmental impact of AI to broader issues of climate justice, arguing that the exploitation of resources for AI disproportionately affects marginalized communities.
What role does labor play in the construction of AI according to Atlas of AI?
- Exploitation of Workers: Crawford reveals how AI systems rely on cheap labor, often sourced from vulnerable populations, to function effectively. This exploitation is a critical aspect of the AI industry.
- Digital Piecework: The book discusses the rise of digital piecework, where workers are paid minimal amounts to perform tasks that support AI systems, such as labeling data.
- Surveillance and Control: Crawford highlights how AI technologies increase surveillance and control over workers, leading to a more regimented and exploitative work environment.
How does Atlas of AI address the issue of data extraction?
- Data Colonialism: Crawford discusses how data extraction often mirrors colonial practices, where marginalized communities are exploited for their data without consent or benefit.
- Consent and Privacy: The book emphasizes the lack of informed consent in data collection practices, particularly in how personal data is harvested for AI training.
- Impact on Communities: The extraction of data can have detrimental effects on communities, reinforcing existing inequalities and injustices.
What critiques does Atlas of AI offer regarding emotion recognition technologies?
- Scientific Foundations Questioned: Crawford highlights that many emotion recognition technologies are based on flawed scientific assumptions, particularly the idea that facial expressions universally correspond to specific emotions.
- Cultural Bias: The book points out that emotion recognition systems often fail to account for cultural differences in emotional expression, leading to biased outcomes.
- Potential for Harm: Crawford warns that deploying these technologies without understanding their limitations can lead to harmful consequences, such as misinterpretation of individuals' emotional states.
How does Atlas of AI connect AI to state power?
- Surveillance and Control: Crawford discusses how AI technologies are increasingly used by state actors for surveillance and control, often without adequate oversight.
- Military Applications: The book explores the relationship between AI development and military applications, particularly through programs like Project Maven.
- Corporate-State Collaboration: Crawford highlights the collaboration between tech companies and government agencies, suggesting that this partnership can lead to a lack of accountability and transparency.
What are the implications of Atlas of AI for the future of technology?
- Reevaluation of AI Development: Crawford's work calls for a reevaluation of how AI technologies are developed and deployed, emphasizing the need for ethical considerations and social responsibility.
- Advocacy for Justice Movements: The book encourages readers to engage in justice movements that address the intersections of technology, labor, and environmental issues.
- Critical Engagement with AI: Crawford urges readers to critically engage with AI technologies, questioning their implications and advocating for transparency and accountability.
Review Summary
Atlas of AI receives mixed reviews, with some praising its critical examination of AI's societal and environmental impacts, while others criticize its repetitive writing and lack of solutions. Readers appreciate the book's exploration of AI's material costs, labor exploitation, and ethical concerns. However, some find it overly pessimistic and lacking in technical depth. The book is commended for its comprehensive approach but criticized for its academic tone and occasional lack of focus. Despite its flaws, many consider it an important read for understanding AI's broader implications.
Similar Books
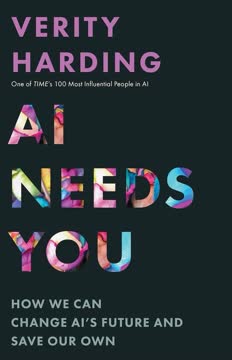
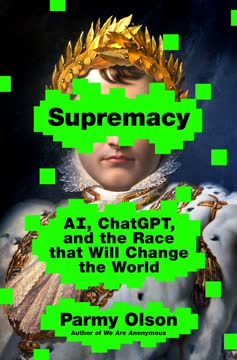
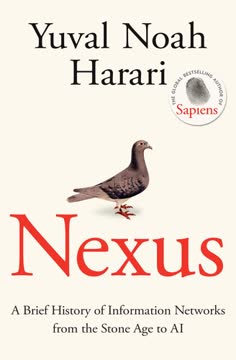
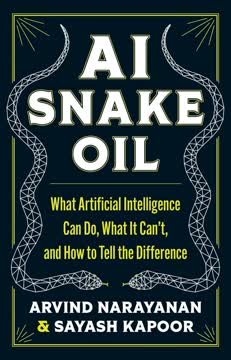
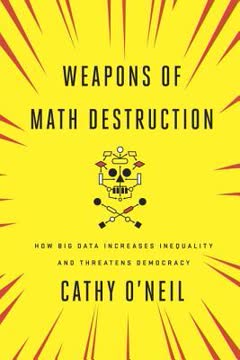
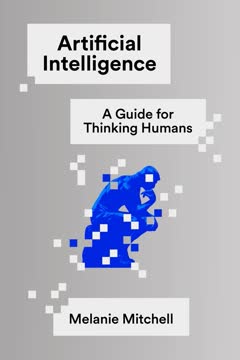
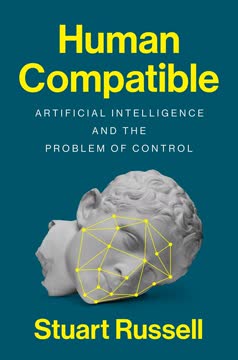
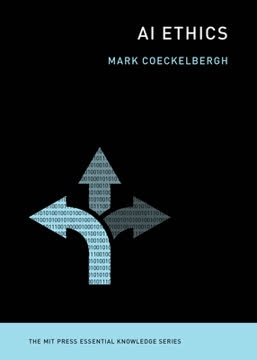
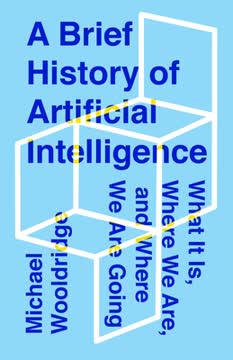
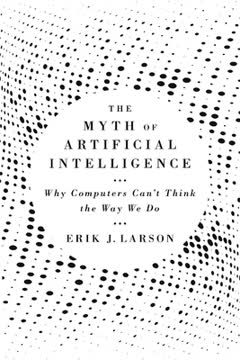
Download PDF
Download EPUB
.epub
digital book format is ideal for reading ebooks on phones, tablets, and e-readers.