Key Takeaways
1. Recognize your emotional responses to statistical claims
We are capable of persuading ourselves to believe strange things, and to doubt solid evidence, in service of our political partisanship, our desire to keep drinking coffee, our unwillingness to face up to the reality of our HIV diagnosis, or any other cause that invokes an emotional response.
Emotional reactions cloud judgment. Our feelings about statistical claims can lead us to accept or reject information prematurely. This emotional filter affects how we process information, often causing us to seek out data that confirms our existing beliefs and dismiss evidence that challenges them.
Awareness is key. To combat this tendency, we must:
- Pause and notice our initial emotional response to a claim
- Ask ourselves why we feel that way
- Consider whether our reaction is based on evidence or preconceptions
- Actively seek out information that challenges our beliefs
By developing this self-awareness, we can approach statistical claims more objectively and make better-informed decisions.
2. Combine bird's-eye data with worm's-eye experience
If we don't understand the statistics, we're likely to be badly mistaken about the way the world is. It is all too easy to convince ourselves that whatever we've seen with our own eyes is the whole truth; it isn't. Understanding causation is tough even with good statistics, but hopeless without them.
Balanced perspective is crucial. Statistics provide a broad, overarching view of trends and patterns, while personal experiences offer detailed, contextual insights. Neither perspective is complete on its own.
To gain a comprehensive understanding:
- Use statistics to identify large-scale trends and patterns
- Draw on personal experiences to provide context and nuance
- Look for ways to reconcile apparent contradictions between data and experience
- Seek out diverse perspectives to broaden your understanding
By integrating both bird's-eye and worm's-eye views, we can develop a more accurate and nuanced understanding of complex issues.
3. Understand what's being measured before drawing conclusions
Before we repeat any statistical claim, I first try to take note of how it makes me feel. It's not a foolproof method against tricking myself, but it's a habit that does little harm and is sometimes a great deal of help.
Definitions matter. Before analyzing or interpreting statistics, it's crucial to understand exactly what is being measured and how. Misunderstandings about definitions can lead to flawed conclusions and misguided decisions.
Key steps to avoid premature enumeration:
- Carefully examine the definitions used in the study or report
- Question assumptions about what terms like "unemployment" or "poverty" actually mean in the context of the data
- Look for potential discrepancies between common understanding and technical definitions
- Consider how different definitions might lead to different conclusions
By taking the time to understand the underlying measurements, we can avoid jumping to conclusions based on misleading or misunderstood data.
4. Seek context and comparisons to put claims in perspective
If you grow tired of undermining trust in your own statistical agency when it isn't producing politically convenient figures, you could always attack the statistical agency of someone else.
Context is essential. Individual statistics, no matter how striking, can be misleading without proper context. To truly understand the significance of a number or trend, we need to compare it to relevant benchmarks and consider its broader implications.
Strategies for gaining context:
- Look for historical trends: How has this number changed over time?
- Make geographical comparisons: How does this statistic compare across different regions or countries?
- Consider relative scales: Is this number large or small compared to relevant baselines?
- Seek out alternative measures: Are there other ways to quantify the same phenomenon?
By placing statistics in context, we can develop a more nuanced and accurate understanding of their true significance.
5. Investigate the origins and potential biases of data
There's a famous remark in a letter between Nightingale and Farr, written in the spring of 1861: 'You complain that your report would be dry. The dryer the better. Statistics should be the dryest of all reading.'
Data sources matter. The quality and reliability of statistics depend heavily on how they were collected and analyzed. Understanding the origins of data can reveal potential biases or limitations that affect their interpretation.
Key questions to ask about data sources:
- Who collected the data, and for what purpose?
- What methods were used to gather and analyze the information?
- Are there potential conflicts of interest or biases in the data collection process?
- Have the findings been replicated or verified by independent sources?
By critically examining the origins of data, we can better assess its reliability and identify potential limitations or biases that might affect our conclusions.
6. Consider who or what might be missing from the statistics
Without statistics, then, governments would fumble in ignorance. But there is an intriguing counterargument, which is that governments are so reliably incompetent that giving them more information is risky: it will only encourage them.
Missing data can distort reality. Statistics often fail to capture the full picture, either intentionally or unintentionally excluding certain groups or factors. Recognizing these gaps is crucial for accurate interpretation.
Areas to consider when evaluating statistical completeness:
- Demographic representation: Are all relevant groups included in the data?
- Geographic coverage: Does the data represent all relevant areas?
- Time frame: Is the data current and comprehensive across the relevant time period?
- Measurement limitations: Are there aspects of the issue that are difficult or impossible to quantify?
By actively seeking out what might be missing from the statistics, we can develop a more comprehensive and nuanced understanding of the issue at hand.
7. Demand transparency in algorithms and big data analytics
A situation where smokers shrugged and said to themselves 'I can't figure out all these confusing claims' was a situation that suited the tobacco industry well. Their challenge was not to convince smokers that cigarettes were safe, but to create doubt about the statistical evidence that showed they were dangerous.
Algorithmic transparency is crucial. As big data and algorithms increasingly shape our world, it's essential to understand how these systems work and what biases they might contain. Without transparency, we risk making decisions based on flawed or biased information.
Key aspects of algorithmic transparency:
- Access to underlying data and methodologies
- Clear explanations of how algorithms make decisions
- Regular audits to check for bias or unintended consequences
- Mechanisms for challenging or appealing algorithmic decisions
By demanding transparency in algorithmic systems, we can ensure that these powerful tools serve the public interest and are subject to appropriate scrutiny and accountability.
8. Value and protect the integrity of official statistics
Without statistics, governments would fumble in ignorance. But there is an intriguing counterargument, which is that governments are so reliably incompetent that giving them more information is risky: it will only encourage them.
Official statistics are foundational. Despite their limitations, government-produced statistics provide a crucial baseline for understanding social, economic, and demographic trends. Protecting the integrity and independence of these institutions is essential for informed decision-making.
Key reasons to value official statistics:
- Provide a consistent, long-term view of societal trends
- Offer a common reference point for public debate
- Enable evidence-based policymaking
- Allow for international comparisons and benchmarking
By recognizing the importance of official statistics and supporting their independence and integrity, we can ensure a more informed and data-driven society.
9. Look beyond beautiful visualizations to assess underlying data
Information is beautiful – but misinformation can be beautiful, too. And producing beautiful misinformation is becoming easier than ever.
Aesthetics can mask flaws. While data visualizations can be powerful tools for conveying information, they can also be used to mislead or obscure important details. It's crucial to look beyond the surface appeal to assess the underlying data and methodology.
Questions to ask when evaluating data visualizations:
- What is the source of the data?
- Are the scales and axes represented accurately?
- Does the visualization show the full context, or is it selective?
- Are there any distortions or visual tricks that might mislead?
By developing a critical eye for data visualizations, we can appreciate their communicative power while avoiding being misled by beautiful but flawed presentations.
10. Keep an open mind and be willing to update your beliefs
'When my information changes, I alter my conclusions. What do you do, sir?'
Flexibility is a strength. In a world of constantly evolving information, the ability to update our beliefs in light of new evidence is crucial. This requires both intellectual humility and a willingness to engage with ideas that challenge our existing views.
Strategies for maintaining an open mind:
- Actively seek out diverse perspectives and sources of information
- Be willing to admit when you're wrong or uncertain
- Treat new information as an opportunity to learn, not a threat to your beliefs
- Practice "steel-manning" opposing arguments by presenting them in their strongest form
By cultivating intellectual flexibility and openness, we can continuously refine our understanding of the world and make better-informed decisions.
Last updated:
FAQ
What's How to Make the World Add Up about?
- Understanding statistics and data: The book explores how to interpret and analyze statistics effectively, emphasizing the importance of understanding the context behind numbers.
- Ten rules for better thinking: Tim Harford presents ten rules to help readers navigate statistical information, encouraging curiosity and skepticism.
- Combating misinformation: It addresses the prevalence of misinformation and how statistics can be manipulated, emphasizing transparency and critical thinking.
Why should I read How to Make the World Add Up?
- Enhance critical thinking skills: The book provides practical tools to help you question and evaluate statistical claims, making you a more informed consumer of information.
- Relevance in today's world: Understanding statistics is crucial for making informed decisions in an age where misinformation spreads rapidly.
- Engaging and accessible writing: Tim Harford's storytelling approach makes complex concepts easier to understand and keeps readers interested.
What are the key takeaways of How to Make the World Add Up?
- Ten essential rules: The book outlines ten rules for thinking differently about numbers, such as "Search your feelings" and "Avoid premature enumeration."
- Importance of context: Understanding the context behind statistics is vital for accurate interpretation, encouraging readers to consider broader trends.
- Curiosity and skepticism: Maintaining a curious and skeptical mindset helps readers navigate the complexities of data and make informed decisions.
How does How to Make the World Add Up address the issue of misinformation?
- Misinformation prevalence: Harford discusses how misinformation is rampant, often fueled by misinterpretations of statistics.
- Statistical manipulation: The book provides examples of how statistics can be manipulated to support misleading narratives.
- Empowerment through knowledge: Understanding statistical reasoning helps readers navigate misinformation and make informed decisions.
What is the significance of the "premature enumeration" concept in How to Make the World Add Up?
- Definition of premature enumeration: It refers to the tendency to rush into analyzing statistics without fully understanding what is being measured.
- Importance of clarity: The concept highlights the need for clarity in definitions and measurements before diving into statistical analysis.
- Real-world implications: Harford illustrates this concept with examples, such as the misinterpretation of infant mortality rates.
How does Tim Harford suggest we deal with our emotional reactions to statistics in How to Make the World Add Up?
- Acknowledge your feelings: Harford advises recognizing emotional reactions when confronted with statistical claims.
- Pause for reflection: Reflecting on how a statistic makes you feel can improve judgment and encourage thoughtful analysis.
- Balance emotion with logic: While emotions are natural, balancing them with logical reasoning helps make informed decisions.
What role does context play in interpreting statistics according to How to Make the World Add Up?
- Understanding broader trends: Harford stresses the importance of considering the context of statistical claims to grasp the bigger picture.
- Comparative analysis: Context allows for meaningful comparisons between different datasets, providing a clearer understanding of the numbers.
- Avoiding misinterpretation: Without context, statistics can be easily misinterpreted or sensationalized.
What specific methods does Tim Harford suggest for interpreting statistics in How to Make the World Add Up?
- Ask critical questions: Question the source of the data, the methodology used, and the context in which the statistics are presented.
- Combine perspectives: Integrate personal experiences with statistical data for a more nuanced understanding of issues.
- Stay curious and open-minded: Maintain a curious mindset to navigate complex information more effectively.
How does How to Make the World Add Up address the issue of bias in statistics?
- Recognizing biases: Harford discusses how biases can influence data collection and interpretation, leading to misleading conclusions.
- Algorithmic bias: The book highlights examples of algorithms trained on biased datasets, warning against blindly trusting them.
- Importance of transparency: Harford advocates for transparency in data collection and analysis to mitigate biases.
What are some common pitfalls in interpreting statistics that How to Make the World Add Up warns against?
- Overgeneralization: Avoid making broad conclusions based on limited data or anecdotal evidence.
- Ignoring context: Failing to consider the context can lead to misinterpretation of statistics.
- Confirmation bias: Be vigilant against seeking information that only supports pre-existing beliefs.
How does How to Make the World Add Up address the relationship between statistics and decision-making?
- Informed decision-making: Understanding statistics is crucial for making informed decisions in both personal and professional contexts.
- Balancing emotion and logic: Recognize emotions while grounding decisions in solid data.
- Practical applications: Harford provides advice on applying statistical reasoning to everyday decisions.
What are the best quotes from How to Make the World Add Up and what do they mean?
- "Good statistics are not a trick, although they are a kind of magic.": Emphasizes that while statistics can seem deceptive, they are a powerful tool for understanding the world.
- "If we give in to a sense that we no longer have the power to figure out what’s true, then we’ve abandoned a vital tool.": Highlights the importance of maintaining confidence in discerning truth through statistical reasoning.
- "What does it say about statistics – and about us – that the most successful book on the subject is, from cover to cover, a warning about misinformation?": Reflects Harford's concern about the public's perception of statistics as misleading.
Review Summary
How to Make the World Add Up offers ten rules for interpreting statistics critically. Readers appreciate Harford's engaging writing style, real-world examples, and emphasis on curiosity. The book teaches how to spot misleading data, consider context, and question assumptions. While some found it repetitive or oversimplified, many praised its accessibility and importance in today's data-driven world. Harford encourages readers to be skeptical yet open-minded, aiming to improve statistical literacy without undermining the value of good data analysis.
Similar Books
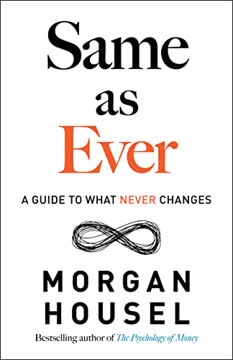
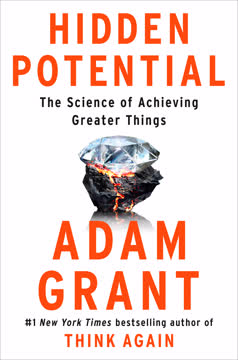
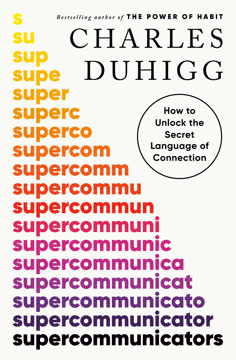
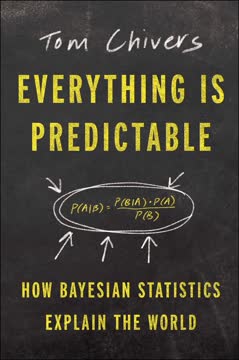
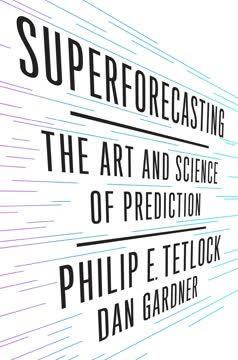
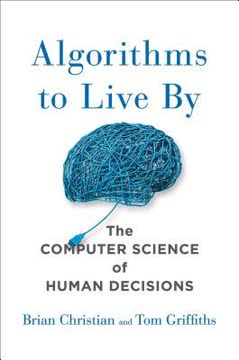
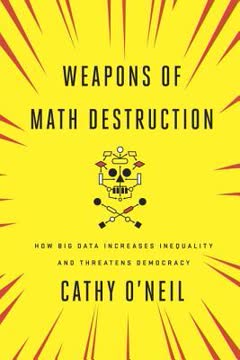
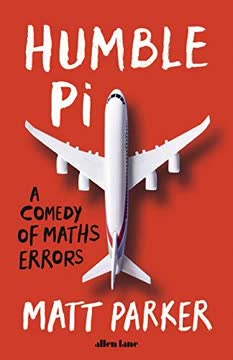
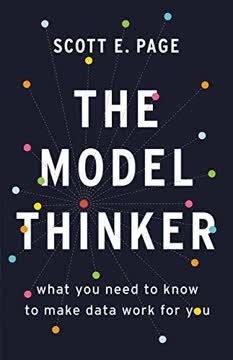
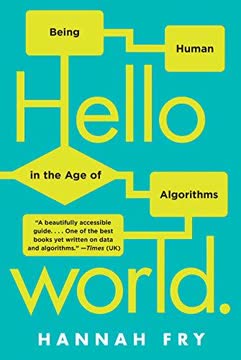
Download PDF
Download EPUB
.epub
digital book format is ideal for reading ebooks on phones, tablets, and e-readers.