Key Takeaways
1. Statistics: The Art of Learning from Data
The numbers have no way of speaking for themselves. We speak for them. We imbue them with meaning.
Data-driven insights. Statistics is the science of learning from data to understand the world and make better decisions. It involves collecting, analyzing, and interpreting data to draw meaningful conclusions. The field combines mathematical rigor with practical problem-solving, allowing us to extract valuable insights from complex information.
PPDAC cycle. A fundamental framework in statistics is the PPDAC cycle:
- Problem: Define the question or issue to be addressed
- Plan: Design the study or experiment
- Data: Collect and organize relevant information
- Analysis: Apply statistical techniques to uncover patterns
- Conclusion: Interpret results and communicate findings
This systematic approach ensures that statistical investigations are well-structured and focused on addressing real-world problems.
2. Turning the World into Data: Challenges and Opportunities
Even our most personal feelings can be codified and subjected to statistical analysis.
Data representation. Transforming real-world phenomena into data is a crucial step in statistical analysis. This process involves defining clear categories, measurements, and variables to represent complex realities. However, this transformation can be challenging and sometimes controversial.
Challenges in data collection:
- Defining precise categories (e.g., what constitutes a "tree"?)
- Ensuring consistent measurements over time
- Balancing detail with practicality
- Accounting for cultural and contextual factors
Despite these challenges, the ability to quantify and analyze various aspects of our world has led to significant advancements in fields such as economics, health, and social sciences. The key is to remain aware of the limitations and assumptions inherent in any data representation.
3. Probability: The Language of Uncertainty and Variability
Probability really is a difficult and unintuitive idea.
Quantifying uncertainty. Probability theory provides a mathematical framework for dealing with uncertainty and variability. It allows us to make predictions, assess risks, and draw inferences from limited data. Understanding probability is crucial for interpreting statistical results and making informed decisions.
Key probability concepts:
- Random variables and distributions
- Expected values and variance
- Conditional probability
- Law of Large Numbers
- Central Limit Theorem
While probability can be counterintuitive, tools like frequency trees and visual representations can help make complex concepts more accessible. Mastering probability is essential for advanced statistical techniques and for critically evaluating claims based on data.
4. Correlation, Causation, and the Power of Randomized Trials
Correlation does not imply causation.
Beyond association. While it's easy to find correlations in data, establishing causal relationships is much more challenging. Observational studies can reveal associations, but they are often confounded by other factors. Randomized controlled trials (RCTs) are the gold standard for determining causation.
Strengths of RCTs:
- Random allocation reduces bias
- Control groups account for placebo effects
- Blinding minimizes observer bias
- Pre-registration prevents p-hacking
However, RCTs are not always feasible or ethical. In such cases, careful study design, controlling for confounding variables, and using statistical techniques like propensity score matching can help strengthen causal inferences from observational data.
5. Statistical Models: Simplifying Complex Realities
All models are wrong, some are useful.
Model-based thinking. Statistical models are simplified representations of reality that help us understand patterns and make predictions. They range from simple linear regressions to complex machine learning algorithms. While all models have limitations, they can provide valuable insights when used appropriately.
Key aspects of statistical modeling:
- Choosing relevant variables
- Specifying relationships between variables
- Estimating parameters from data
- Assessing model fit and diagnostics
- Understanding limitations and assumptions
It's crucial to remember that models are tools for understanding, not perfect representations of reality. The goal is to find models that are useful for specific purposes while being aware of their limitations.
6. The Perils of P-values and the Reproducibility Crisis
Scientific conclusions and business or policy decisions should not be based only on whether a P-value passes a specific threshold.
Beyond statistical significance. P-values have long been used as a measure of statistical significance, with p < 0.05 often considered the threshold for "discovery." However, this approach has led to numerous problems in scientific research, including publication bias and the reproducibility crisis.
Issues with p-values:
- Misinterpretation of their meaning
- Arbitrary thresholds for significance
- Encouragement of p-hacking
- Neglect of effect sizes and practical significance
To address these issues, many statisticians advocate for more nuanced approaches, such as reporting effect sizes and confidence intervals, using Bayesian methods, and focusing on replication of results rather than single studies.
7. Bayesian Thinking: Learning from Experience
Bayes' legacy is the fundamental insight that the data does not speak for itself – our external knowledge, and even our judgement, has a central role.
Updating beliefs. Bayesian statistics provides a framework for updating our beliefs as we gather new evidence. It combines prior knowledge with observed data to form posterior probabilities. This approach is particularly useful in situations with limited data or when incorporating expert knowledge.
Key Bayesian concepts:
- Prior and posterior distributions
- Likelihood and Bayes' theorem
- Credible intervals
- Model comparison using Bayes factors
Bayesian methods offer a more intuitive approach to uncertainty and can be particularly useful in fields like medical diagnosis, where prior probabilities of diseases are well-known. However, they require careful consideration of prior distributions and can be computationally intensive.
8. Data Ethics and Responsible Statistics in the Modern World
Increasing concern about the potential misuse of personal data, particularly when harvested from social media accounts, has focused attention on the ethical aspects of data science and statistics.
Ethical considerations. As data becomes increasingly central to decision-making in various domains, statisticians and data scientists must grapple with ethical considerations. This includes issues of privacy, fairness, transparency, and the potential for misuse of statistical results.
Key ethical challenges:
- Protecting individual privacy in big data analyses
- Ensuring fairness in algorithmic decision-making
- Communicating uncertainty and limitations of analyses
- Addressing potential biases in data collection and analysis
- Balancing the benefits of data-driven insights with potential harms
Responsible statistical practice involves not only technical expertise but also a commitment to ethical principles and an awareness of the broader societal impacts of our work. As the field evolves, incorporating ethics into statistical education and professional practice becomes increasingly crucial.
Last updated:
FAQ
What's The Art of Statistics: Learning from Data about?
- Focus on Statistical Science: The book emphasizes the role of statistical science in understanding the world and making informed decisions based on data.
- Real-World Applications: It uses examples like Harold Shipman and child heart surgery to show how statistics can uncover truths and inform public health.
- Problem-Solving Framework: Introduces the PPDAC cycle (Problem, Plan, Data, Analysis, Conclusion) as a structured approach to statistical inquiry.
Why should I read The Art of Statistics?
- Enhance Data Literacy: It improves your ability to critically assess statistical claims and understand data implications in everyday life.
- Accessible to All: Designed for both students and general readers, it makes complex statistical concepts approachable without advanced math skills.
- Empower Decision-Making: Understanding statistical principles equips you to make informed decisions in personal and professional contexts.
What are the key takeaways of The Art of Statistics?
- Understanding Uncertainty: Emphasizes that all statistical estimates come with uncertainty, crucial for data interpretation.
- Importance of Context: Highlights how context influences data interpretation and perceptions of risk and outcomes.
- Causation vs. Correlation: Stresses the distinction between correlation and causation, a fundamental principle in statistics.
What are the best quotes from The Art of Statistics and what do they mean?
- "The numbers have no way of speaking for themselves. We speak for them.": Highlights the need for interpretation and context in deriving meaning from data.
- "All models are wrong, but some are useful.": Acknowledges the limitations of statistical models while recognizing their utility in predictions.
- "Correlation does not imply causation.": Reminds that correlation between variables does not mean one causes the other.
How does the PPDAC cycle work in The Art of Statistics?
- Structured Approach: PPDAC stands for Problem, Plan, Data, Analysis, and Conclusion, providing a systematic framework for statistical inquiries.
- Iterative Process: Each stage informs the next, allowing for continuous refinement based on findings.
- Real-World Examples: Illustrated with case studies, demonstrating its application in real-world analysis.
How does The Art of Statistics explain the difference between correlation and causation?
- Key Distinction: Emphasizes that correlation does not imply causation; other factors may influence the relationship.
- Examples Provided: Uses examples like ice cream sales and drowning rates to illustrate common misconceptions.
- Critical Thinking: Encourages critical thinking about variable relationships and seeking evidence of causation.
What is a confidence interval, as defined in The Art of Statistics?
- Definition: An estimated range within which an unknown parameter likely lies, based on observed data.
- Calculation: Typically calculated as the estimate ± a margin of error, reflecting the uncertainty of the estimate.
- Interpretation: Expresses the precision of an estimate, helping understand data reliability and variability.
What is the significance of the distinction between sample statistics and population parameters in The Art of Statistics?
- Understanding Estimates: Sample statistics estimate population parameters, crucial for accurate data interpretation.
- Uncertainty in Estimates: Discusses how sample statistics come with uncertainty, quantified using methods like bootstrapping.
- Implications for Inference: Highlights the importance of sample size and representativeness for making inferences about a population.
How does The Art of Statistics address the concept of causation?
- Causation vs. Correlation: Emphasizes careful analysis to establish causal relationships, not just correlations.
- Bradford Hill Criteria: Introduces criteria for assessing causation in observational studies, considering factors like strength and consistency.
- Importance of Randomized Trials: Advocates for randomized controlled trials as the gold standard for establishing causation.
What role does probability play in The Art of Statistics?
- Foundation for Inference: Provides the mathematical foundation for statistical inference, quantifying uncertainty and making predictions.
- Different Interpretations: Discusses classical, frequentist, and subjective approaches, highlighting their relevance in different contexts.
- Real-World Applications: Applied to scenarios like estimating unemployment rates, reinforcing its practical importance.
How does The Art of Statistics explain the concept of bootstrapping?
- Resampling Technique: Described as a method of repeatedly sampling from a dataset with replacement to estimate variability.
- Confidence Intervals: Used to create confidence intervals, enhancing understanding of uncertainty in sample statistics.
- No Strong Assumptions: Does not require strong assumptions about population distribution, making it a flexible tool.
What are some common pitfalls in statistical practice highlighted in The Art of Statistics?
- Questionable Research Practices: Discusses issues like selective reporting and P-hacking, leading to misleading conclusions.
- Publication Bias: Highlights the problem of publication bias, skewing scientific literature and misleading future research.
- Misinterpretation of Results: Warns against confusing correlation with causation or overgeneralizing from small samples.
Review Summary
The Art of Statistics is praised for its engaging approach to explaining statistical concepts without heavy math. Readers appreciate the real-world examples and clear explanations of complex topics. Many find it useful for understanding how to interpret statistics in media and research. Some criticize it for being too basic in parts and too complex in others. Overall, it's recommended for those wanting to improve their statistical literacy, though opinions vary on its accessibility for complete beginners.
Pelican Books Series Series
Similar Books
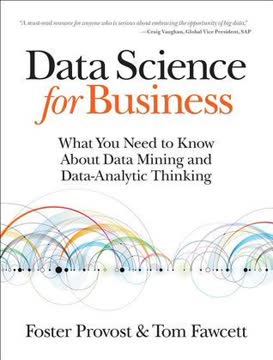
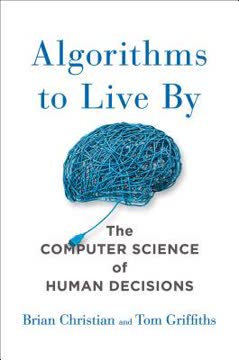
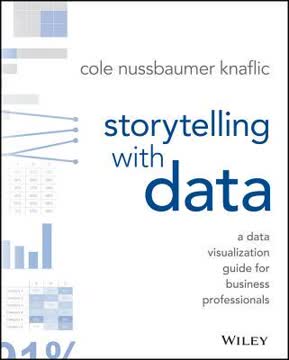
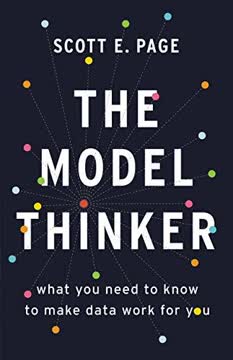

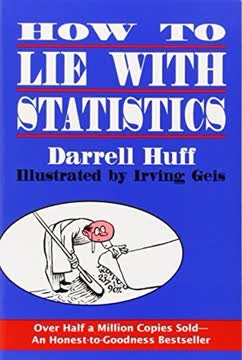
Download PDF
Download EPUB
.epub
digital book format is ideal for reading ebooks on phones, tablets, and e-readers.