Key Takeaways
1. Data Science: The Art of Extracting Actionable Insights from Data
The goal of data science is to improve decision making by basing decisions on insights extracted from large data sets.
Defining data science. Data science encompasses a set of principles, problem definitions, algorithms, and processes for extracting nonobvious and useful patterns from large data sets. It combines elements from various fields, including machine learning, data mining, and statistics, to analyze complex data and derive actionable insights.
Key components of data science:
- Data collection and preparation
- Exploratory data analysis
- Machine learning and statistical modeling
- Data visualization and communication of results
Value of data science. Organizations across industries are leveraging data science to gain competitive advantages, improve operational efficiency, and make better-informed decisions. From predicting customer behavior to optimizing supply chains, data science is transforming how businesses operate and compete in the modern world.
2. The CRISP-DM Process: A Framework for Data Science Projects
The CRISP-DM life cycle consists of six stages: business understanding, data understanding, data preparation, modeling, evaluation, and deployment.
Understanding CRISP-DM. The Cross Industry Standard Process for Data Mining (CRISP-DM) provides a structured approach to planning and executing data science projects. This iterative process ensures that projects remain focused on business objectives while maintaining flexibility to adapt to new insights.
The six stages of CRISP-DM:
- Business Understanding: Define project objectives and requirements
- Data Understanding: Collect and explore initial data
- Data Preparation: Clean, transform, and format data
- Modeling: Select and apply modeling techniques
- Evaluation: Assess model performance and alignment with business goals
- Deployment: Implement the model and integrate results into business processes
Importance of iteration. The CRISP-DM process emphasizes the need for continuous refinement and adaptation throughout a project's lifecycle. This iterative approach allows data scientists to incorporate new insights, address challenges, and ensure that the project remains aligned with evolving business needs.
3. Machine Learning: The Engine of Data Science
Machine learning involves using a variety of advanced statistical and computing techniques to process data to find patterns.
Fundamentals of machine learning. Machine learning algorithms enable computers to learn from data without being explicitly programmed. These algorithms can identify patterns, make predictions, and improve their performance with experience.
Key types of machine learning:
- Supervised Learning: Learns from labeled data to make predictions
- Unsupervised Learning: Discovers hidden patterns in unlabeled data
- Reinforcement Learning: Learns through interaction with an environment
Popular machine learning algorithms:
- Linear and Logistic Regression
- Decision Trees and Random Forests
- Neural Networks and Deep Learning
- Support Vector Machines
- K-Means Clustering
Machine learning forms the core of many data science applications, enabling organizations to automate complex tasks, make accurate predictions, and uncover insights that would be difficult or impossible for humans to discern manually.
4. Clustering, Anomaly Detection, and Association Rules: Key Data Science Tasks
Clustering involves sorting the instances in a data set into subgroups containing similar instances.
Essential data science tasks. These techniques form the foundation of many data science applications, enabling businesses to gain valuable insights from their data.
Clustering:
- Groups similar data points together
- Applications: Customer segmentation, image compression
- Common algorithm: K-means clustering
Anomaly detection:
- Identifies unusual patterns or outliers in data
- Applications: Fraud detection, system health monitoring
- Techniques: Statistical methods, machine learning algorithms
Association rule mining:
- Discovers relationships between variables in large datasets
- Applications: Market basket analysis, recommendation systems
- Popular algorithm: Apriori algorithm
These techniques provide powerful tools for uncovering hidden patterns, identifying potential issues, and making data-driven decisions across various industries and applications.
5. Prediction Models: Classification and Regression in Practice
Prediction is the task of estimating the value of a target attribute for a given instance based on the values of other attributes (or input attributes) for that instance.
Understanding prediction models. Prediction models are a crucial application of machine learning in data science, allowing organizations to make informed decisions based on historical data and current inputs.
Two main types of prediction models:
- Classification: Predicts categorical outcomes (e.g., spam or not spam)
- Regression: Predicts continuous numerical values (e.g., house prices)
Key steps in building prediction models:
- Data collection and preparation
- Feature selection and engineering
- Model selection and training
- Model evaluation and fine-tuning
- Deployment and monitoring
Prediction models have wide-ranging applications, from customer churn prediction in telecommunications to price forecasting in financial markets. The success of these models depends on the quality of data, appropriate feature selection, and careful model evaluation.
6. The Data Science Ecosystem: From Data Sources to Analytics
Databases are the natural technology to use for storing and retrieving structured transactional or operational data (i.e., the type of data generated by a company's day-to-day operations).
Components of the data science ecosystem. A robust data science infrastructure typically includes various components that work together to enable efficient data storage, processing, and analysis.
Key elements of the ecosystem:
- Data Sources: Transactional databases, IoT devices, social media, etc.
- Data Storage: Relational databases, data warehouses, data lakes
- Big Data Technologies: Hadoop, Spark, NoSQL databases
- Analytics Tools: SQL, R, Python, SAS, Tableau
- Machine Learning Platforms: TensorFlow, scikit-learn, H2O.ai
Trends in the ecosystem:
- Cloud-based solutions for scalability and flexibility
- Integration of real-time and batch processing
- Emphasis on data governance and security
- Adoption of automated machine learning (AutoML) tools
The evolving data science ecosystem enables organizations to handle increasing volumes and varieties of data, perform complex analyses, and derive actionable insights more efficiently than ever before.
7. Ethical Considerations and Privacy in the Age of Big Data
It is very difficult to predict how these changes will play out in the long term. A range of vested interests exist in this domain: consider the differing agendas of big Internet, advertising and insurances companies, intelligence agencies, policing authorities, governments, medical and social science research, and civil liberties groups.
Balancing innovation and privacy. As data science capabilities grow, so do concerns about privacy, fairness, and the ethical use of data. Organizations must navigate complex ethical considerations while harnessing the power of data science.
Key ethical considerations:
- Data privacy and protection
- Algorithmic bias and fairness
- Transparency and explainability of models
- Informed consent for data collection and use
- Responsible use of personal data
Regulatory landscape:
- General Data Protection Regulation (GDPR) in the EU
- California Consumer Privacy Act (CCPA) in the US
- Sector-specific regulations (e.g., HIPAA for healthcare)
Data scientists and organizations must prioritize ethical considerations in their work, implementing practices such as privacy by design, algorithmic auditing, and transparent data usage policies to build trust and ensure responsible innovation.
8. The Future of Data Science: Personalized Medicine and Smart Cities
Medical sensors worn or ingested by the patient or implanted are being developed to continuously monitor a patient's vital signs and behaviors and how his or her organs are functioning throughout the day.
Emerging applications of data science. As data science techniques advance and more data becomes available, new applications are emerging that promise to transform various aspects of our lives.
Personalized medicine:
- Genomic analysis for tailored treatments
- Continuous health monitoring through wearable devices
- AI-assisted diagnosis and treatment planning
Smart cities:
- Real-time traffic management and optimization
- Predictive maintenance of infrastructure
- Energy efficiency and sustainability improvements
- Enhanced public safety through predictive policing
These applications demonstrate the potential of data science to improve healthcare outcomes, enhance urban living, and address complex societal challenges. However, they also raise important questions about privacy, data ownership, and the balance between technological progress and individual rights.
9. Principles for Successful Data Science Projects
Successful data science projects need focus, good-quality data, the right people, the willingness to experiment with multiple models, integration into the business information technology (IT) architecture and processes, buy-in from senior management, and an organization's recognition that because the world changes, models go out of date and need to be rebuilt semiregularly.
Key success factors. Successful data science projects require a combination of technical expertise, business acumen, and organizational support.
Critical principles for success:
- Clear problem definition and project focus
- High-quality, relevant data
- Skilled and diverse project team
- Experimentation with multiple models and approaches
- Integration with existing IT systems and business processes
- Strong executive sponsorship and support
- Iterative approach with regular model updates
Common pitfalls to avoid:
- Lack of clear business objectives
- Poor data quality or insufficient data
- Overreliance on a single algorithm or approach
- Failure to integrate results into business processes
- Neglecting ethical considerations and privacy concerns
By adhering to these principles and avoiding common pitfalls, organizations can maximize the value of their data science initiatives and drive meaningful business impact.
Last updated:
FAQ
What's "Data Science" by John D. Kelleher about?
- Overview of Data Science: The book provides a comprehensive introduction to data science, covering its principles, problem definitions, algorithms, and processes for extracting patterns from large data sets.
- Relation to Other Fields: It explains how data science is related to data mining and machine learning but is broader in scope, encompassing data ethics and regulation.
- Practical Applications: The book discusses how data science is applied in various sectors, including business, government, and healthcare, to improve decision-making and efficiency.
- Historical Context: It offers a brief history of data science, tracing its development from data collection and analysis to its current state driven by big data and technological advancements.
Why should I read "Data Science" by John D. Kelleher?
- Comprehensive Introduction: The book is part of the MIT Press Essential Knowledge series, providing an accessible and concise overview of data science.
- Expert Insights: Written by leading thinkers, it delivers expert overviews of data science, making complex ideas accessible to nonspecialists.
- Practical Relevance: It highlights the impact of data science on modern societies, illustrating its applications in various fields like marketing, healthcare, and urban planning.
- Ethical Considerations: The book addresses the ethical implications of data science, including privacy concerns and the potential for discrimination.
What are the key takeaways of "Data Science" by John D. Kelleher?
- Data Science Definition: Data science involves principles and processes for extracting useful patterns from large data sets, improving decision-making.
- CRISP-DM Process: The book outlines the Cross Industry Standard Process for Data Mining, a widely used framework for data science projects.
- Machine Learning Role: Machine learning is central to data science, providing algorithms to create models from data for prediction and analysis.
- Ethical Challenges: It emphasizes the importance of addressing ethical issues, such as privacy and discrimination, in data science applications.
How does "Data Science" by John D. Kelleher define data science?
- Principles and Processes: Data science is defined as a set of principles, problem definitions, algorithms, and processes for extracting patterns from data.
- Broader Scope: It is broader than data mining and machine learning, encompassing data ethics, regulation, and the handling of unstructured data.
- Decision-Making Focus: The primary goal is to improve decision-making by basing decisions on insights extracted from large data sets.
- Interdisciplinary Nature: Data science integrates knowledge from various fields, including statistics, computer science, and domain expertise.
What is the CRISP-DM process mentioned in "Data Science" by John D. Kelleher?
- Standard Framework: CRISP-DM stands for Cross Industry Standard Process for Data Mining, a widely adopted framework for data science projects.
- Six Stages: It consists of six stages: business understanding, data understanding, data preparation, modeling, evaluation, and deployment.
- Iterative Process: The process is iterative, allowing data scientists to revisit previous stages based on new insights or challenges.
- Focus on Business Needs: It emphasizes understanding business needs and ensuring that data science solutions align with organizational goals.
How does "Data Science" by John D. Kelleher explain machine learning's role in data science?
- Core Component: Machine learning is a core component of data science, providing algorithms to extract patterns and create predictive models from data.
- Supervised vs. Unsupervised: The book explains the difference between supervised learning (with labeled data) and unsupervised learning (without labeled data).
- Model Evaluation: It discusses the importance of evaluating models to ensure they generalize well to new, unseen data.
- Algorithm Selection: The book highlights the need to experiment with different algorithms to find the best fit for a given data set and problem.
What ethical challenges does "Data Science" by John D. Kelleher address?
- Privacy Concerns: The book discusses the ethical implications of data science, particularly regarding individual privacy and data protection.
- Discrimination Risks: It highlights the potential for data science to perpetuate and reinforce societal prejudices and discrimination.
- Profiling Issues: The book examines how data science can be used for social profiling, leading to preferential treatment or marginalization.
- Regulatory Frameworks: It reviews existing legal frameworks and guidelines for protecting privacy and preventing discrimination in data science.
What is the significance of big data in "Data Science" by John D. Kelleher?
- Three Vs of Big Data: Big data is characterized by its volume, variety, and velocity, presenting both opportunities and challenges for data science.
- Technological Advancements: The book discusses how advancements in data storage, processing power, and analytics have driven the growth of big data.
- Impact on Society: Big data has transformed various sectors, enabling more informed decision-making and personalized services.
- Ethical Considerations: The book emphasizes the need to address ethical concerns related to big data, such as privacy and data ownership.
How does "Data Science" by John D. Kelleher describe the role of data visualization?
- Exploratory Tool: Data visualization is an important tool for exploring and understanding data, helping to identify patterns and trends.
- Communication Aid: It aids in communicating the results of data analysis to stakeholders, making complex data more accessible and understandable.
- Historical Context: The book traces the development of data visualization from early statistical graphics to modern techniques.
- Effective Design: It emphasizes the principles of effective data visualization, such as clarity, accuracy, and relevance.
What are the best quotes from "Data Science" by John D. Kelleher and what do they mean?
- "Data science is a partnership between a data scientist and a computer." This quote highlights the collaborative nature of data science, where human expertise and computational power work together to extract insights from data.
- "The goal of data science is to improve decision making by basing decisions on insights extracted from large data sets." This emphasizes the primary objective of data science: to enhance decision-making processes through data-driven insights.
- "Data are never an objective description of the world. They are instead always partial and biased." This quote underscores the importance of recognizing the limitations and biases inherent in data, which can affect analysis and conclusions.
- "Without skilled human oversight, a data science project will fail to meet its targets." This highlights the critical role of human expertise in guiding data science projects to success.
How does "Data Science" by John D. Kelleher address the future trends in data science?
- Smart Devices and IoT: The book discusses the proliferation of smart devices and the Internet of Things, which are driving the growth of big data.
- Personalized Medicine: It highlights the potential of data science to revolutionize healthcare through personalized medicine and precision treatments.
- Smart Cities: The book explores the development of smart cities, where data science is used to optimize urban planning and resource management.
- Ongoing Challenges: It acknowledges the ongoing challenges in data science, including ethical considerations and the need for continuous model updates.
What practical advice does "Data Science" by John D. Kelleher offer for successful data science projects?
- Clear Focus: The book emphasizes the importance of clearly defining the problem and goals of a data science project from the outset.
- Quality Data: It stresses the need for high-quality data and the importance of data preparation and cleaning in the project lifecycle.
- Team Collaboration: Successful projects often involve collaboration among a diverse team with complementary skills and expertise.
- Iterative Process: The book advocates for an iterative approach, allowing for continuous improvement and adaptation of models and processes.
Review Summary
Data Science receives generally positive reviews as an accessible introduction to the field. Readers appreciate its clear explanations of key concepts, algorithms, and ethical considerations. Many find it helpful for beginners or those seeking an overview, though some note it lacks technical depth. The book's coverage of real-world applications and business aspects is praised. While some criticize the basic nature of the content, others value its broad perspective on data science principles, tasks, and future trends.
Similar Books
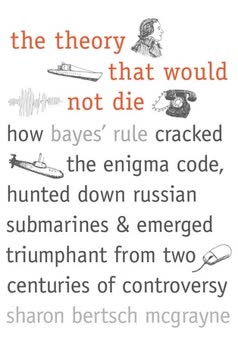
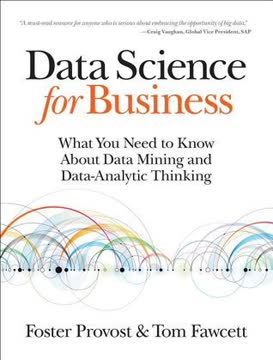
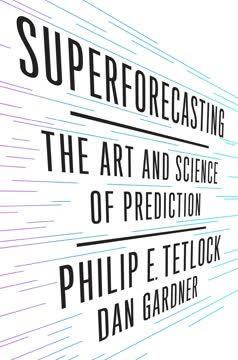
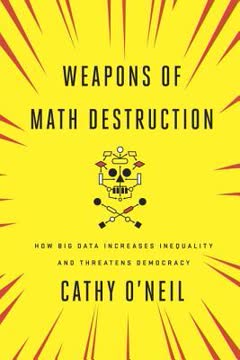
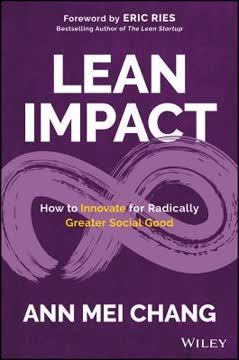
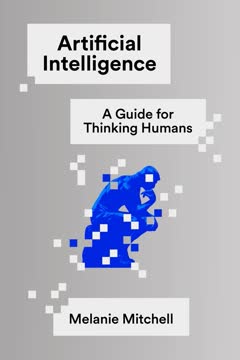
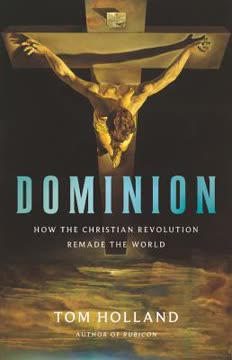
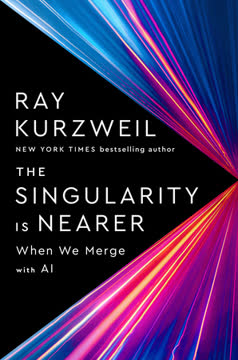
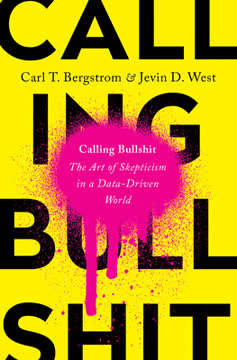
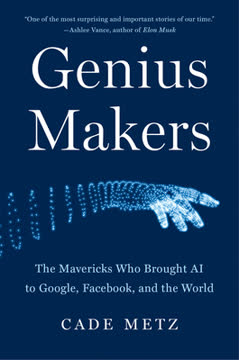
Download PDF
Download EPUB
.epub
digital book format is ideal for reading ebooks on phones, tablets, and e-readers.